.jpg)
The most important component of any business intelligence strategy is data quality. Creating systems for information distribution and collection is one thing, but if the data is corrupted or unreliable, it defeats its purpose. Therefore, data cleaning is necessary regardless of the industry a business operates in or the type of data it collects.
Why is data purification important to businesses? Well, think of it as spring cleaning. Over time, clutter accumulates, and those difficult-to-reach areas become dusty. Even though dust cannot be seen with the naked eye, it still causes minor symptoms like allergies. Data can be viewed in the same way.
Small clusters can become corrupted, out-of-date, or incorrect. Minor symptoms then begin to interfere with your company's day-to-day operations, even if they are challenging to see. Eventually, those problems worsen if no action is taken.
That’s where data cleaning comes in. It’s the process of removing errors, redundancies and other anomalies from databases to ensure accuracy. With data cleaning, businesses can rest assured they have reliable information to make informed decisions and stay ahead of the curve.
But to help you better understand the data cleaning process and why it's important for enterprise businesses, let’s explore the following topics in more detail:
- Why is data cleaning important for enterprise businesses?
- What is the goal of data cleaning?
- What are the steps involved in data cleaning?
- Components of quality data

Why Is Data Cleaning Important For Enterprise Businesses?
Data cleaning is an essential process for enterprise businesses as it ensures that the data they are using is accurate, valid, and consistent. With the ever-increasing use of data in business operations.
Data cleaning can help enterprise businesses identify and resolve any errors in their data and ensure that their data is up-to-date and ready to be used. By ensuring the data is accurate, businesses can be confident that their decisions are based on valid and reliable data.
Plus, data cleaning helps eliminate any discrepancies in the data, ensuring that the data can be used without any issues. This can help businesses avoid costly errors and delays in their operations. Additionally, it helps businesses save time and money. Businesses can avoid potential legal issues that may arise from using inaccurate data.
Also, data cleaning helps improve customer satisfaction. By ensuring that their data is accurate and up-to-date, businesses can provide their customers with a better experience. Businesses can easily identify customer issues and address them quickly.
What Is The Goal Of Data Cleaning?
Data cleaning aims to improve the accuracy, integrity, and quality of data. This process involves identifying and correcting errors and inconsistencies in the data and removing any unnecessary or irrelevant data.
Also, data cleaning involves standardizing data formats, such as changing a date format from DD/MM/YYYY to YYYY-MM-DD. This process is essential for ensuring that the data is reliable and can be used for analysis and decision-making.
What Are The Benefits Of Data Cleaning?
Data cleaning is a beneficial process for enterprise businesses, as it helps to improve the accuracy, validity, and consistency of their data.
By taking the time to clean up their data, businesses can reap a variety of rewards, including:
- Improved Data Quality
Data cleaning helps reduce errors and increase data accuracy. It can also help identify any discrepancies or inconsistencies in the data, which can be addressed before further analysis begins.
- Increased Efficiency
By eliminating any errors or duplicates in the data, businesses can save time and resources that would otherwise be spent manually correcting mistakes. This helps to improve the efficiency of the data analysis process.
- Reduced Costs
Businesses can save money by avoiding costly mistakes and delays caused by errors in their data. Data cleaning also helps to streamline processes, resulting in fewer resources being used.
- Improved Decision-Making
Clean data helps to ensure that the decisions your business makes are based on reliable and accurate information. This helps to improve the overall quality of the decisions that your business makes.
If you are looking to take advantage of the benefits that data cleaning offers for enterprise businesses, Capella can help! We are a leading data service provider and can ensure your data is up-to-date, accurate, and reliable. Contact us today to learn more!
What Are The Steps Involved In Data Cleaning?
Data cleaning is an essential process for ensuring the accuracy, validity, and consistency of data. It involves checking data for any errors and correcting them if necessary. The steps involved in data cleaning vary depending on the data type and the project’s specific goals.
Identify Any Potential Issues With The Data
The first step in data cleaning is identifying any potential data issues. This includes identifying any missing values, incorrect data types, or outliers. It is important to identify any potential issues early on in the process to ensure that the data can be corrected before further analysis.
Perform Data Validation
The next step is to perform data validation. This involves checking the data for any errors or inconsistencies. This can be done manually or by using automated tools. Once any errors have been identified, they can be corrected.
Perform Data Transformation
The third step is to perform data transformation. This involves changing the format of the data to make it easier to work with. This could involve changing the data type, converting the data into a different format, or combining multiple data sources into one.
Perform Data Standardization
The fourth step is to perform data standardization. This involves ensuring that all data follows a consistent format. This can include changing the format of dates, ensuring that all numerical values are represented in the same way, and ensuring that all text is in the same format.
Double Check Data
Finally, the data can be checked for any remaining errors. This includes checking for any duplicates or inconsistencies in the data. Once all errors have been identified, they can be corrected.
Data cleaning is an essential process for ensuring the accuracy, validity, and consistency of data. By following the steps outlined above, businesses can ensure that their data is clean and ready for further analysis.
Components Of Quality Data
Data quality is essential for any enterprise business to make informed decisions. Quality data can be defined as accurate, consistent, and up-to-date data. Also, it can be described as data that has been checked for errors and is free of any inaccuracies.
When it comes to data quality, there are a few key components to consider. These components are accuracy, completeness, consistency, timeliness, and relevance.
Accuracy
Data accuracy refers to the degree to which data is free from errors. This means that the data must be free from typos, incorrect values, and other inaccuracies.
Completeness
This refers to the extent to which a dataset contains all of the necessary data needed for accurate analysis and decision-making. A complete dataset has no missing, incomplete, or blank values.
Consistency
This component of data quality refers to the degree that a dataset is consistent with other datasets within the same system. It must have a uniform format, as well as consistent data types and units of measure.
Timeliness
Timeliness refers to how often data is updated. This can be measured in terms of both frequency and latency (time lag). For example, if you are collecting data about customer purchases, you would want to update it as quickly as possible in order to make the most accurate decisions.
Relevance
Data relevance refers to the degree to which data is relevant to the task at hand. This means that the data must be relevant to the task and provide the necessary information.

