.png)
The loan application process can be tedious and time-consuming for both applicants and lenders. Verifying an applicant's creditworthiness is a critical step, but gathering all the required financial documents and calculating credit scores manually takes significant effort.
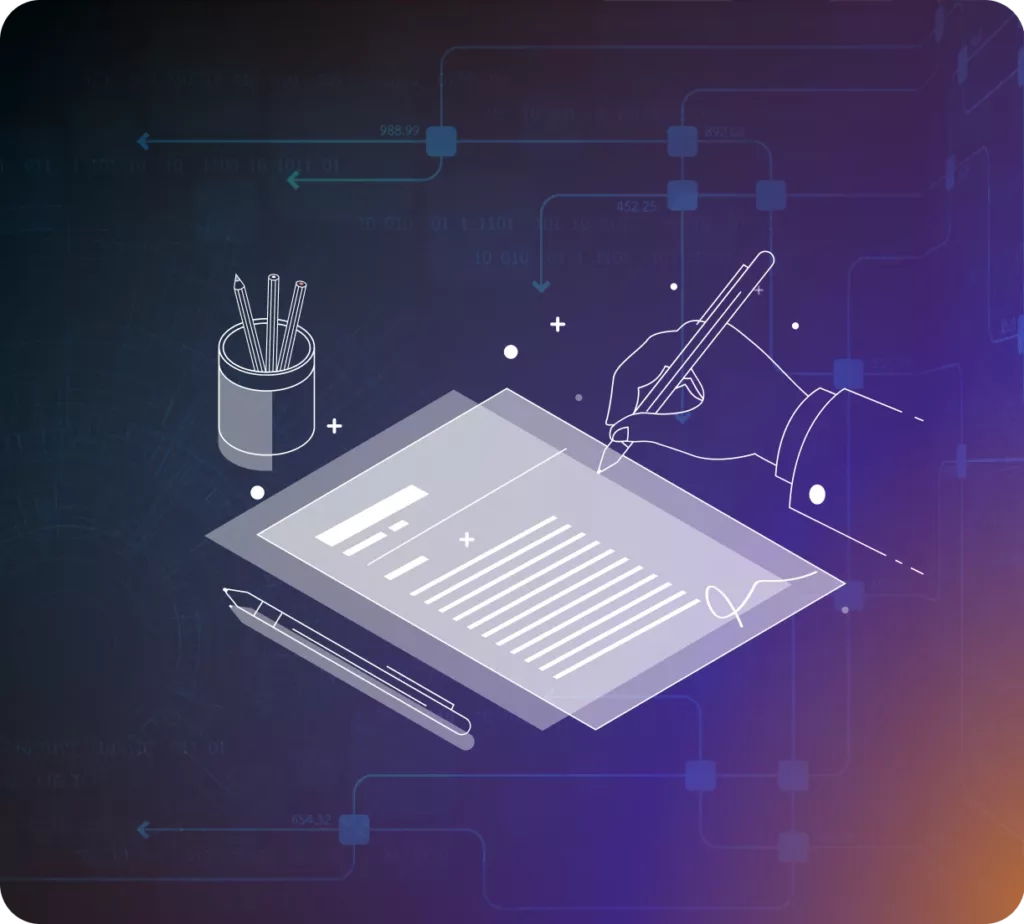
Banks and other lenders are increasingly looking to artificial intelligence (AI) and automation to make credit score reporting faster and more efficient. In this post, we'll explore the benefits of automating credit score reporting and review some of the latest AI techniques that are transforming loan processing.
