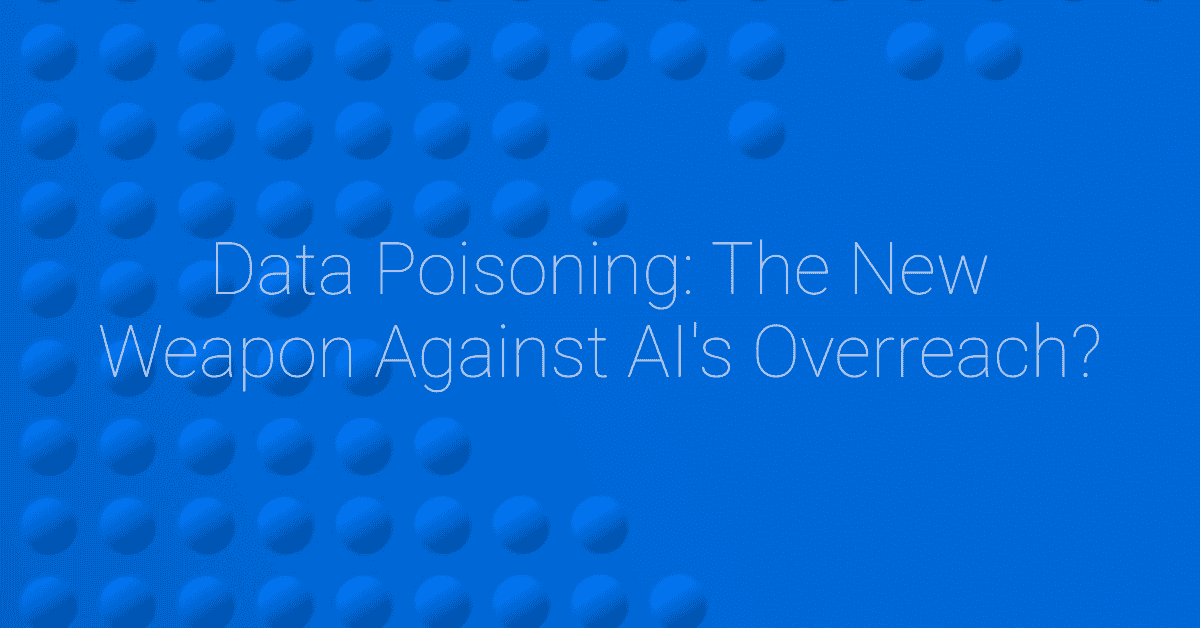
AI is advancing at an incredible pace. Yet with each stride it takes towards mimicking and even surpassing human intelligence, concerns around its potential overreach multiply. Generative AI models like DALL-E, Midjourney and Stable Diffusion have crossed new frontiers in creativity. But they’ve also raised alarms about copyright infringements, unauthorized use of data, and intellectual property violations.
Enter data poisoning - the new kid on the block promising to be a potent counterweight to AI's unfettered power. But how exactly does it work? And what are its wider ripple effects on technology, business, and society? Let's dive in.
