
As the world around us continually mutates into an unending stream of data, the ability to leverage predictive analytics in making informed business decisions is transforming from a luxury to a necessity. But don't panic, because there's an intriguing proposition on the horizon: low-code tools.
These tools democratize access to analytics by making it accessible to non-developers. How might you ask? In the same way the elevator made the penthouse accessible to those not keen on climbing 50 flights of stairs.
In this blog, we'll dive deep into the world of low-code tools and how you can use them to build your predictive analytics system. But first, let's get everyone on the same page.

Predictive Analytics: The Crystal Ball of Business Decision Making

Predictive analytics is the practice of extracting information from existing data sets to determine patterns and predict future outcomes and trends. In other words, it's as close as we've come to a business crystal ball.
Imagine being able to anticipate customer churn, forecast sales, and even predict maintenance issues before they happen. That's the power predictive analytics brings to your enterprise.
What are Low-Code Tools?
Low-code tools are software platforms that provide graphical user interfaces for programming and thereby reduce the amount of hand-coding necessary. In essence, they're the shortcut to creating business applications without the need to become fluent in Python or R.
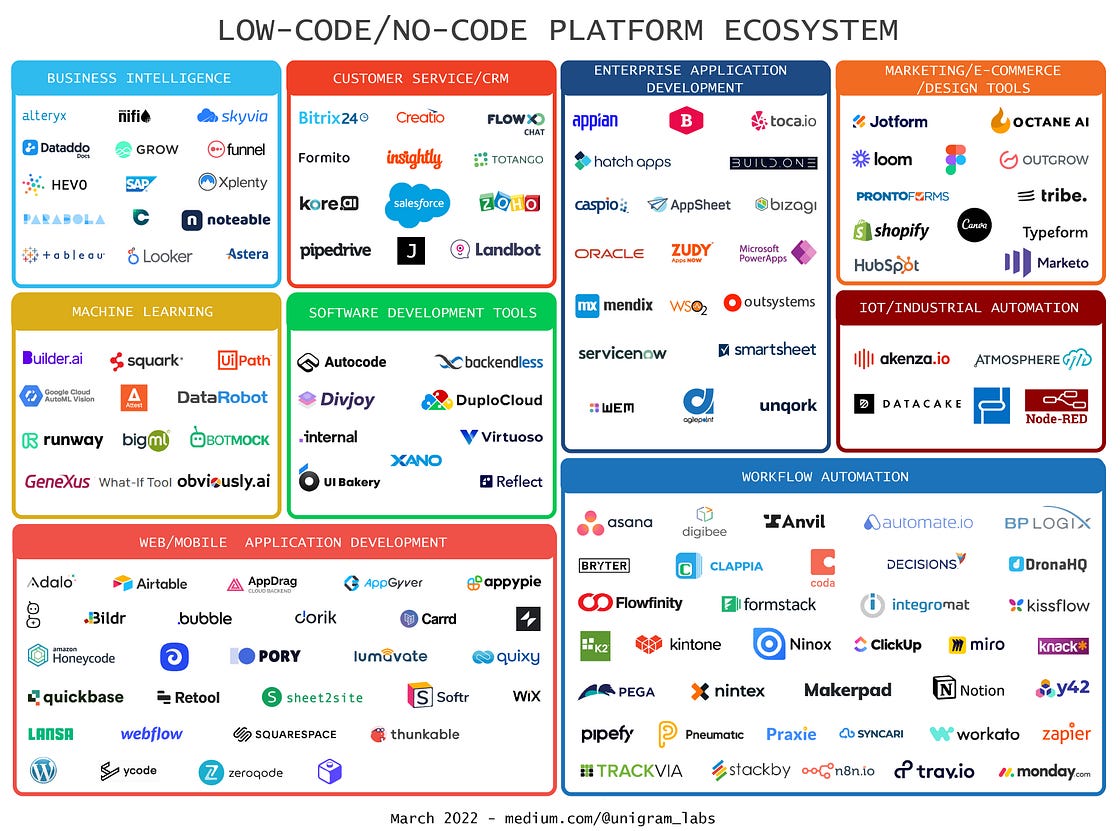
Low-code platforms provide pre-built components (think of them as Lego blocks) that you can use to build your applications. According to Gartner, over 50% of all medium and large-sized organizations were expected to use a low-code development platform as one of their main application platforms by 2023. This was primarily due to the need for simpler and faster application development. Low-code platforms empower both professional developers and non-developers to build complex enterprise solutions that are easier to integrate, modify, and upgrade, allowing organizations to adapt more quickly to changes in the market, customer needs, and even legislation.