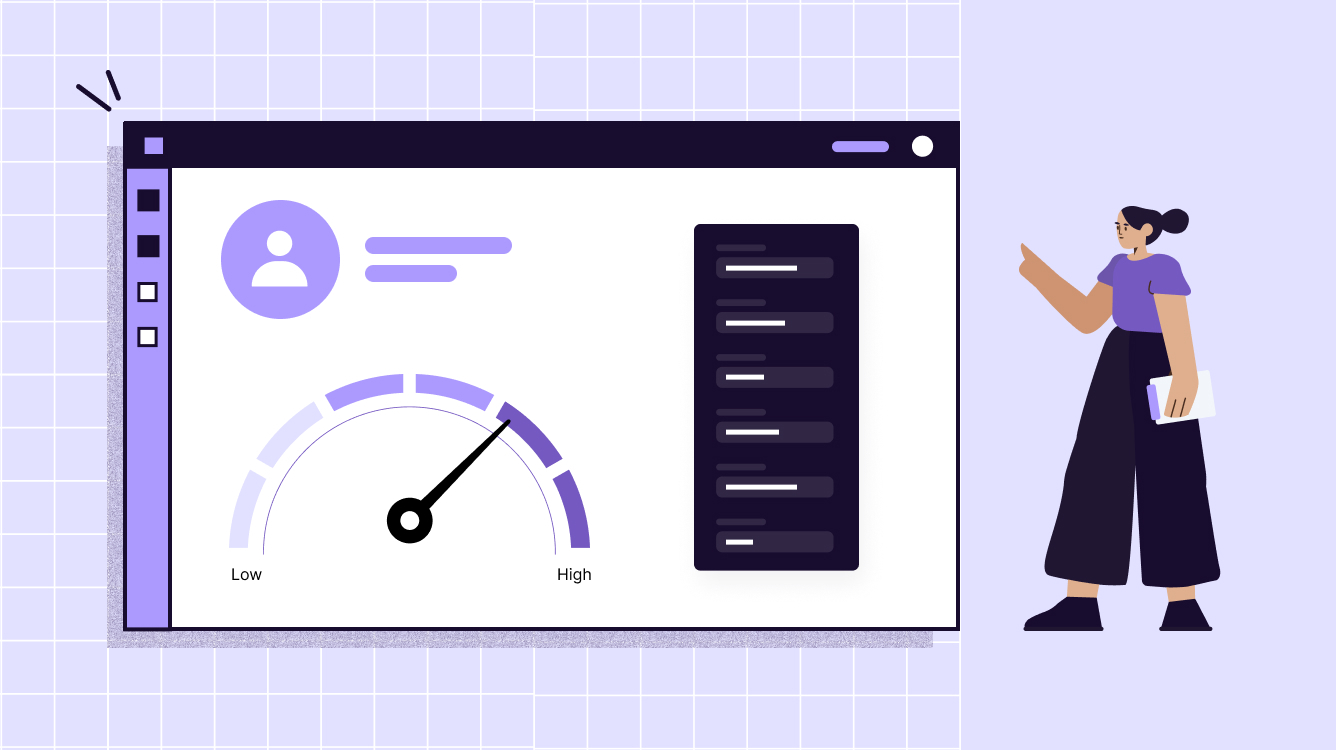
The Challenges of Manual Credit Score Reporting

Checking an applicant's credit score is essential for lenders to estimate their risk profile and make informed lending decisions. The traditional process involves:
- Requesting documents like bank statements, tax returns, and credit reports from the applicant
- Verifying the applicant's identity and employment status through external agencies
- Gathering credit history data from credit bureaus like Equifax, Experian, and TransUnion
- Calculating the credit score using algorithms like FICO or VantageScore
- Creating a credit report summary for the underwriter to review
This manual process can take anywhere from 2 days to 2 weeks. Delayed or inaccurate credit checks can frustrate applicants and lead to missed opportunities for lenders. Data entry errors can also creep in, leading to incorrect credit scoring.
On top of that, the sources of credit history data are fragmented. Lenders need to access consumer credit files across multiple bureaus and validate records across different systems. Important information can slip through the cracks.

How AI is Automating Credit Score Reporting

AI and automation technologies are emerging to make credit reporting seamless, near real-time, and highly accurate. Here are some of the top applications:

Instant Identity Verification
Verifying a loan applicant's identity is critical to prevent fraud. AI services like Jumio use computer vision and machine learning to automatically extract and validate ID information.