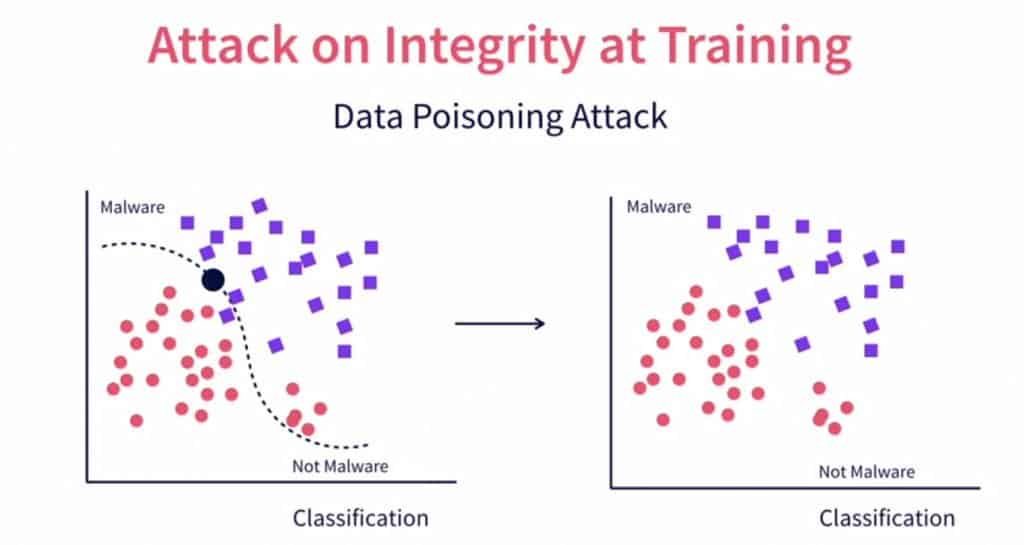
How Data Poisoning Throws AI Models Off Track
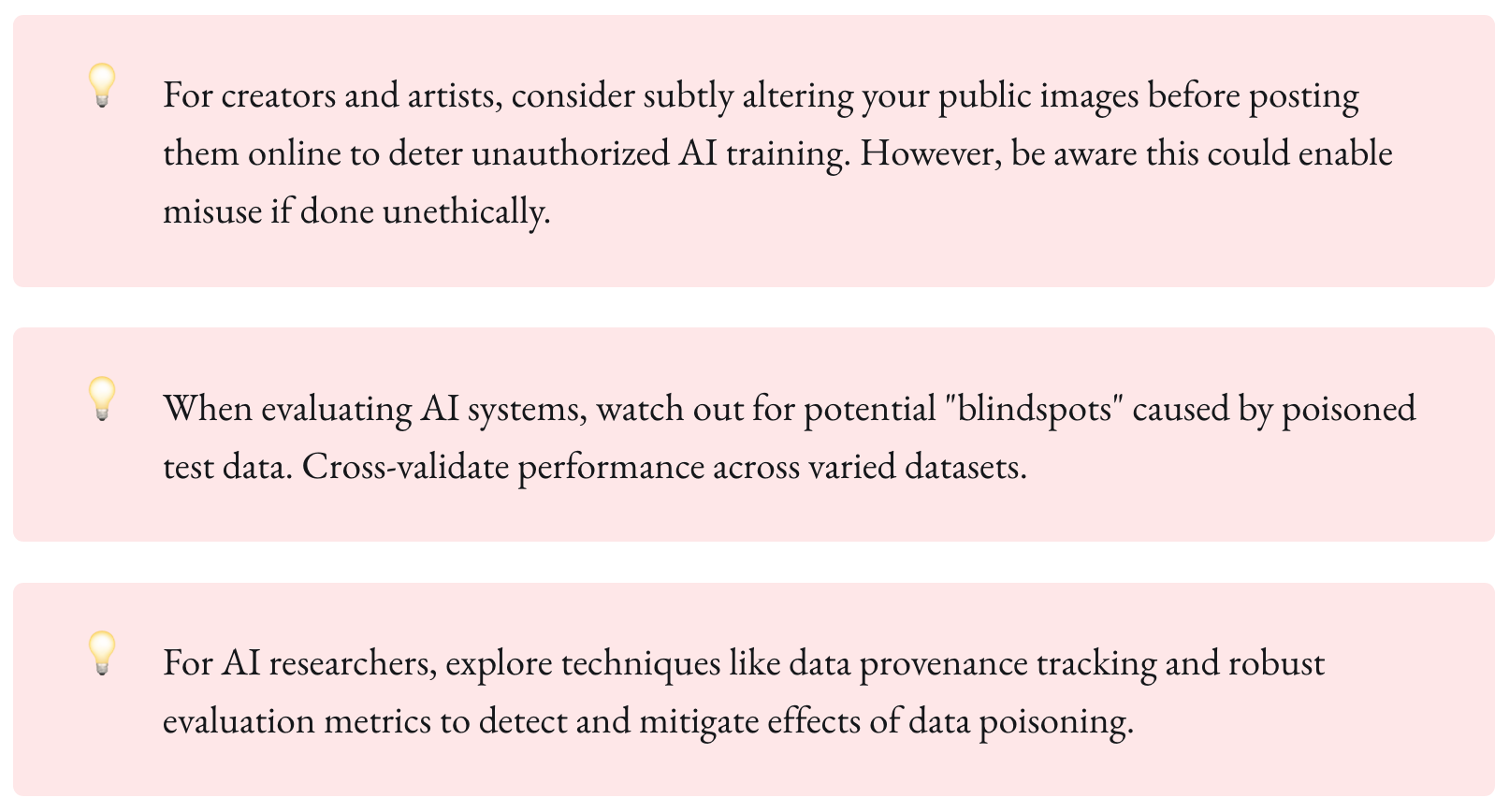
At its core, data poisoning relies on a simple insight - corrupt the data, and you distort the AI.

