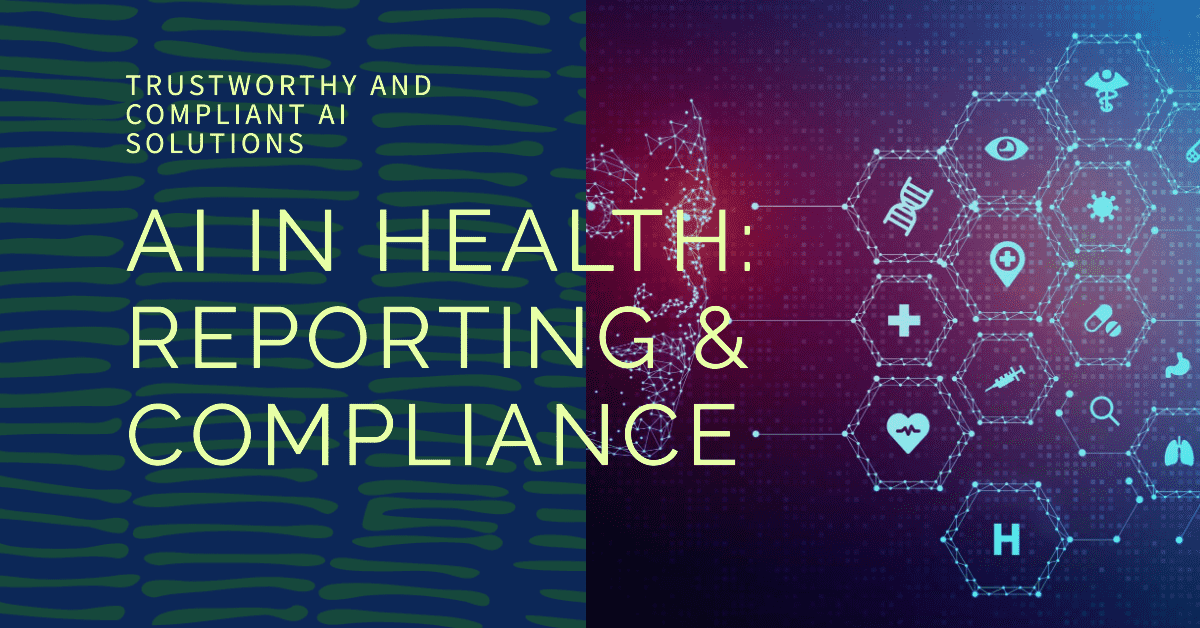
The healthcare industry is undergoing a digital transformation. Artificial intelligence (AI) is being adopted across the care continuum to improve clinical, financial, and operational outcomes. However, with innovation comes new challenges around reporting, governance, and compliance.
In this post, we'll explore how AI is impacting healthcare reporting and compliance requirements. We'll also provide recommendations for creating a robust governance framework to support ethical and responsible AI adoption in healthcare.
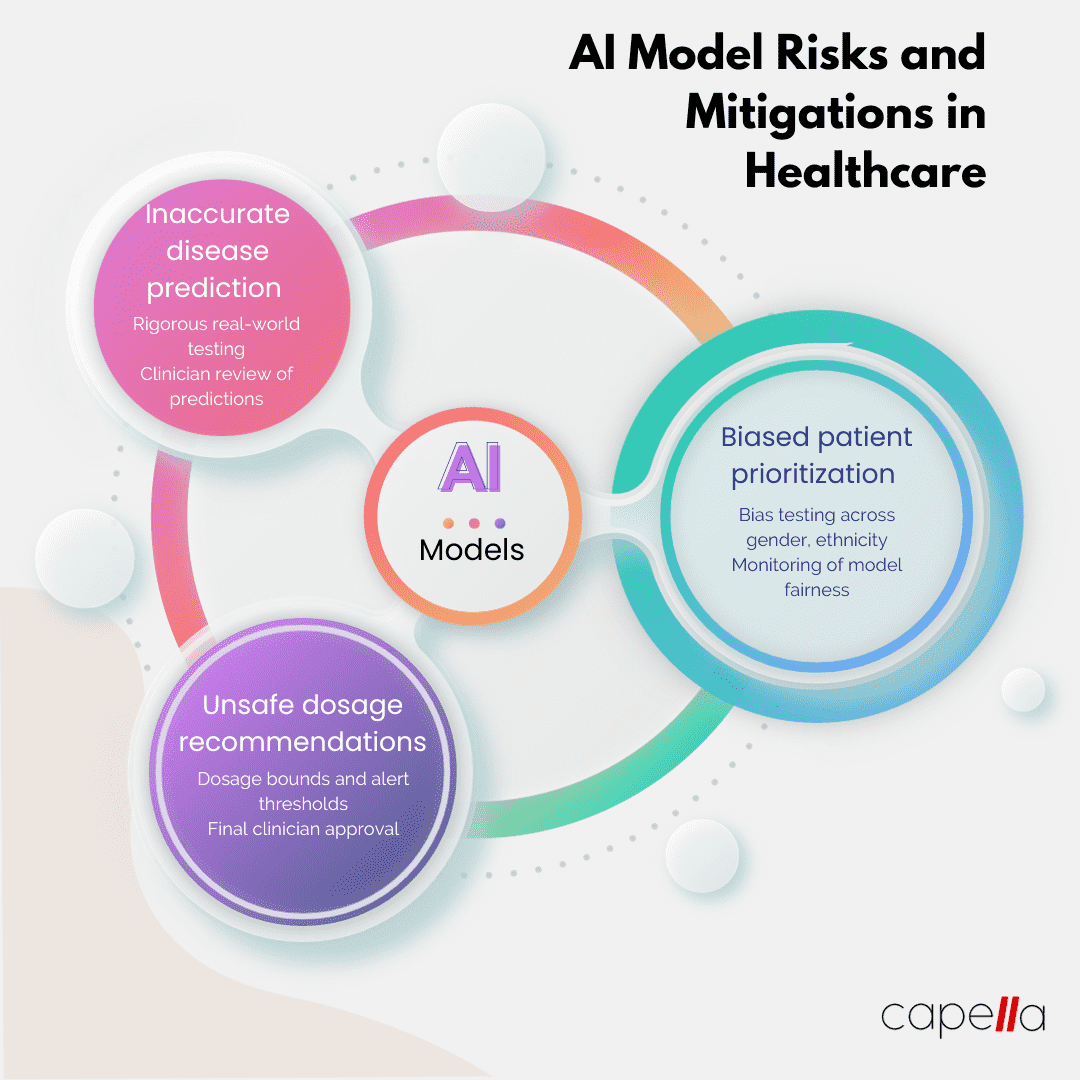
The Promise and Perils of AI in Healthcare
AI has huge potential to improve healthcare delivery. Algorithms can analyze vast amounts of data to derive insights faster than humans. AI can automate mundane tasks to allow doctors to focus on patients. And machine learning models can provide personalized medicine tailored to an individual's genetics, lifestyle, and medical history.
Here are some examples of how AI is enhancing healthcare today:
- Diagnostics - Algorithms can analyze medical images to detect abnormalities and diseases earlier and more accurately than clinicians. AI can also process test results and patient data to diagnose conditions.
- Treatment plans - AI can develop customized treatment plans by assessing the patient's records against medical guidelines and knowledge from millions of other cases.
- Risk identification - Machine learning can analyze a patient's medical and family history to predict risks of developing certain conditions, allowing preventive care.
- Administrative tasks - Virtual assistants powered by AI are handling appointment bookings, billing questions, and other clerical tasks to improve efficiency.
While promising, AI also raises challenges around transparency, bias, and control. Some considerations include:
- AI is only as good as the data it's trained on. Models can perpetuate biases in the data.
- It's not always clear how AI makes decisions. Lack of transparency can be problematic.
- AI behavioral patterns can shift over time as models continue learning. This creates risks of unintended consequences.
- Poorly designed systems could disempower doctors and put patients at risk.
Let's look at how these issues impact reporting and compliance in healthcare.
