
At Capella, I have seen firsthand how artificial intelligence (AI) is transforming this field. Supply chains have become increasingly complex, with globalized networks, many parties involved, and dynamic changes in demand. AI has emerged as a solution to make sense of all this complexity and enable data-driven decision-making.
In this essay, I will discuss the key ways AI is improving supply chain optimization across three main areas: forecasting, logistics, and inventory management. I will share tactical examples of how companies are applying AI-based solutions to drive quantifiable results. By the end, you will understand the tangible benefits AI can offer and some practical ways to leverage this technology in your operations.
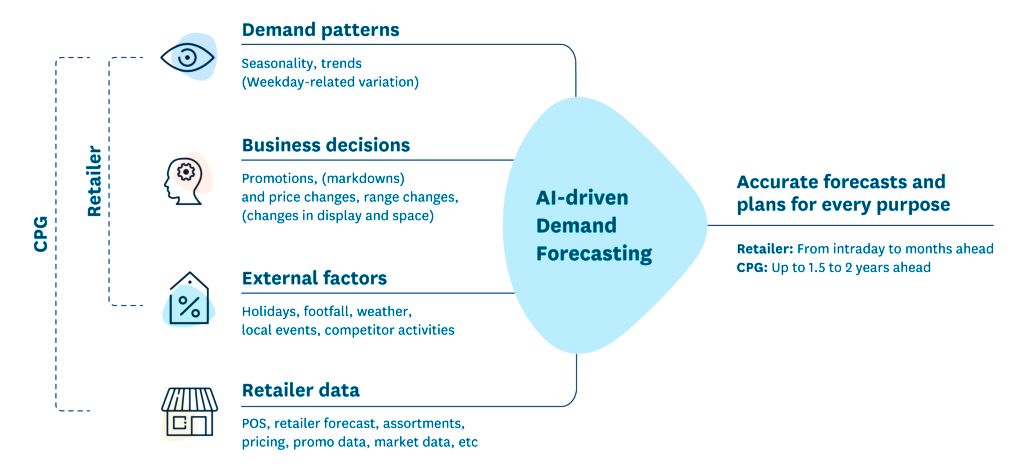
More Accurate Demand Forecasting
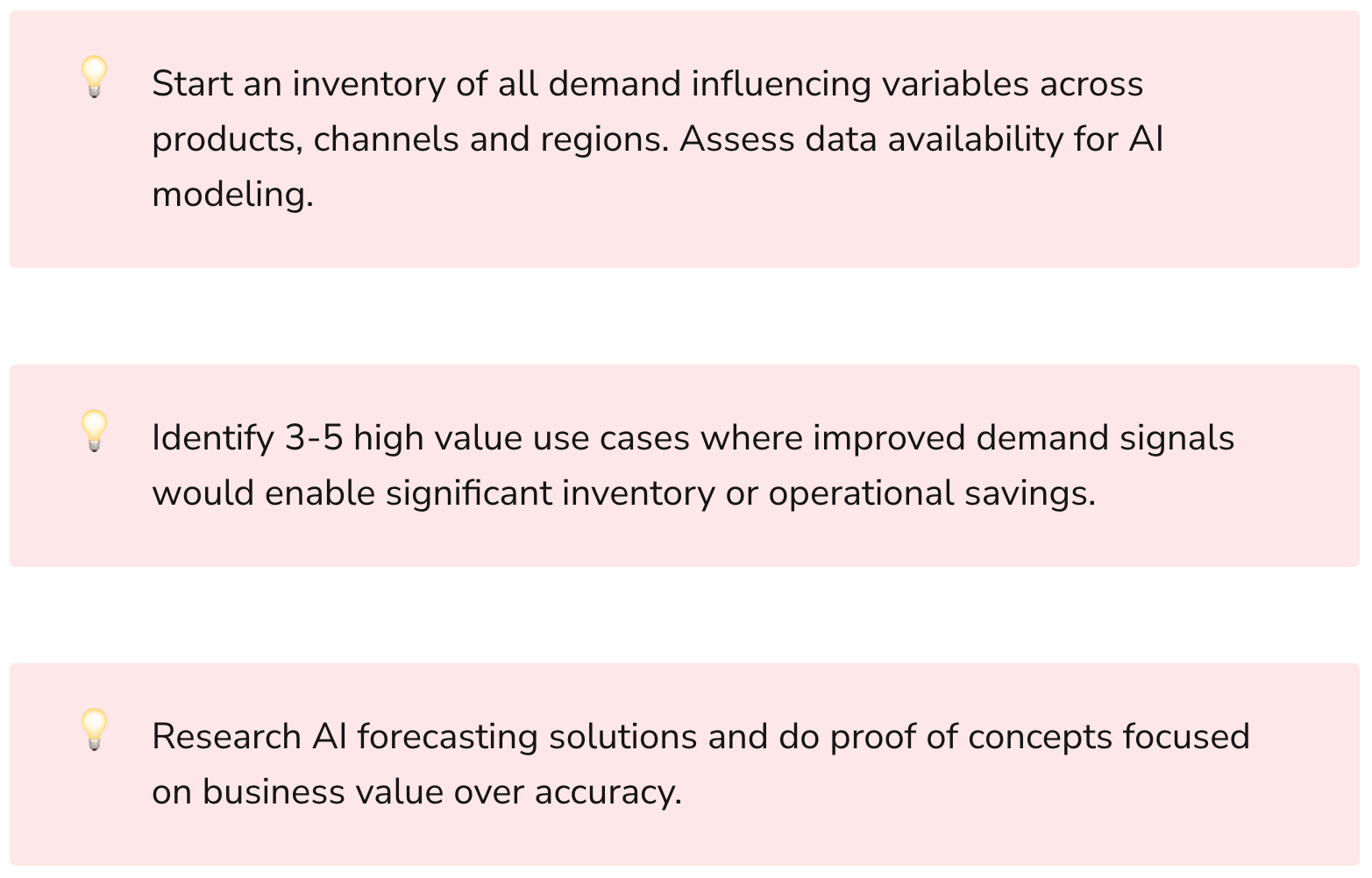
Demand forecasting is one of the most important yet challenging aspects of supply chain management. Without a clear sense of future demand, companies struggle to align inventory, production, and logistics plans. Poor demand visibility leads to stock outs, excess inventory, and missed revenue.

AI is transforming demand sensing and forecasting with machine learning algorithms that can analyze a variety of data to detect complex patterns. This provides a more accurate signal of future demand across product hierarchies and geographic regions.
For example, one of our customers is a major CPG company selling over 100,000 SKUs globally. By deploying our AI demand forecasting engine, they improved forecast accuracy by 19% within the first 8 months across their portfolio. This led to a 7% increase in product availability and $42 million savings in inventory carrying costs.

Some key ways AI improves demand forecasting include:
- Multivariate analysis: AI systems analyze thousands of internal and external variables that correlate with demand, including past sales, promotions, pricing, seasonality, weather data, competitive activity, market trends, events, and more. They automatically determine which drivers are most relevant and apply the optimal weighting to each.
- Continuous learning: Machine learning algorithms continuously ingest the latest data on sales and demand drivers to train on new patterns and improve predictive accuracy over time. Models are updated dynamically instead of relying on static assumptions.
- Granular projections: AI can forecast at very granular levels, down to the SKU-store level in retail or part number-region level in manufacturing. This prevents distortions from aggregation.
- Confidence metrics: The machine learning model outputs probability scores on forecasts to indicate the level of certainty. This allows planners to size buffer stock and contingency plans based on uncertainty.
- What-if simulation: Users can assess the demand impact of different scenarios by altering certain variables. For example, how would a 20% price drop for a product affect sales in Germany over the holidays? This allows for contingency planning.
With better visibility into future demand, companies using AI can significantly reduce inventory costs while improving service levels and planning agility.