AI models like Midjourney are trained on massive datasets. They learn complex representations and patterns within this data, allowing them to generate new outputs like images and text. Data is their lifeline.
Poisoning this data involves subtly tweaking parts of it such that the AI gets confused. Pixel values in images could be altered ever so slightly. Imperceptible to the human eye, but enough to mislead the AI.
What's the impact? Say you fed Midjourney a beach photo altered via data poisoning. It might interpret a boat as a car, trees as buildings.
Subtle changes in the training data lead to categorically wrong outputs. The AI's perception is knocked off-kilter.
"Data poisoning attacks the integrity of the data pipeline that feeds machine learning models. Much like how food poisoning attacks the integrity of the human body."
Tools like Nightshade operationalize data poisoning specifically to trip up generative AI models. The motive? Protecting artists and creators from copyright theft.
By poisoning data from the internet that's being used to train these models, tools like Nightshade aim to distort the AI's outputs. If Midjourney can't accurately interpret an image, it can't repurpose and profit from it without permission.
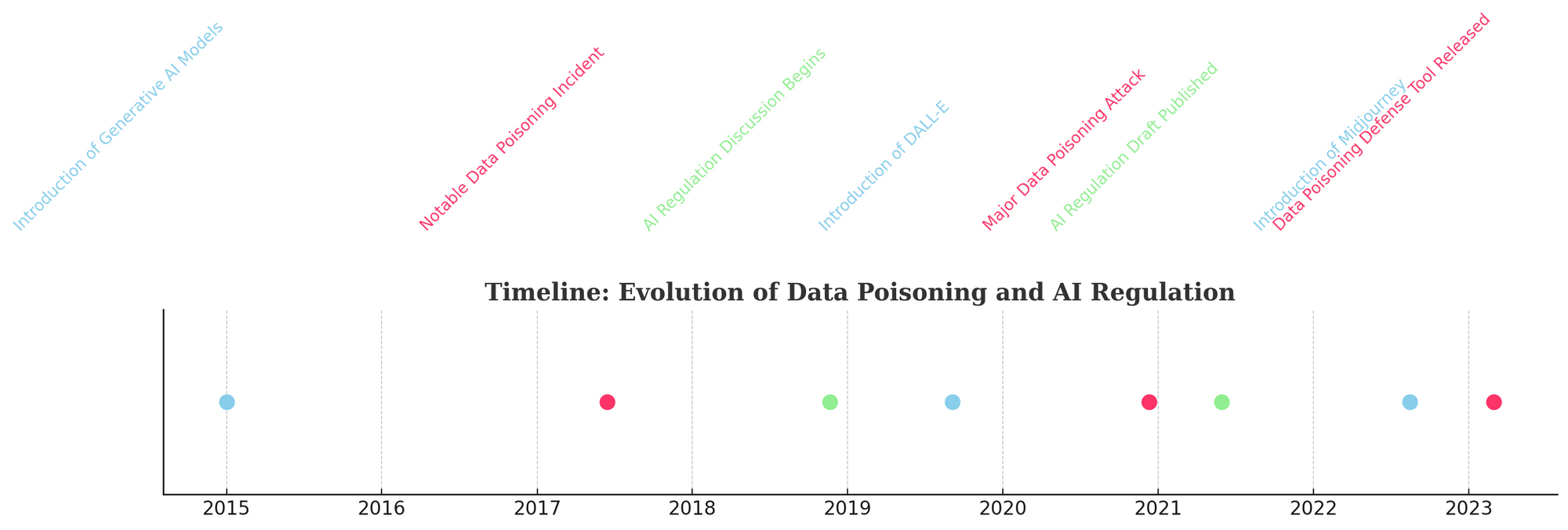
Why Data Poisoning Spooks Big Tech
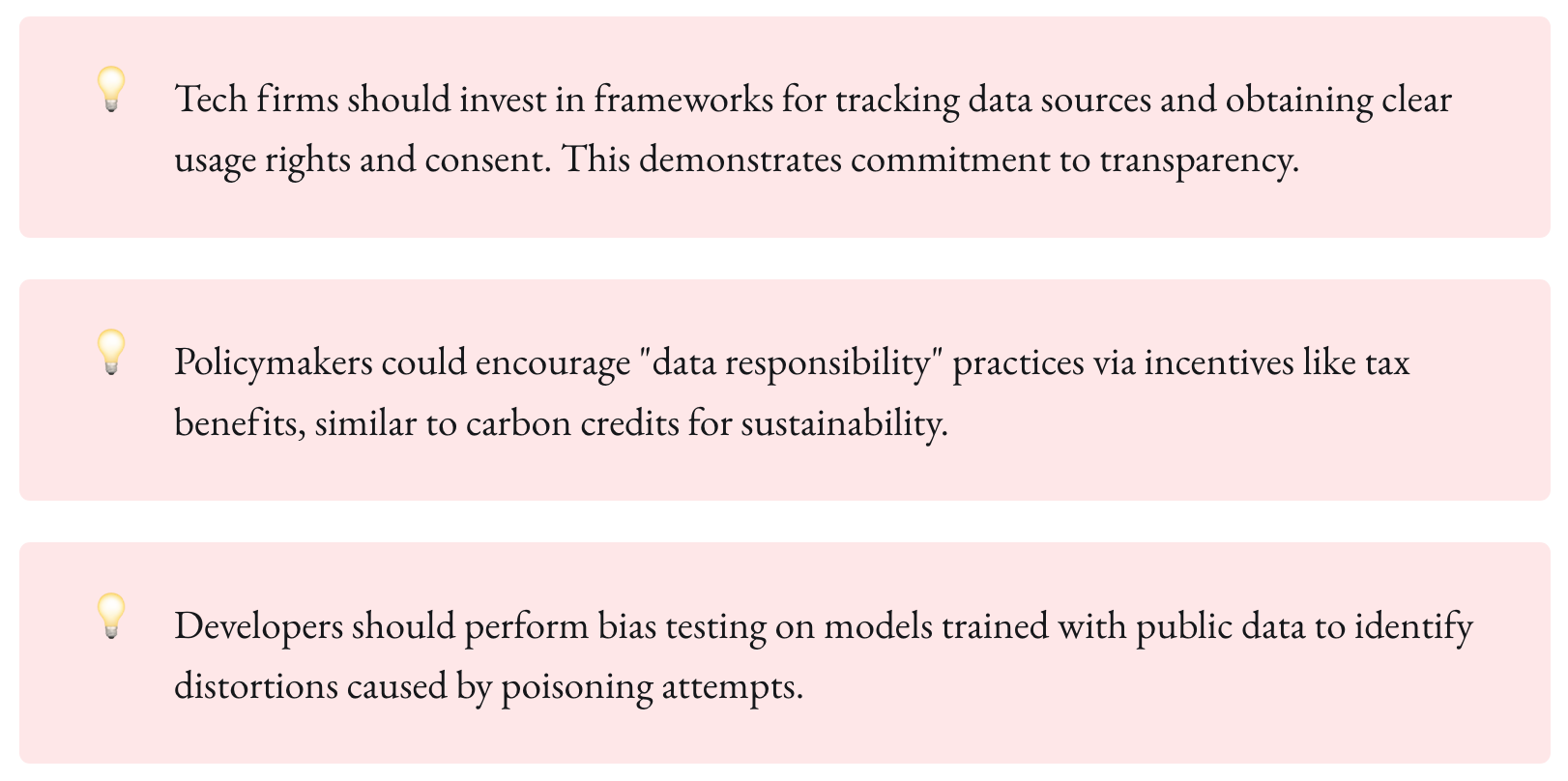
Generative AI has big tech rattled. Google, Microsoft, Meta - all racing to stay atop the AI game.