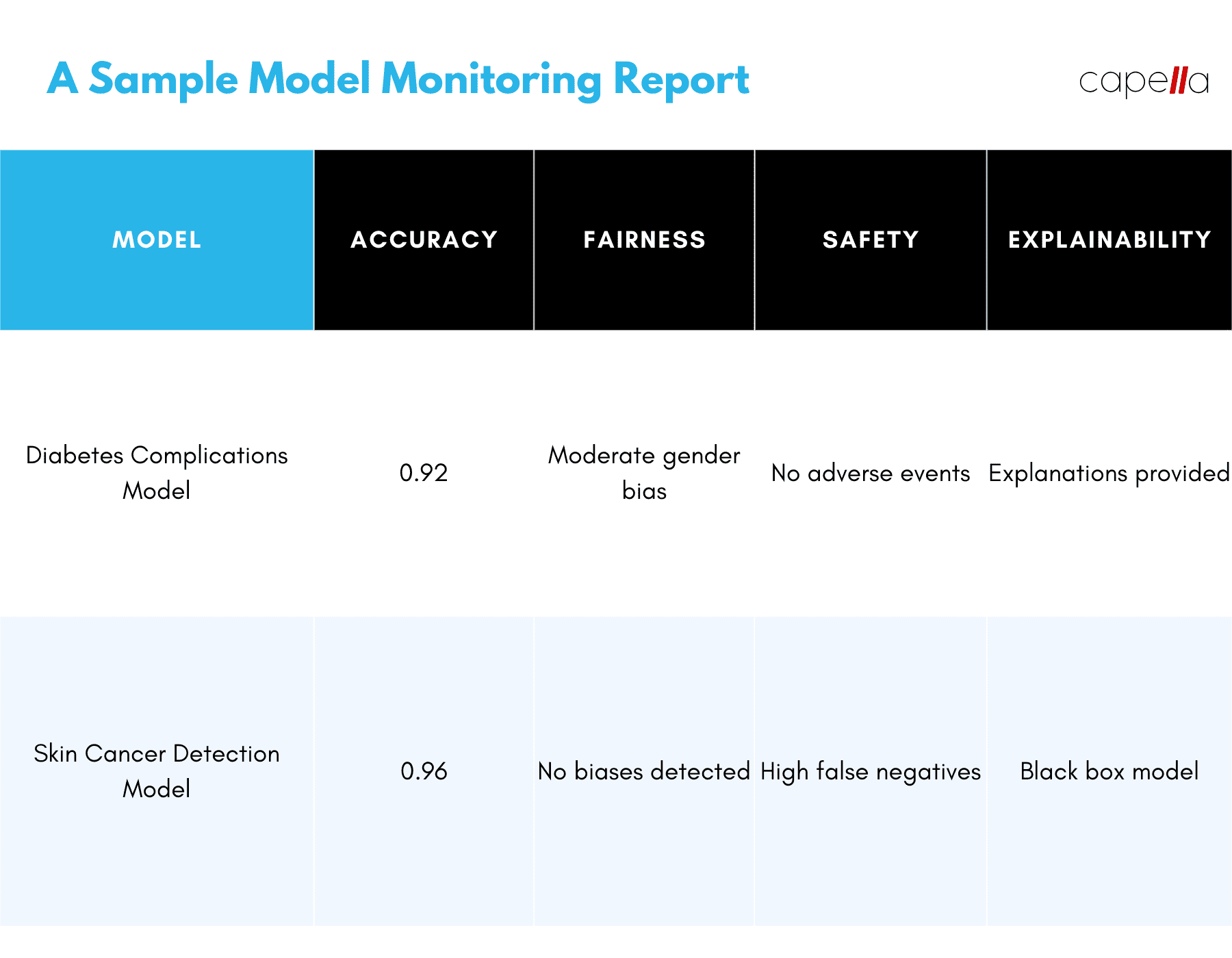
AI Reporting in Healthcare
Robust reporting is crucial for monitoring AI risks and ensuring it's used ethically. Here are some examples of important AI reports in healthcare:
1. Model monitoring reports
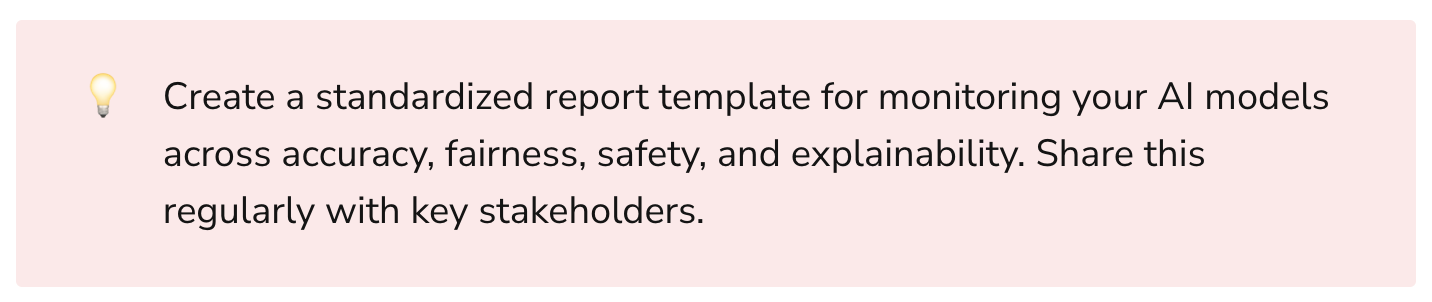
These provide visibility into how AI models are performing over time. Key metrics to report on include:
- Accuracy - Are model predictions correct? Is performance consistent across patient subgroups?