Smarter Logistics Management
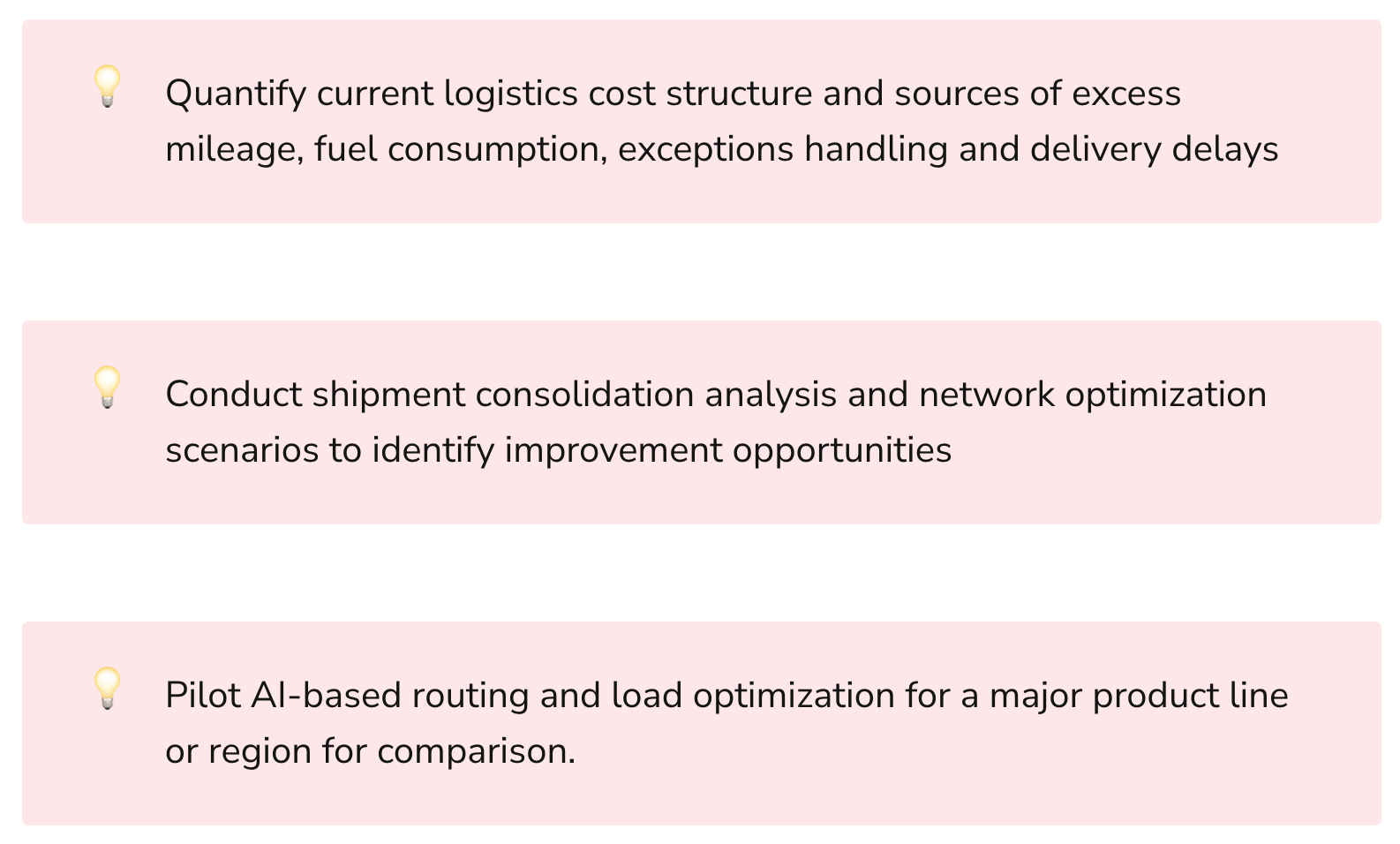
Logistics is another complex supply chain process involving global transportation networks, warehouses, carriers, routes, vehicles, delivery models, returns and more. Hundreds of variables are constantly in flux that impact decisions such as:
- What carrier and service level is optimal for each shipment?
- How can trucks be packed most efficiently to save transportation expenses?
- How should inventory be positioned across distribution centers to enable faster, lower-cost delivery?
Manual analysis would be insufficient with so many moving parts. AI and machine learning thrive at analyzing many variables and detecting optimal patterns.
For example, Google leverages AI to analyze billions of data points across routes, fuel, weather, traffic and more to recommend the most efficient paths for its delivery vehicles, reducing mileage by 10-15%. This significantly lowers fuel consumption and carbon emissions.