Their models need copious data to train on. Billions of images, videos, text excerpts from the internet - free for the taking[2].
Or is it? Cue data poisoning.
Big tech's data free-for-all is being challenged like never before. Legal boundaries around copyright and fair use are murky. Data poisoning adds kindling to the fire.
"Data poisoning means tech giants can't just scrape and monetize public data without repercussions. The scale required for training AI models means maintaining high data quality now seems almost impossible."
Not just training, even evaluating AI models gets tougher. How can you benchmark performance if some test data is poisoned in subtle ways? The trust in AI unravels.
Panic ensues. Microsoft caps image outputs by DALL-E at 50 per user, fearing copyright issues. Nvidia pauses public access to research models. Big tech is scrambling to avoid legal landmines.
The public perception of AI and big tech hangs in the balance. As data poisoning proliferates, transparency and rigor around sourcing data becomes imperative.

When Defense Morphs Into Offense

Data poisoning began as a defensive shield against AI overreach. But it's morphing into an offensive sword.
Adversaries now have a potent way to corrupt machine learning models. Poisoned data activated at the right time could wreak havoc - misclassifying objects for a self-driving car, garbling translations, botching facial recognition.
The stakes are high. As AI permeates healthcare, finance, transportation, data poisoning presents a looming threat. Its power to corrupt at scale cannot be ignored.
But the tech industry isn't defenseless either. An arms race around AI security has been brewing for years. Scrubbing poisoned data, securing models with blockchain, new frameworks to verify training sources - countermeasures are being developed.
Nonetheless, data poisoning remains a formidable danger. The window of opportunity for adversaries is only widening as models become more complex.

The Road Ahead: Fierce Debates, Tighter Regulation


Data poisoning brings some complex questions to the fore:
- Who really owns public data in the age of AI?
- What should the legal recourse be against malicious data poisoning?
- How can proper consent be ensured when training AI models?
- Can we develop provably robust models despite poisoned data?
Tricky tradeoffs abound. Consider watermarking images before releasing them publicly - helps creators track stolen IP, but also makes poisoning easier.
The legal side remains a big gray zone. Some compare data poisoning to illegally sabotaging products, while others see it as necessary civil disobedience against big tech's unchecked power. Tests in courtrooms worldwide seem inevitable.
Tighter regulation around data collection and usage seems likely. The EU has already pioneered strict data protection laws. As models become central to finance, healthcare and more, regulatory scrutiny will only grow.
But bans could be counterproductive. Instead, policymakers should aim to incentivize openness around training data, auditable AI, and inclusive public oversight. Proactive cooperation between tech firms and regulators is key.
"Data poisoning conjuresgrave dangers of misuse. But it also provides an opportunity to better align the development of AI with social interests."
The Clash of Tech's Titans
For too long, big tech has profited richly from largely ungoverned AI systems. Data poisoning finally heralds some reckoning.
Creators and activists are signaling that open season on the internet's data treasures is over. The legitimacy and ethics of these AI empires are under attack.
Can tech firms defend themselves while also addressing valid concerns about overreach? They now have a chance to transform creaky legal frameworks and usher in better data accountability.
But the window for self-regulation is closing. Governments will likely step in soon with strict rules. And they have public sentiment on their side - 78% of Americans already mistrust social media firms and their AI applications.
The stakes in this clash couldn't be higher. Beyond profits and fortunes, the very future of human-AI cooperation hangs in the balance. A healthy equilibrium between technological progress and social welfare is possible, but only through foresight and responsibility.