- Fairness - Is the model free from unfair bias against protected groups?
- Safety - Does the model avoid harmful or dangerous actions?
- Explainability - Can the model's reasoning be understood by humans?
Continuously monitoring these metrics allows identifying any deterioration or unfair outputs before they impact patients.
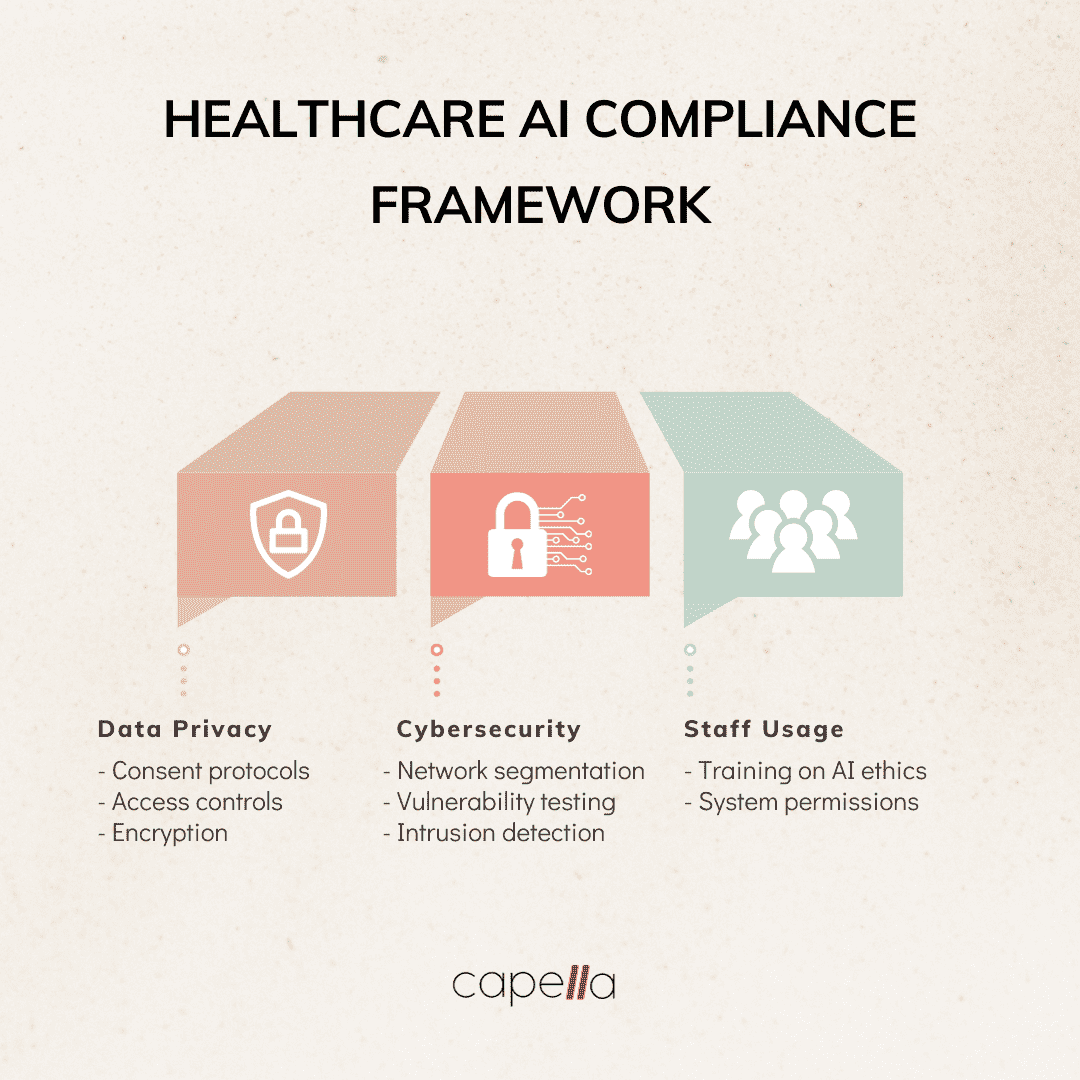
2. Compliance reports

These ensure AI adheres to healthcare regulations, laws, and organizational policies. Some key areas to report on include:
- Data privacy - Is personal health information (PHI) being collected, stored, and used appropriately?
- Consent - Are clear consent processes in place before collecting patient data?
- Staff usage - Is AI being used appropriately by doctors, nurses, and other staff?