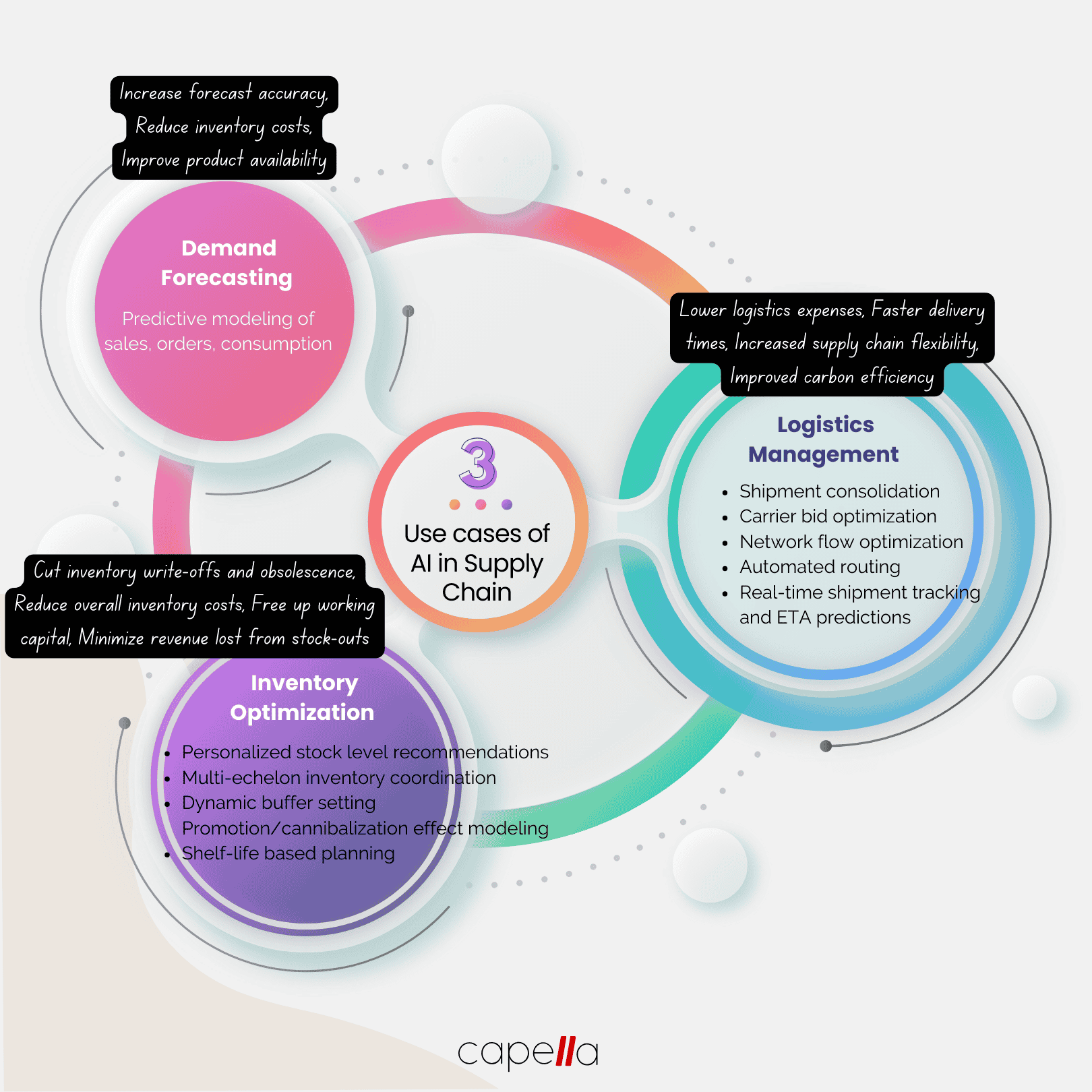
We worked with a medical supplies distributor who used our AI logistics engine to optimize inventory positioning, shipment routing and delivery consolidation across their network. This reduced their annual logistics expenses by 25% while enabling faster delivery times to hospitals and clinics.
Here are some ways AI drives logistics improvements:
- Network optimization: AI can balance tradeoffs across cost, delivery speed and other objectives to determine the optimal distribution of inventory, location of distribution centers, routing through transportation hubs, and flow through the logistics network. This leads to lower expenses and higher service levels.
- Shipment consolidation: The AI engine can analyze all outbound logistics demand across regions and determine the optimal way to consolidate shipments, balance truckloads and share containers to minimize per unit costs. This reduces miles traveled and carbon emissions.
- Automated routing: By processing billions of data points on weights, volumes, distances, traffic, fuel, weather and more, AI systems recommend optimal routes that balance timing, fuel costs, tolls and other factors to reduce mileage and logistics spend.