Applicants simply take a picture of their government ID. The service extracts fields like name, age, address, ID number, and photo in seconds and cross-checks them against external databases to confirm authenticity.
Automated Data Collection
Tedious financial document collection is being automated using optical character recognition (OCR) and natural language processing (NLP).
OCR scans documents like bank statements and tax forms to instantly extract key data fields. Smart logic built into the OCR handles variations in document formats across different institutions.
NLP algorithms like named entity recognition (NER) can read through unstructured text data like employment contracts to pull out key details on income, employment history, and more.
API Integrations with Credit Bureaus
Having to manually request and merge credit reports from different bureaus slows down the process.
Many lenders are now integrating directly with credit bureaus like Equifax and Experian through APIs. This allows instantly accessing applicant credit file data directly from the lender's loan origination system.
Credit bureau APIs give underwriters real-time access to applicant credit profiles. APIs also refresh data automatically if the credit file changes during loan processing.
AI-Powered Credit Scoring
Legacy credit algorithms are rules-based and lack flexibility. AI credit risk models are emerging that can continuously learn from new applicant data patterns.
These AI scoring engines ingest wider alternative data like utility payments, rent history, education, and employment patterns. This gives a more holistic view of creditworthiness.
Models are tuned using techniques like logistic regression and neural networks to refine credit scoring accuracy. AI allows easily tweaking models for different lending products.
Automated model retraining allows improving accuracy over time as more loan application data comes in.

Benefits of Automated Credit Score Reporting

Automating the credit score reporting process with AI delivers powerful benefits:
- Faster credit decisions - Instant identity verification and data collection accelerates the applicant experience. Credit risks can be assessed within hours rather than days.
- Higher application conversion - Reduced drop-off from long application processes. Automation provides a smooth lending experience.
- Lower costs - Less employee time needed for manual verification and data entry. AI systems scale easily with application volumes at a lower cost.
- Minimized fraud - Automated identity checks and API data integration prevents manipulation of records.
- Improved model accuracy - AI credit scoring builds more predictive models by continuously learning from new data.
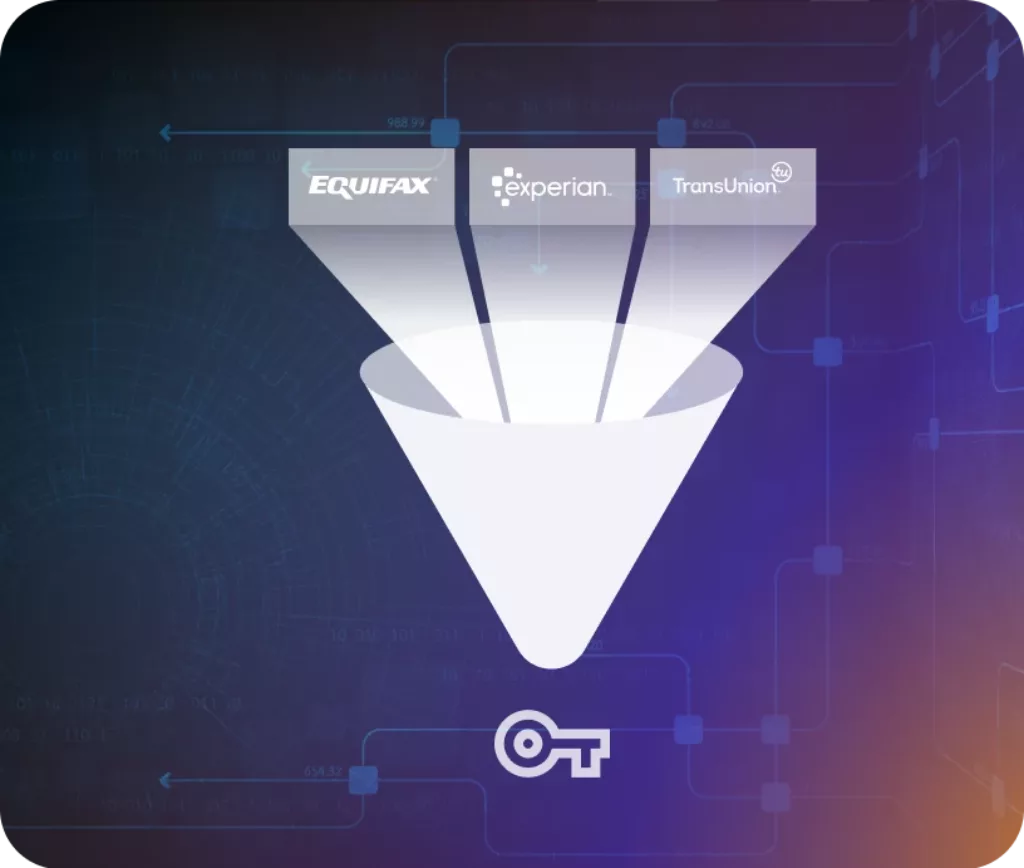
Let's look at a case study showing the impact of implementing automated credit score reporting.