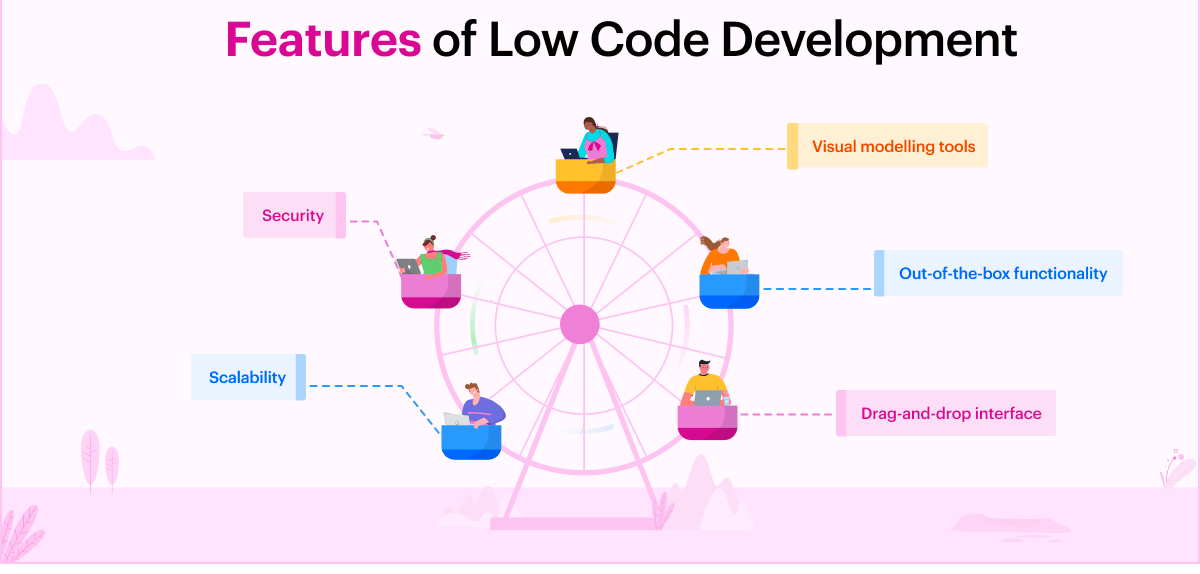
Why Should You Care?
Speed: Low-code tools can significantly speed up the development process, which is critical in the fast-paced world we live in.
Accessibility: They make predictive analytics accessible to a broader range of people within your organization, not just the data scientists.
Cost-effective: They can reduce the costs associated with hiring specialized software developers.
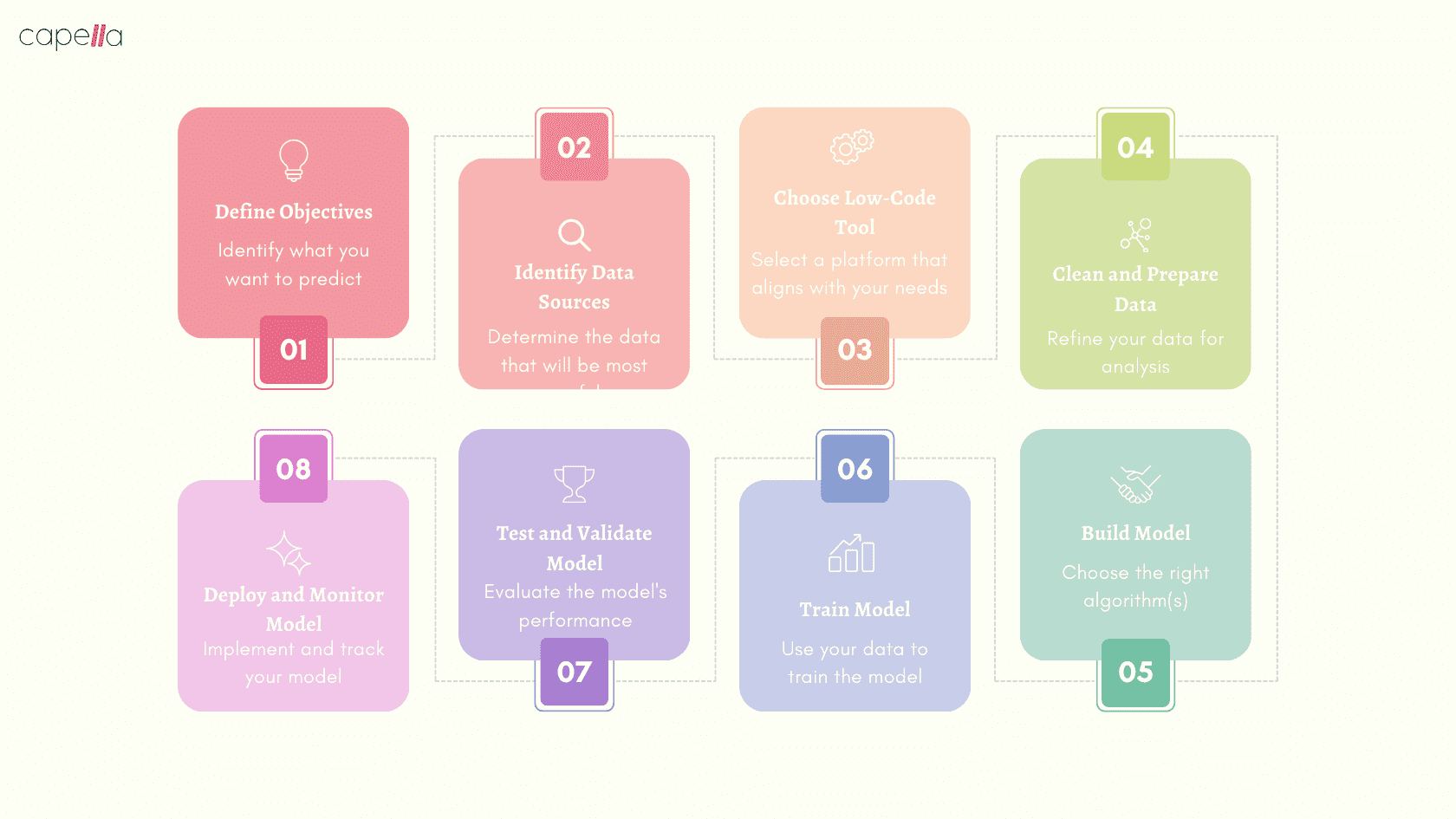
Building a Predictive Analytics System with Low-Code Tools: A Step-by-Step Guide
Now, let's get down to the nitty-gritty of how you can build a predictive analytics system using low-code tools. Buckle up because we're about to embark on an insightful journey.
Step 1: Define Your Business Objectives
Before you start building anything, you need to clearly define your business objectives. What do you want to predict? What actions will you take based on those predictions? The answers to these questions will guide your entire project.
Step 2: Identify Your Data Sources
You can't make predictions without data. Identify the data sources that will be most useful in achieving your business objectives. This could be anything from your CRM system to your social media analytics.
Step 3: Choose Your Low-Code Tool
Now that we understand the rising prominence of low-code tools, it's time to pick one that aligns with your business needs. There's a wide array of platforms available, each offering a unique blend of features. When choosing, consider factors such as ease of use, integration capabilities, scalability, and of course, cost.
Step 4: Clean and Prepare Your Data
Data is the fuel for your predictive analytics engine. But like all fuels, it needs to be refined before it can be used. This process involves cleaning the data to remove errors and inconsistencies, followed by transforming it into a format that can be used for analysis. Low-code platforms typically offer tools to simplify this process, but the quality of your data is ultimately your responsibility.
Step 5: Build Your Model
With your clean, prepared data in hand, it's time to build your predictive model. This involves selecting an algorithm (or several) that will be used to make predictions. Don't let the word 'algorithm' intimidate you, though. In the world of low-code, these algorithms often come in the form of drag-and-drop components.
Step 6: Train Your Model
Once your model is built, it needs to be trained using your data. This is where your model learns the patterns and relationships in the data that will allow it to make predictions. The good news is that many low-code platforms automate much of this process. Still, it's important to monitor the training to ensure your model is learning effectively.