- Predictive ETAs: Machine learning tracks historical transit times across modes, carriers, and routes to predict estimated arrival times and update downstream production plans accordingly. This minimizes lead time surprises.
- Dynamic re-routing: When unexpected delays occur, the AI engine can assess downstream impacts across the network and reroute shipments in real-time to meet delivery SLAs at the lowest cost.
Leveraging AI and advanced analytics helps logistics teams improve on-time delivery, lower costs, reduce waste and enable faster responses to change. This leads to a competitive advantage.

Smarter Inventory Management

With supply chains facing so much volatility and uncertainty today, balancing service levels while avoiding excess and obsolete inventory is critical yet challenging. AI again provides tangible value here by detecting subtle demand signals, tracking inventory dynamics, and recommending data-driven stocking policies.
For example, one industrial manufacturer we work with was struggling with $100 million in surplus inventory. By deploying our AI inventory optimization solution, they identified $35 million of excess stock that could be safely drawn down over 8 months without impacting service levels. This freed up significant working capital.
Some key applications where AI drives inventory improvements are:
- Personalized forecasting: By analyzing every SKU's unique demand patterns and variability, AI forecasts demand and optimal stock levels tailored to each item instead of broader category averages. This prevents distortion from aggregation.
- Multi-echelon optimization: AI assesses demand signals, variability, and constraints across the entire supply network, including suppliers, manufacturing plants, distribution centers and end customers. This leads to optimal stock positioning across the chain.
- Automated buffer setting: Machine learning determines the right mix of cycle stock and safety stock customized for the demand profile, variability, and lead times of each SKU. This minimizes shortages while reducing excess buffers.
- Shelf-life based optimization: For perishable items, the AI engine considers remaining shelf life to dynamically adjust order quantities and clearance discount pricing to minimize waste from spoilage and expiration.
- Promotion and cannibalization modeling: AI builds models that capture how pricing, promotions, product introductions and retirements impact demand for each SKU and related items. This helps size orders and set inventory buffers at the right level.
The recommendations from AI systems enable supply chain teams to implement data-driven inventory policies tailored to the unique profile of each SKU. This optimizes service levels while eliminating unnecessary waste, holding costs and obsolescence across the network.
Real Business Impact
While AI and advanced analytics offer clear value on paper, what matters are real business outcomes. Across the nearly 100 enterprise deployments I have overseen at Capella, our customers have achieved remarkable ROI through AI-based supply chain improvements:
- Reduced inventory costs by 25-35%
- Improved product availability by 10-25%
- Lowered supply chain operating expenses by 20-30%
- Increased forecast accuracy by 20-50%
- Cut logistics costs by 15-25%
- Minimized inventory write-offs and obsolescence by 30-45%
These results speak for themselves on the ability of AI to drive automation, efficiency, and insights across forecasting, logistics and inventory management.
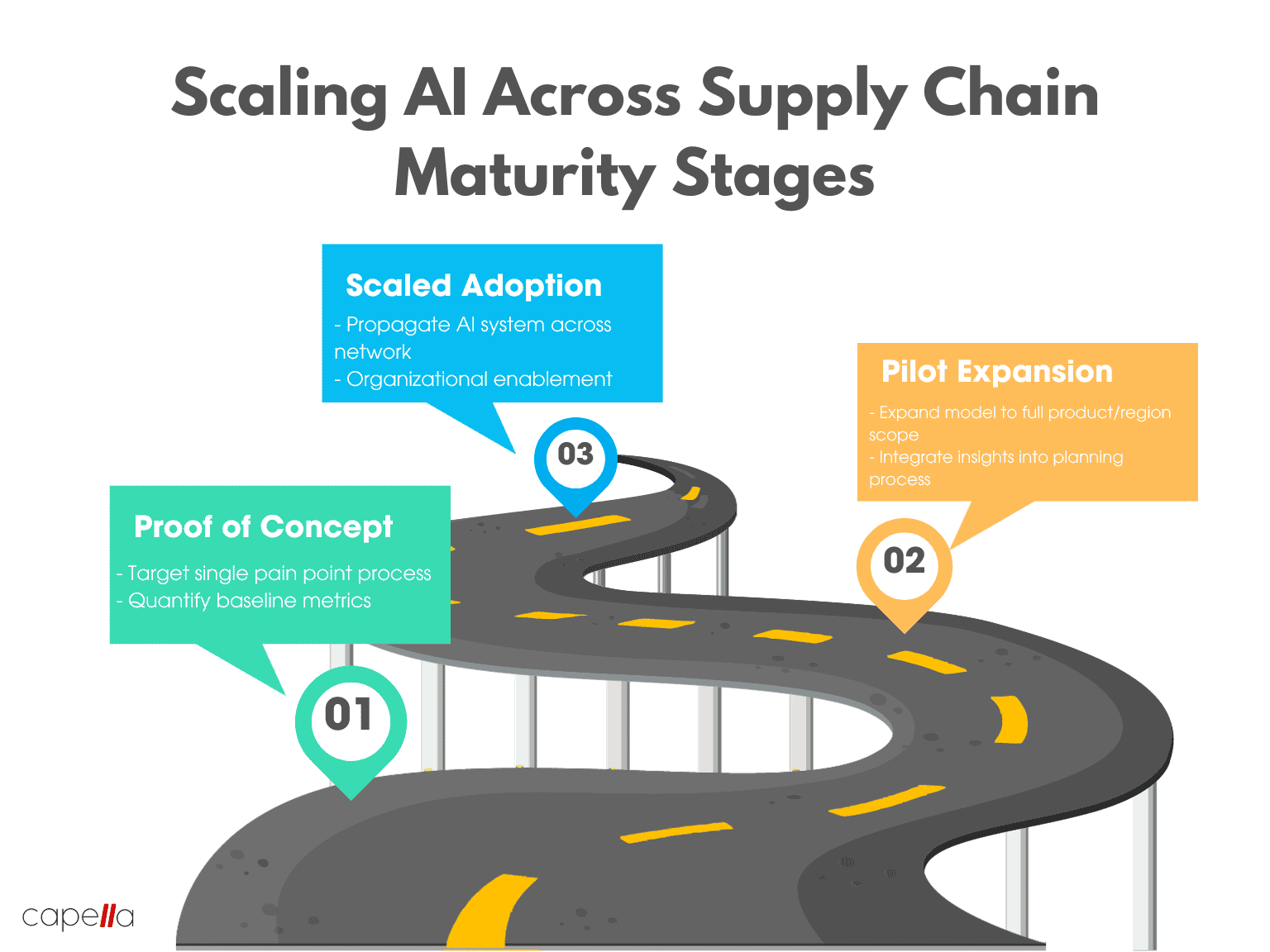
Scaling AI Across the Supply Chain
To achieve meaningful ROI, AI solutions cannot just be isolated proofs of concept or pilots. The key is scaling AI across the end-to-end supply chain to enable systemwide advancement.
Some recommendations on scaling AI adoption based on best practices I have observed:
Start with pain points: Prioritize the biggest areas of waste, inefficiency, and lack of reliability. Target quick wins to demonstrate value.
Take an end-to-end view: Assess how to capture data, apply AI and leverage recommendations across integrated planning – from suppliers to customers. Siloed solutions have limited impact.
Plan the organizational alignment: Ensure executive sponsorship, data science team support, line level adoption protocols and educational campaigns so AI integrates across operations.
Allow for organizational learning: Expect a period of learning, calibration and trust-building between the humans and algorithms. Enable feedback loops, so the AI models can continuously improve.
Focus on talent acquisition: Having data scientists work hand-in-hand with veteran supply chain experts is an imperative to translate technical advances into real operational results.
Monitor business KPIs: While technical accuracy of the AI model is important, shift focus to tangible outcomes against bottom-line profitability, cost efficiency and customer service targets.
The Future with AI
AI adoption in supply chain and operations is still early but quickly accelerating given the proven benefits. IDC predicts over 50% of large manufacturers will leverage AI-based logistics systems by 2025, while Gartner sees AI-based inventory optimizations improving margins by up to 90 basis points for retailers. Better forecasting algorithms also ranked high on supply chain executives wish lists in recent Adelante surveys.
While AI will not eliminate all variability and uncertainty, it will significantly tame turbulence through better sensing, optimization and orchestration. Leading enterprises are already differentiating through AI-based capabilities, hard for competitors to replicate overnight. Overreliance on legacy processes and institutional knowledge can lead to competitive declines.
Now is the time to educate, invest and pilot AI innovations across the supply chain. Early movers will build capabilities, talent pipelines and data foundations that fuel a multi-year advantage. I encourage supply chain leaders to envisage how AI can upgrade planning outcomes, empower teams with dynamic insights and enable higher service levels at lower costs.