Case Study: SlickCash Loan Approvals
SlickCash provides short-term personal loans and was experiencing high applicant dropout due to lengthy verification processes.
By integrating Jumio for ID verification and OCR tools to extract bank statements, loan approval time dropped from 5 days to 1 day.
API integration with Equifax credit data further reduced approval time to just 2 hours in most cases.

Automation led to faster service, increased approvals, and higher productivity for SlickCash.
Implementing Automated Credit Score Reporting

Here are some best practices for lenders looking to implement automated credit score reporting:
- Evaluate AI software - Assess different vendor solutions for identity verification, credit data APIs, OCR, and credit scoring models. Prioritize accurate and compliant solutions.
- Start with a pilot - Run a controlled pilot program across part of your loan portfolio to test effectiveness. Measure results against key metrics like approval time, conversion, and costs.
- Integrate with core systems - Ensure tight integration between the AI systems and your existing loan origination and credit scoring software. Smooth interoperability is essential.
- Monitor for bias - Credit scoring models should be regularly monitored and tested for algorithmic bias. Collect feedback across underwriters and tweak models to ensure fair decisions.
- Retrain models periodically - Refresh AI credit scoring models on new loan application data every 6-12 months. Continuously adapt to new lending patterns.
The Future of Automated Credit Scoring
Fully automated and real-time credit decisioning is on the horizon. Here are some innovations we can expect:
- Wider range of alternative data from sources like education, licenses, and behavioral patterns used to build 360-degree applicant profiles
- Tighter integration between identity verification and credit checking will allow instant decisions with minimal applicant data input
- More predictive modeling techniques like gradient boosting, random forests, and deep learning applied to credit risk modeling
- Federated learning to build shared models across lenders while keeping applicant data secure
- Embedded finance allowing credit decisions and lending from within everyday apps and services
Automated credit score reporting will transform loan origination. Lenders that leverage these innovations will be able to meet consumer expectations for speed and convenience. They'll also be able to expand access to credit by broadening data sources and powering innovative lending products.
Key Takeaways
- Manual credit reporting processes are slow, expensive, and prone to errors
- AI innovations in identity verification, data extraction, credit APIs, and predictive modeling are automating credit analysis
- Automated reporting improves applicant experience, reduces costs, and minimizes fraud
- Lenders should start with controlled pilots of AI tools and closely track impact on approval times, conversion, and underwriting productivity
- Continued improvements in alternative data analysis and predictive algorithms will enable real-time automated credit decisions
By harnessing AI to automate credit score reporting, lenders can accelerate approvals, reduce costs, and scale decision-making - all while providing consumers with the speed and convenience they demand. The future looks bright for accessible and responsible lending powered by smart financial automation.