What Is The Difference Between Data Cleaning And Data Transformation?
Data cleaning and data transformation are two different processes that are used to improve the quality of data.
Data cleaning is the process of detecting and correcting any errors in data. On the other hand, data transformation is changing the format or structure of data to make it more usable.
Data cleaning is an essential first step in any data analysis project. It involves identifying and correcting errors in the data, such as typos, incorrect values, and missing values. Additionally, it involves standardizing the data. For example, ensuring that all dates are in the same format and that all values are represented in the same units. This process is necessary to ensure data is accurate and reliable.
On the other hand, data transformation refers to making data more usable. This process involves changing the data format, such as converting text data into numerical data or converting data from one format to another. Data transformation also involves aggregating data, such as combining data from multiple sources into one table.
Data Cleaning Vs. Data Cleansing
Data cleaning and data cleansing are often used interchangeably, but they are actually two distinct processes.
Data cleaning is the process of identifying and correcting errors and inconsistencies in data. It involves checking the data for any errors (such as incorrect and missing values, incorrect formatting, etc.) and correcting them if necessary.
On the other hand, data cleansing refers to the process of transforming raw data into a more useful form. It involves cleaning up the data by removing duplicate records, standardizing the data format, and filling in missing values. Data cleansing is often used to prepare data for analysis or to improve the accuracy of predictive models.
Data cleaning and data cleansing are both important processes for ensuring your data's accuracy, validity, and consistency. Data cleaning is necessary for identifying and correcting errors in the data, while data cleansing is necessary for transforming raw data into a more useful form. However, both processes are essential for enterprise businesses that rely on data-driven decisions.
Improving Data-Driven Decisions With Data Cleaning
Data-driven decisions are becoming increasingly important for enterprise businesses. Companies need to make sure that their data is accurate, valid, and consistent in order to make the best decisions.
Data cleaning is essential for ensuring that the data used for decision-making is reliable and up-to-date. By identifying any inconsistencies or errors in the data, businesses can identify areas where they can improve their data collection and management processes. This can help to ensure that the data used for decision-making is more accurate and reliable.
Also, data cleaning helps businesses reduce the time it takes to make data-driven decisions. By ensuring that the data is accurate and valid, businesses can make decisions faster and more efficiently.

Final Thoughts
Data cleaning is a critical process for ensuring that the data used for decision-making is reliable and accurate. By identifying and correcting errors and inconsistencies in the data, businesses can reduce the time it takes to make data-driven decisions and improve their decision-making accuracy.
However, data cleaning takes time and expertise to ensure that the data is as accurate and valid as possible. Therefore, businesses should partner with a reliable data service provider like Capella to ensure their data is cleaned and transformed correctly.
From modern technology to an experienced team of data experts, Capella can help enterprise businesses clean and transform their data so they can make better decisions quickly and confidently.
So, don't wait any longer -- contact Capella today and let us help you get the most out of your data!
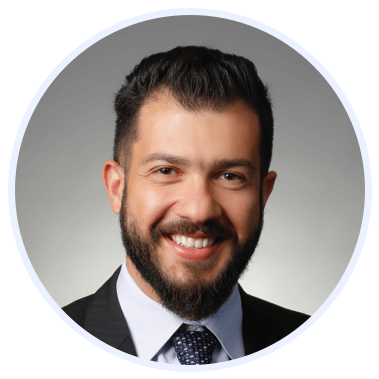
Rasheed Rabata
Is a solution and ROI-driven CTO, consultant, and system integrator with experience in deploying data integrations, Data Hubs, Master Data Management, Data Quality, and Data Warehousing solutions. He has a passion for solving complex data problems. His career experience showcases his drive to deliver software and timely solutions for business needs.