Non-compliance in any of these areas poses legal, ethical and organizational risks.
3. Risk assessment reports
These evaluate emerging risks from AI to patient health and safety. Sample risks dimensions include:
- Patient misdiagnosis - Could the AI fail to detect a serious illness?
- Erroneous prescription - Could the model recommend an incorrect drug or dosage?
- Delayed attention - Might the AI lead to a patient not receiving timely care?
Proactively identifying such risks allows mitigation before harm. Risk assessments should cover both machine and human components of AI systems.
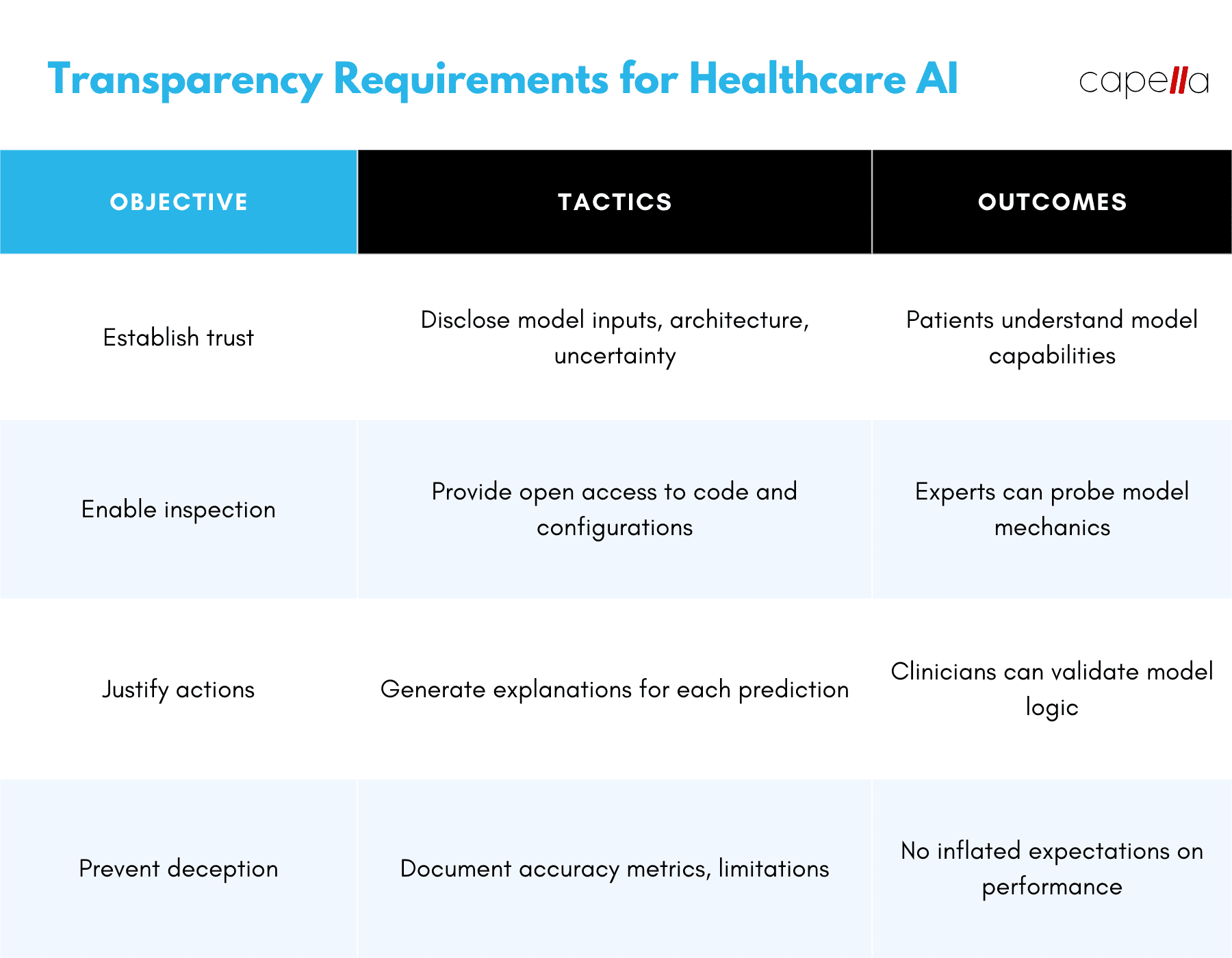
Health Compliance Considerations for AI
While healthcare has always involved extensive compliance needs, AI introduces new requirements. Key areas to address include:
Informed consent
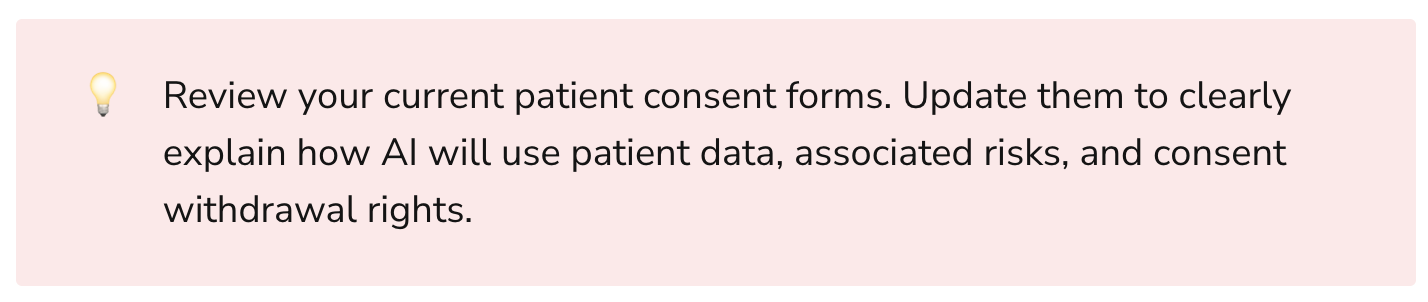
Patients must expressly permit the collection and AI analysis of their data. This encompasses:
- Disclosing how the data will be used
- Articulating benefits and risks
- Explaining rights to revoke consent
- Confirming patient understands consent
Obtaining informed consent is mandatory both legally and ethically before using patient data for AI.
Control measures

Checks must be put in place to prevent unethical or risky AI use. Examples include:
- Human-in-the-loop reviews of AI output before clinical implementation
- Sparing particularly sensitive use cases (e.g. end-of-life decisions) from automation