Step 7: Test and Validate Your Model
After your model has been trained, it's time to test it. This involves running your model on a separate set of data (known as a validation set) to see how well it performs. It's like the final exam for your model. If it performs well, it's ready to graduate to the next step. If not, it may need more training or adjustments.
Step 8: Deploy and Monitor Your Model
Congratulations, your model is ready for the big leagues. It's time to deploy it and start making predictions. But don't just set it and forget it. Be sure to monitor your model's performance over time. Like a fine wine, models can get better with age, but they can also go bad if not properly cared for.
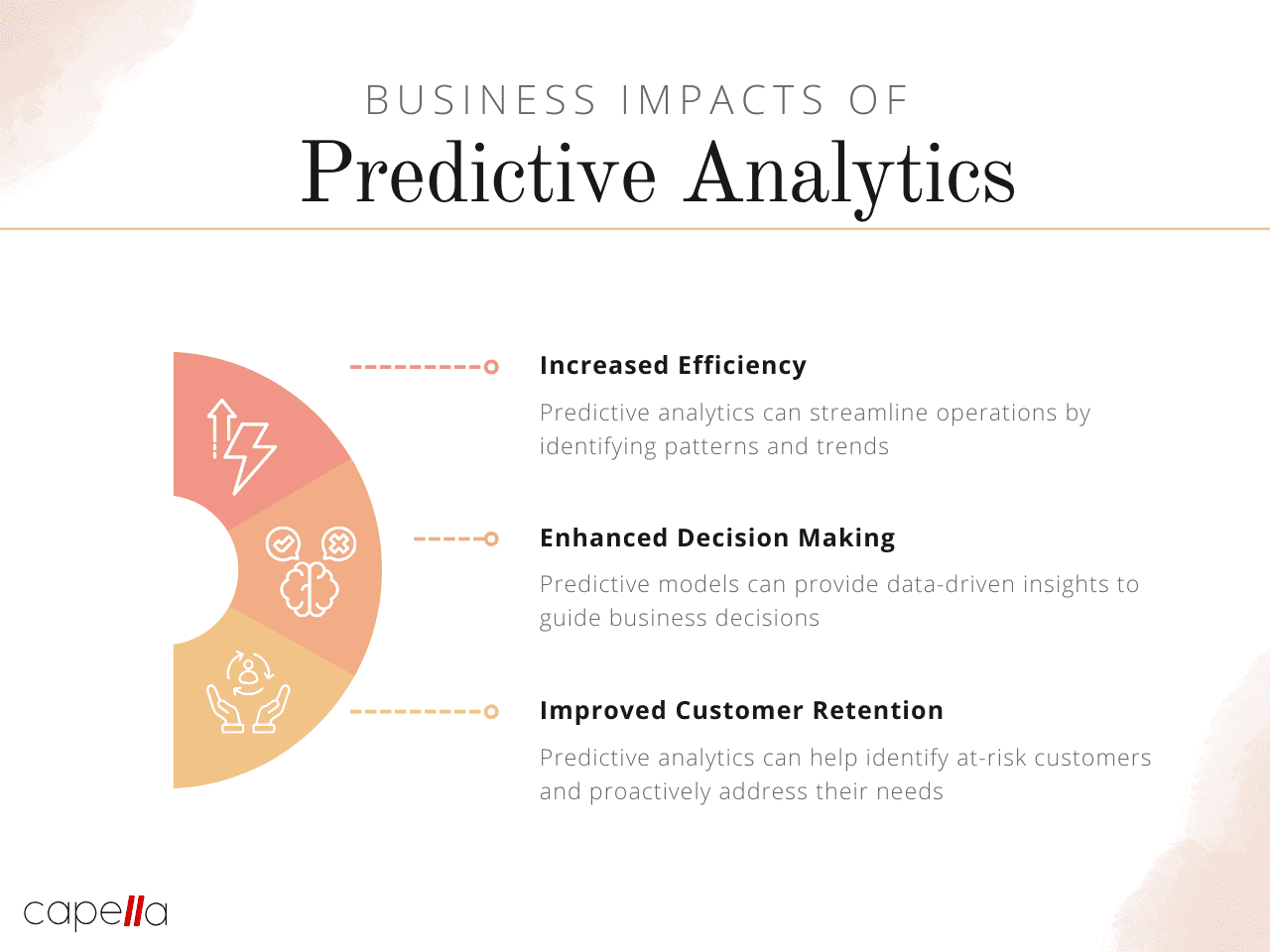
Practical Examples: Low-Code Predictive Analytics in Action
Let's bring these steps to life with a couple of practical examples.