The future for supply chain excellence is undoubtedly AI-powered. Will you lead the way?
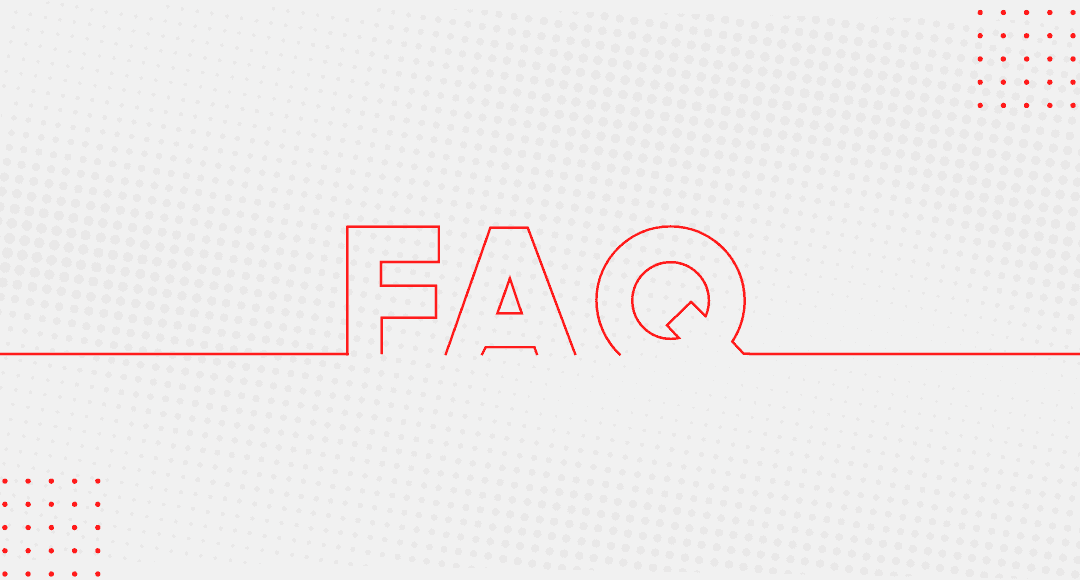
1. What are some common use cases for AI in supply chain management?
Some of the most common supply chain applications for AI include:
- Demand forecasting - AI analyzes hundreds of internal and external variables to create highly accurate demand predictions across products, segments, and geographies. This prevents distortion from aggregation in traditional statistical forecasting.
- Logistics optimization – AI engines can route shipments, pack trucks, optimize routes and choose carriers to minimize transit time and logistics costs. With so many interconnecting variables, AI can assess tradeoffs humans cannot manually process.
- Promotion and price modeling – By assessing correlations between pricing, discounts, feature introduction and demand over time, AI models the revenue lift or risk of promotions and cannibalization to optimize planning.
- Predictive inventory optimization – For each SKU, AI determines optimal cycle stock and buffer stock levels based on its demand variability, shelf life constraints and lead time elements. This minimizes waste from overage or lost sales.
- Intelligent replenishment – AI triggers automated purchase orders and stock transfers based on projected demand, current inventory and business rules customized at the product level. This prevents out of stocks while reducing over stocks.
2. How much ROI have companies achieved from AI supply chain projects?
Documented ROI statistics from AI supply chain initiatives include:
- 25-35% reduction in total inventory costs
- 20-30% lower supply chain operating expenses
- 15-25% drop in annual logistics spend
- 30-50% improvement in demand forecast accuracy
- 10-25% increase in product availability and service levels
- 20-45% less obsolescence and expired/spoiled stock
The efficiency gains, waste reduction and revenue lift from AI adoption have delivered remarkably high returns across use cases in 6-12 months averages.
3. What are some challenges of scaling AI across the supply chain?
Scaling AI comes with a few key challenges:
Data integration – Collating, cleansing and contextualizing data from across many disparate systems like ERPs, WMS, TMS and MES for AI consumption.
Talent gaps – Data science teams may lack supply chain experience while operations teams lack analytics skill sets. Cross-disciplinary pairing is key.
Organizational alignment – Executive leaders must communicate the AI vision while frontline teams should have protocols for assessing and adopting AI recommendations.