- Monitoring staff usage of AI and setting appropriate permissions
Such control measures create accountability within the AI system.
Transparency mandates
Many jurisdictions now mandate transparency in healthcare AI. This requires:
- Explaining what patient data the model uses
- Justifying suggested actions so users can trust the AI
- Enabling inspection of the model's architecture and code
- Disclosing accuracy metrics to establish trust
Fulfilling transparency requirements builds patient and clinician confidence in adopting AI.
Cybersecurity and privacy
AI systems must prevent unauthorized access to sensitive patient data. Robust safeguards are needed for:
- Access controls to PHI
- Data encryption, both in transit and at rest
- Network segmentation and intrusion detection
- Ongoing vulnerability testing and patching
Any breaches pose major compliance, reputational and patient trust risks and must be avoided.
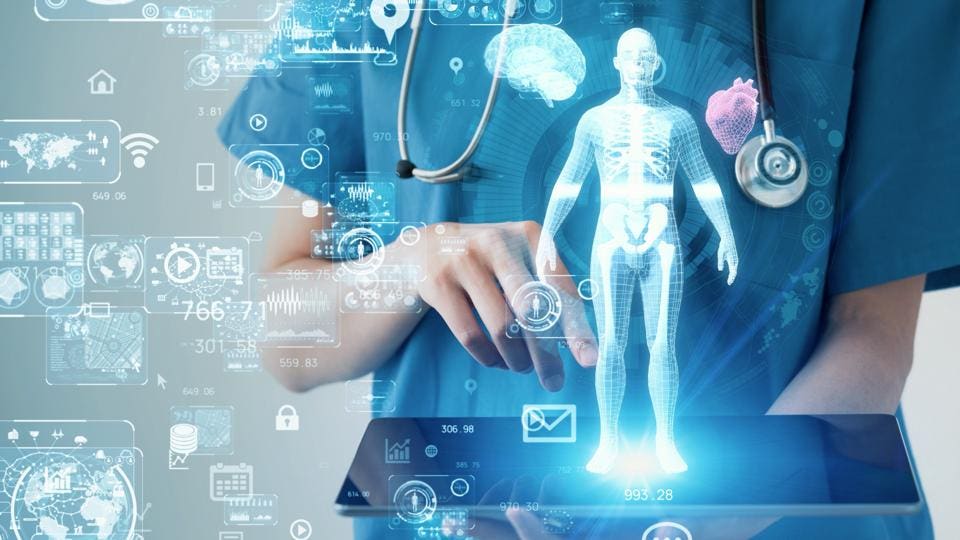
Creating a Governance Model for Healthcare AI
Responsibly scaling AI in healthcare requires a deliberate governance strategy. Key pillars of an effective model include:
Policies
Documented policies codify responsible AI practices across the organization. Examples include:
- Consent policy - standardizes informed consent across applications
- Risk management - sets protocols to identify and mitigate AI risks
- Bias prevention - establishes controls to avoid unfair model outputs
Published policies provide a north star for teams implementing AI.