Example 1: Predicting Customer Churn
Imagine you're a telecom company struggling with customer churn. By using a low-code tool, you can quickly build a predictive analytics model using your customer data. This model can identify patterns and signs of a customer likely to churn. With this information at hand, you can proactively reach out to these customers, address their issues, and hopefully, keep them from leaving.
Example 2: Forecasting Sales
Or perhaps you're a retail company looking to better predict your sales. With a low-code tool, you can use past sales data, along with other factors such as seasonal trends and promotional activities, to forecast future sales. This can help you better manage inventory, staffing, and other resources.
The Future is Low-Code
The rise of low-code tools is democratizing access to predictive analytics, making it accessible to a broader range of people within organizations. Whether you're a seasoned data scientist or a business analyst, low-code platforms offer a faster, more accessible, and cost-effective way to harness the power of predictive analytics.
Remember, the journey of a thousand miles begins with a single step. And the journey to predictive analytics begins with choosing a low-code tool. So why wait? The future is low-code, and the future is now.
The Power of Predictive Analytics at Your Fingertips
In the world of business, the ability to look ahead and make informed decisions is a game-changer. With predictive analytics and low-code tools, the power to make data-driven predictions is at your fingertips. Whether it's predicting customer churn, forecasting sales, or optimizing operations, predictive analytics can provide the insights you need to drive business success.
The beauty of low-code tools is their accessibility. They allow individuals and teams, regardless of their technical abilities, to create powerful predictive models that can provide valuable insights. By removing the barriers to entry, low-code tools are enabling more businesses to leverage the power of predictive analytics.
In this age of digital transformation, businesses that are quick to adapt and innovate will be the ones that thrive. With low-code tools, you can fast-track your business transformation efforts through faster application delivery, becoming digitally proactive and data-driven.
In conclusion, the world of predictive analytics is no longer just for data scientists and IT experts. With the right low-code tool, anyone can tap into the power of predictive analytics, driving business growth and staying ahead of the curve.
Now, as we wrap up this discourse, I leave you with this thought: The future is not to be feared, but to be anticipated. Predictive analytics is the compass that lets you navigate the vast sea of possibilities that lie ahead. Harness its power, and steer your enterprise towards the shores of success.