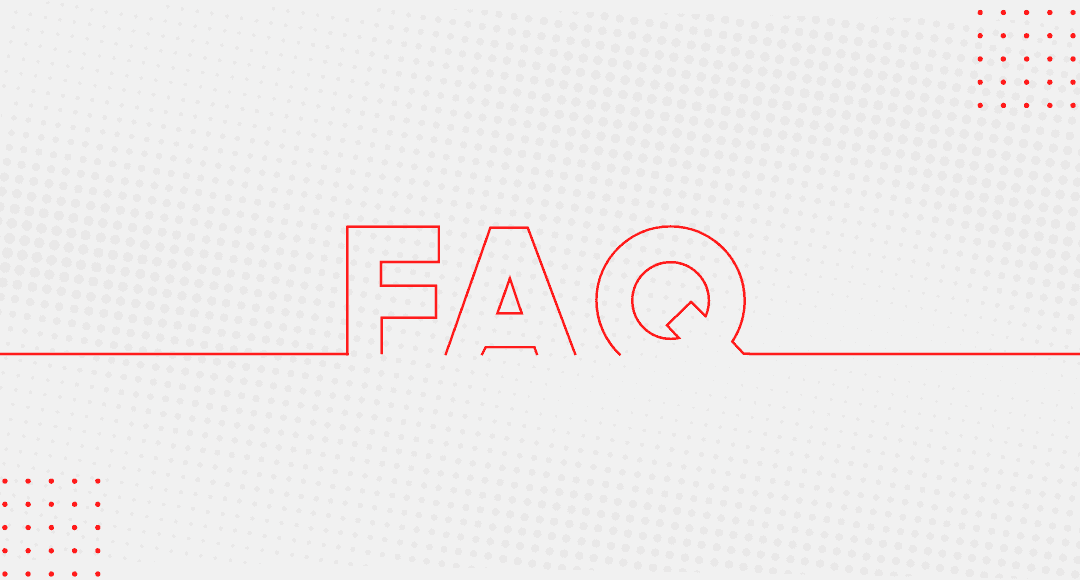
What are the main benefits of automating credit score reporting for lenders?
Automating the credit score reporting process delivers several advantages for lenders:
- Faster application decisions - Automation like instant identity verification and API credit data integration significantly reduces the time required to assess applicants, from days to just hours. This improves customer satisfaction.
- Higher conversion rates - With a smoother and faster application process, fewer applicants will drop off and more will complete their applications. This directly improves loan volumes and revenue.
- Lower costs - Automating repetitive manual tasks reduces staffing costs for data entry and verification roles. Fixed costs of AI systems are also less than growing human teams.
- Minimized fraud - Automatic identification checks and seamless data aggregation makes it much harder for applicants to falsify records. This reduces overall lending risk.
- Improved accuracy - AI credit scoring algorithms become more predictive over time as they continuously learn from new applicant data. This results in better lending decisions.
What are some challenges in implementing automated credit score reporting?
Key challenges faced by lenders when implementing credit automation include:
- Integrating new AI systems with existing loan origination software and workflows
- Training staff to work alongside automated components like identity verification
- Curating accurate training data to develop high-performing AI credit scoring models
- Monitoring and mitigating algorithmic bias issues in predictive models
- Handling model explainability and compliance requirements for AI systems
- Retraining models regularly with the latest applicant data to keep accuracy high
Careful change management and governance is crucial to address these challenges during automation.
How can alternative credit data improve lending decisions?
Alternative credit data such as rent payments, utility bills, mobile phone subscriptions, and education details can complement traditional credit reports to give lenders a fuller picture of an applicant's creditworthiness. Benefits include:
- Helping new credit customers with limited traditional history qualify for loans
- Enabling customized lending decisions tailored for specific applicant segments
- Identifying responsible borrowers missed by traditional credit scoring
- Detecting early warning signs of financial trouble not visible in mainstream credit data
However, alternative data must be used judiciously to avoid unfairly disadvantaging certain demographics.
What AI techniques are best suited for credit risk modeling?
AI techniques that excel at predicting default probability from applicant data are ideal for credit modeling, including:
- Logistic Regression - Simple and fast to train, easily handles multiple risk factors
- Random Forests - More robust against overfitting, handles complex variable interactions
- Neural Networks - Can model highly non-linear relationships, though complex to tune
- Gradient Boosted Trees - Flexible, avoids overfitting, and stable predictions
Key is choosing models that balance accuracy, explainability, and rapid re-training on new data.