Change management
Modifying AI systems can have unintended effects. A structured change process should:
- Require full documentation of proposed changes
- Set review and approval procedures by risk level
- Log all changes and alert relevant stakeholders
- Test changes in controlled environments before deployment
This governance helps safely evolve models while minimizing compliance issues.
Education

Clinicians and patients may misunderstand how AI works. Education programs should:
- Train staff on ethical AI practices and operating procedures
- Coach patients on basics of how models use their data
- Set appropriate expectations on AI strengths and limitations
Learning helps ensure AI is used properly and improves adoption.
Oversight
Independent oversight provides unbiased perspective on the state of AI governance. Useful methods include:
- External audits to validate controls and compliance
- Advisory boards with subject matter experts
- Internal compliance reviews
Multi-pronged oversight identifies blind spots, enhances accountability, and provides reassurance.
The Future of Healthcare AI
AI has vast potential to improve healthcare outcomes if governance keeps pace with innovation. As algorithms become more central in care delivery, maintaining trust via responsible AI practices will be critical. With deliberate effort, healthcare organizations can harness AI to provide safer, more equitable and personalized care for all.
In this post, we explored healthcare reporting requirements, compliance considerations, and governance models for AI. Responsible AI practices will be imperative as algorithms play a growing role in care. While challenges remain, healthcare leaders that prioritize fair, transparent and controlled AI development can unlock huge value for clinicians, patients, and communities.
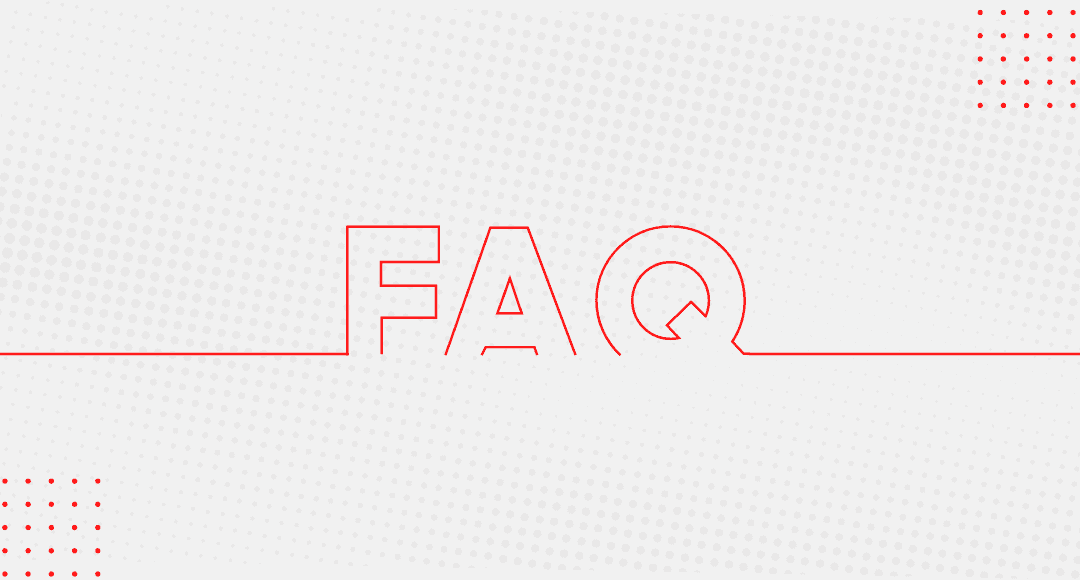
1. What are some of the main benefits of AI in healthcare?
AI has wide-ranging potential to improve healthcare by automating mundane tasks, speeding analysis of complex data, and enhancing clinical decision making. Key benefits include faster diagnosis of illness based on scans and tests, personalized treatment plans tailored to the patient, automated monitoring of patient health between visits, and workload reduction for clinicians through AI assistants. Overall, AI promises more accurate, consistent and efficient healthcare delivery.