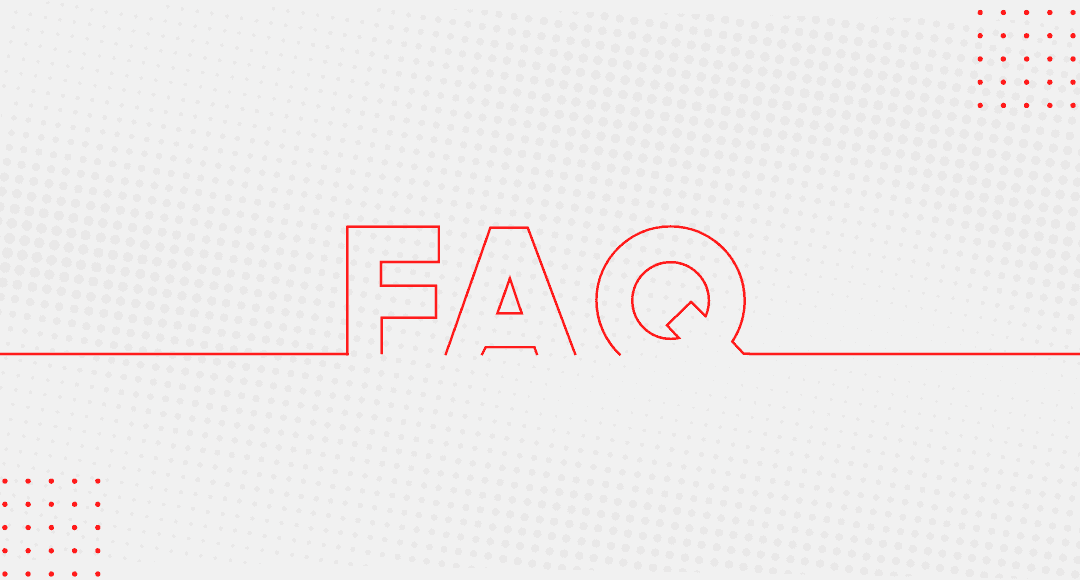
1. What is predictive analytics?
Predictive analytics is a branch of advanced analytics that uses historical data, statistical algorithms, and machine learning techniques to identify future outcomes based on data patterns. It doesn't tell you what will happen in the future; rather, it forecasts what might happen with an acceptable level of reliability, and includes what-if scenarios and risk assessment.
2. What are low-code tools?
Low-code tools are software development platforms that enable rapid application delivery with minimal hand-coding, and quick setup and deployment. They provide pre-built components (like Lego blocks) that you can use to build your applications. These platforms empower both professional developers and non-developers to create complex enterprise solutions that are easier to integrate, modify, and upgrade.
3. Why combine predictive analytics with low-code tools?
Combining predictive analytics with low-code tools brings the power of advanced data analysis to the hands of business users and analysts who may not have a deep technical background. This combination allows businesses to quickly build and deploy predictive models, leading to more agile decision-making and better business outcomes.
4. How can I start building a predictive analytics system with low-code tools?
Building a predictive analytics system involves several steps, including defining your objectives, identifying your data sources, choosing your low-code tool, cleaning and preparing your data, building your model, training your model, testing and validating your model, and finally, deploying and monitoring your model.
5. What type of data is needed for predictive analytics?
Predictive analytics can work with a wide variety of data types. This includes structured data (e.g., customer age, income, previous purchases) and unstructured data (e.g., customer reviews, social media posts). The key is to have enough historical data that can be used to train the predictive model.
6. How do I choose the right low-code platform for my business?
Choosing the right low-code platform depends on several factors such as your business needs, the technical skill level of your team, the platform's ease of use, its integration capabilities, scalability, and cost. It's recommended to research different platforms, try out demos, and consider reviews from other users before making a decision.
7. What are some practical examples of predictive analytics?
There are numerous practical examples of predictive analytics across industries. For instance, in retail, predictive analytics can be used to forecast sales, manage inventory, and optimize pricing. In the telecom industry, it can be used to predict customer churn. In healthcare, it can be used to predict disease outbreaks or patient readmissions.
8. What are the benefits of using low-code tools for predictive analytics?
Low-code tools make predictive analytics more accessible by reducing the need for extensive coding knowledge. They allow for faster development and deployment of predictive models, encourage innovation by making it easier to test and iterate models, and can lead to cost savings by reducing the need for specialized personnel or outsourcing.
9. How does predictive analytics fit into a larger business strategy?
Predictive analytics can be a crucial part of a larger business strategy by providing insights that inform decision-making. It can help businesses anticipate customer needs, identify potential risks, optimize operations, and ultimately, drive profitability and growth.
10. How can I ensure the accuracy of my predictive analytics system?
Ensuring the accuracy of your predictive analytics system involves regularly testing and validating your model, using clean and relevant data, and continuously monitoring the model's performance after deployment. It's important to remember that predictive analytics doesn't provide absolute certainty about the future, but rather, probable outcomes based on historical data. Regular reviews and adjustments of the system are also key to maintaining its effectiveness.
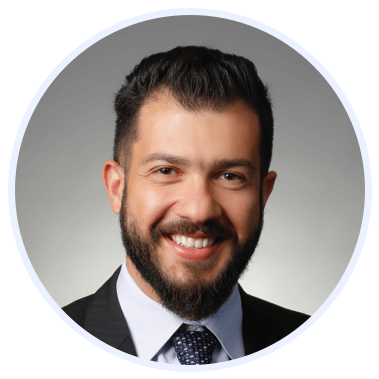
Rasheed Rabata
Is a solution and ROI-driven CTO, consultant, and system integrator with experience in deploying data integrations, Data Hubs, Master Data Management, Data Quality, and Data Warehousing solutions. He has a passion for solving complex data problems. His career experience showcases his drive to deliver software and timely solutions for business needs.