Legacy process adaption – Embedding insights from AI systems into complex planning workflows requires integration and alignment of inputs, outputs, and interfaces.
With program governance and change management, these barriers can be overcome to extend AI at scale.
4. How quickly can AI be implemented and show benefits?
The first proof of concepts focused on a clear pain point can produce benefits in as little as 8–12 weeks. One batch chemical company improved demand sensing accuracy by 30% across 20 top products in their initial AI pilot in just 10 weeks.
However, organization-wide implementations may take 6–9 months for meaningful scale. The keys are taking an iterative crawl-walk-run approach and showcasing quick wins first.
5. How do AI systems detect demand signals and predict trends?
AI leverages perceptual data across hundreds of demand indicators, applies correlation and causation analysis to identify key drivers, determines optimal weighting schemes per predictor, and develops multivariate non-linear models personalized for each product/segment.
By continuously ingesting data, the machine learning model trains itself to detect subtle pattern shifts early. This creates a more dynamic demand signal compared to traditional statistical forecasting reliant on stale assumptions.
6. Does AI optimize logistics better than humans?
AI can outperform even the savviest human planners in optimizing logistics, thanks to:
Hyperparameter optimization – AI simultaneously considers exponentially more routing options, transit modes, carrier bids and constraints to identify the most efficient options.
Continuous learning – The latest data on costs, traffic, obstacles, and delays trains the model to refine recommendations instantly.
Unbiased evaluation – Without political incentives or vendor affiliations, AI objectively detects choices delivering the lowest expense and highest quality.
Humans create intuitive rules of thumb. AI detects precise patterns across extreme complexity.
7. How does AI enable personalized inventory optimization?
Most traditional inventory techniques use broad classifications and hierarchical forecasting. However, AI creates a “profile” for every product that captures its demand patterns, variability, constraints, correlations, and cannibalization risks from substitutes at an individual SKU level. This allows truly personalized dynamic stock allocation, buffer setting and replenishment order generation based on projected demand for that specific item. Humans cannot replicate this level of personalization across thousands of SKUs.
8. How can companies start testing AI in their supply chains?
We recommend a crawl-walk-run approach:
Crawl – Assess 1-2 pain point operational areas, quantify potential ROI, gather datasets and run simulation scenarios
Walk – Probe AI recommendations against current decisions for 1-2 planning cycles and track business KPI impacts
Run – For validated high potential use cases, scale AI adoption across products/regions with change management protocols
Quick wins demonstrate tangible value while building trust and program momentum.
9. Which supply chain areas will be most disrupted by AI?
The more complex and fast-moving the decision-making, the higher the value potential from AI automation and intelligence. Areas seeing rapid AI innovation include:
- Demand volatility sensing and predictive modeling
- Load optimization, routing, and shipment consolidation
- Predictive maintenance and quality assurance
- Automated warehousing and logistics robots
As innovations propagate across the above areas over 5–10 years, human planners may transition towards more strategy, exception handling and optimization roles.
10. Are there risks related to supply chain AI adoption?
As with any technology, AI comes with some implementation risks to navigate:
Data errors – “Garbage in, garbage out” holds true. Bad data leads models astray. Rigorous data governance must embed.
Interpretability tradeoffs – The most accurate AI models have lower human explainability. Striking the right balance is key.
Model degradation – If not retrained on new data, model accuracy may erode over time due to dynamic system changes.
Job displacement concerns – AI should enable planners to focus on higher judgement tasks rather than fully replace roles. Proactive change management matters.
With mitigation practices, leading organizations are forging ahead with proven benefits.
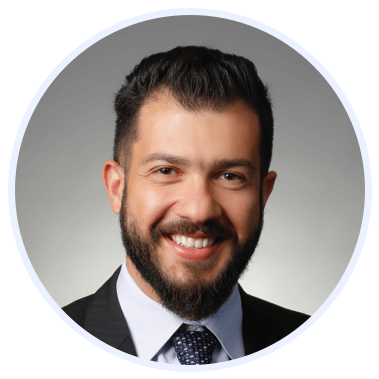
Rasheed Rabata
Is a solution and ROI-driven CTO, consultant, and system integrator with experience in deploying data integrations, Data Hubs, Master Data Management, Data Quality, and Data Warehousing solutions. He has a passion for solving complex data problems. His career experience showcases his drive to deliver software and timely solutions for business needs.