2. What are some key risks and challenges with deploying AI in healthcare?
AI introduces several risks that must be managed:
- Potential for perpetuating biases if the algorithms are trained on biased datasets
- Lack of transparency around how AI systems make decisions, which reduces trust
- Cybersecurity vulnerabilities since AI relies on vast data collection and sharing
- Difficulty predicting AI behavior as the systems continue learning after deployment
- Loss of human accountability as more decisions get automated
3. Why is rigorous reporting on AI models important in healthcare?
Careful reporting helps ensure AI is improving healthcare equitably and safely without introducing unintended risks. Key reporting dimensions include monitoring for accuracy, fairness, safety, explainability, and compliance. This reporting creates accountability and opportunities to tune the AI if any issues emerge.
4. What are some best practices for obtaining patient consent for AI in healthcare?
Rigorous consent processes are crucial. Patients must expressly permit the collection and AI analysis of their data. This requires:
- Disclosing intended uses of the data
- Articulating potential benefits and risks from AI
- Detailing continuous learning aspects where patterns gleaned from the data will be applied to other patients
- Explaining rights to revoke consent and have data deleted
- Allowing sufficient time for the patient to review consent details without pressure
- Following up to ensure consent is fully understood before signature
5. How can healthcare organizations manage risks from AI systems?
Proactive risk management is imperative. Useful strategies include:
- Extensive testing of AI systems for safety and unintended biases before deployment
- Maintaining humans in the loop for reviewing and approving all high-risk AI recommendations
- Monitoring AI system behavior continuously after deployment to catch emerging risks
- Establishing robust cybersecurity protections for patient data
- Creating mechanisms for explaining AI decisions to engender clinical trust
6. What transparency requirements apply to AI in healthcare?
Various laws now mandate transparency for healthcare AI to build patient and clinician confidence. Key requirements include:
- Disclosing which patient data elements are used by the models
- Providing details on model architecture and algorithms
- Explaining the reasoning behind each AI-generated recommendation
- Publishing accuracy statistics to show real-world performance
7. What safeguards help ensure AI compliance in healthcare?
Key compliance safeguards include:
- Mandatory training for clinicians on responsible and ethical AI use
- Checking algorithmic outputs for fairness across protected groups before implementation
- Rigorous consent protocols for collecting and analyzing patient data
- Ongoing audits to verify continued data privacy and cybersecurity controls
- Establishing access controls to limit unauthorized access or changes to AI systems
Multilayered governance helps maintain compliance as AI usage evolves.
8. How can healthcare organizations foster responsible AI practices?
Responsible AI requires concerted effort across policies, processes, and education:
- Set policies for informed consent, bias testing, risk reviews, and more
- Institute change management processes to carefully evolve AI systems
- Provide extensive training to clinicians on AI capabilities and ethical use
- Perform rigorous compliance and safety audits
- Create independent advisory boards to oversee AI risks
Combining these efforts promotes patient wellbeing.
**9. What is the role of clinicians in an era of advanced AI?
Even with powerful AI, clinicians remain integral:
- They help patients interpret and feel comfortable with AI recommendations
- They provide oversight of case management and treatment plans along with AI
- They make judgment calls on the applicability of AI suggestions for a patient
- They supply vital social and emotional support unavailable from machines
- They bring accountability and ethics to decisions around patient care
So while AI takes over select tasks, clinicians retain an essential and evolving role.
10. What does responsible and ethical AI adoption in healthcare require?
Responsible AI adoption necessitates:
- A relentless focus on patient benefit, safety, privacy, and fair treatment
- Continuous monitoring of AI risks, biases, and model performance
- Extensive training to develop AI literacy and oversight skills in clinicians
- Comprehensive risk assessment and mitigation for AI systems
- Full transparency on AI capabilities, limitations, and reasoning to patients
- Empowered clinicians who critically evaluate AI suggestions based on their experience and expertise
With deliberate efforts across these dimensions, healthcare providers can unlock AI’s benefits while minimizing its risks.
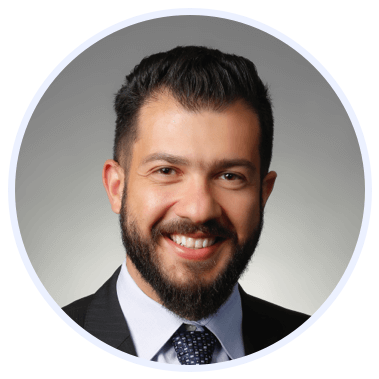
Rasheed Rabata
Is a solution and ROI-driven CTO, consultant, and system integrator with experience in deploying data integrations, Data Hubs, Master Data Management, Data Quality, and Data Warehousing solutions. He has a passion for solving complex data problems. His career experience showcases his drive to deliver software and timely solutions for business needs.