How can lenders ensure AI credit decisions are fair and ethical?
Maintaining fair AI systems takes diligence across the model development pipeline:
- Monitor training data distributions by applicant demographics to ensure balanced representation
- Perform statistical tests for biases along gender, age, ethnicity before deploying models
- Enable human-in-the-loop reviews to check for unfair model predictions
- Continuously collect feedback data across underwriters and impacted demographics
- Keep iterating models to minimize unintended biases that emerge over time
Ongoing model reviews, bias testing, and training data audits are crucial.
What privacy protections apply when tapping into credit bureau data?
Lenders must respect applicant privacy when handling credit information:
- Only collect applicant-authorized credit data required for lending decisions
- Anonymize applicant names/identifiers before storing credit data
- Encrypt credit data end-to-end, only decrypt during active processing
- Limit employee access to credit data only on a need-to-know basis
- Have stringent data deletion policies post decisioning to minimize retention
Staying compliant with laws like GDPR and CCPA is a must when managing credit data access.
How can lenders start small with credit automation pilots?
Starting with a focused pilot makes automation less risky:
- Automate identity verification first for faster applicant onboarding
- Pick a specific loan product and start with API integration to credit bureaus
- Develop AI scoring model just for that product using relevant training data
- Compare model decisions vs underwriter decisions before full deployment
- Slowly expand scope to more products as models mature
Targeted pilots allow demonstrating benefits and building confidence before going all-in.
What are emerging innovations that will shape the future of credit automation?
Upcoming innovations that will reshape credit automation include:
- Alternative data from more diverse sources (social, IoT, blockchain) for richer applicant profiles
- Federated learning for collaborative modeling across lenders without exposing raw data
- Differential privacy and synthetic data to keep applicant data protected
- Quantum machine learning for exponentially faster training of complex models
- Embedded credit decisions directly within relevant commercial workflows
As these technologies mature, the speed and sophistication of automated credit analysis will rapidly improve.
How can lenders ensure responsible use of automated credit scoring?
Using AI responsibly for credit decisions means:
- Making fairness and transparency core principles guiding automation
- Rigorously testing models for demographic biases before and after deployment
- Providing explainability into model logic and credit decisions for applicants
- Enabling applicants to correct inaccurate data that may adversely impact them
- Having an efficient appeals process for applicants to contest unfavorable decisions
- Continuously improving models to minimize unintended disadvantages
Automation should augment but not replace human oversight and diligent governance.
What skills are required to maintain and operate automated credit scoring systems?
Key skills needed to effectively run AI credit operations include:
- Data engineering to aggregate quality dataset for model training
- Machine learning expertise for model development, tuning and testing
- Cloud and DevOps skills to reliably deploy models and APIs
- Cybersecurity knowledge to lock down credit data access and integrations
- Product sense to refine systems based on applicant feedback
- Communication skills to explain model decisions to applicants
- Ethics training to ensure fairness objectives are met
A cross-disciplinary team is required to make credit automation impactful yet responsible.
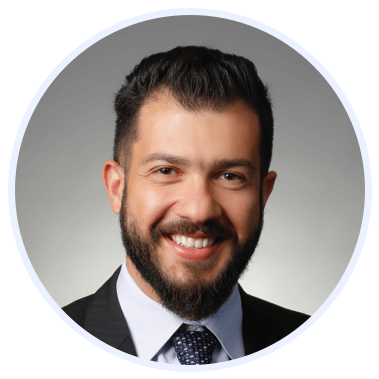
Rasheed Rabata
Is a solution and ROI-driven CTO, consultant, and system integrator with experience in deploying data integrations, Data Hubs, Master Data Management, Data Quality, and Data Warehousing solutions. He has a passion for solving complex data problems. His career experience showcases his drive to deliver software and timely solutions for business needs.