In substance and symbolism, data poisoning encapsulates the struggles in achieving this equilibrium. Its evolution will shape much more than just innovations in AI - it will mold the ethical fabric of the dawning age of artificial intelligence.
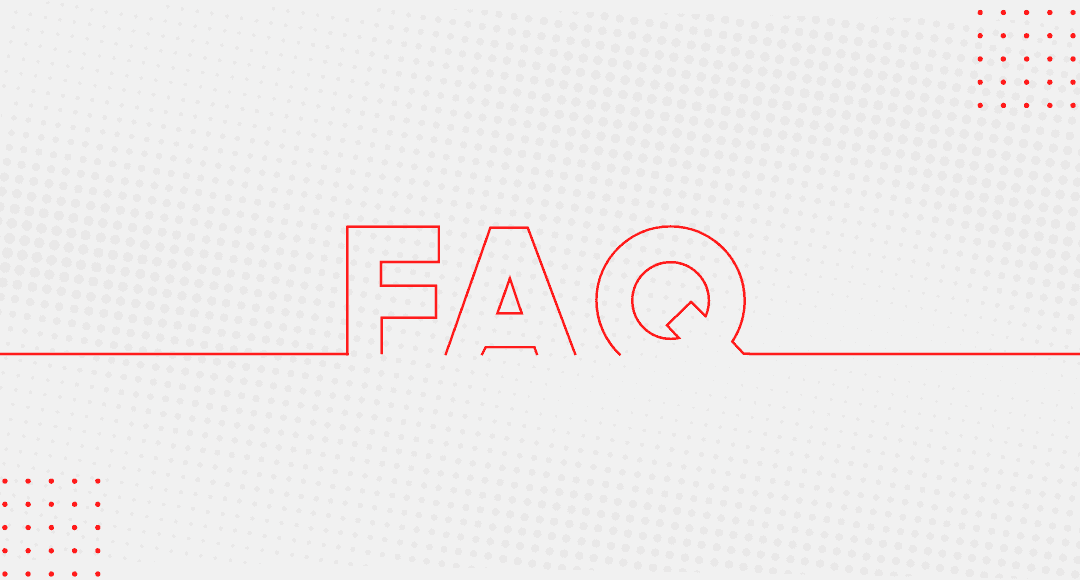
What exactly is data poisoning and how does it work?
Data poisoning refers to the intentional corruption of training data used by AI systems, through subtle alterations that end up distorting the AI's performance. For example, an image classification model could be fooled into mislabeling objects by tweaking a few pixels in its training images. These minor perturbations are imperceptible to humans but throw off the mathematical patterns that the AI relies on. By undermining the quality of the source data, data poisoning ends up severely compromising the AI system's ability to produce reliable outcomes.
What are some examples of where data poisoning has been used against AI?
Data poisoning attacks have primarily targeted computer vision AI so far. Artists and photographers have used techniques like Nightshade to alter their openly shared images - for instance, by overlaying a faint watermark or texture. This causes Generative AI models like Midjourney to mistake these images during training, hampering their ability to mimic the original art style or creation without permission. Researchers have also demonstrated poisoning attacks that could fool facial recognition and autonomous driving systems with potentially dangerous real world consequences.
Who is most concerned about data poisoning and why?
Major technology firms like Google, Meta, and Microsoft who rely extensively on large AI models trained on vast datasets scraped from the internet are most anxious about data poisoning. The indiscriminate gathering of images, text, and other data to feed their AI engines is being challenged. Poisoned data could act as a crippling force multiplier against these models. Startups exploring nascent AI capabilities also fear training their models on contaminated datasets that skew outcomes. However, not all views of data poisoning are negative - digital rights advocates see it as a form of protest against unfettered AI overreach.
What countermeasures are being developed against data poisoning?
While still in their early stages, some promising safeguards are emerging. These include improved data provenance techniques to audit sources and prevent poisoning, systems that distribute model training across nodes to avoid central failure points, expanded testing on diverse adversarial data, and blockchain-protected training pipelines. However, countering data poisoning at the scale required for industrial AI could prove exceedingly difficult. The cat-and-mouse game between AI offense and defense is clearly intensifying.
What are the ethical concerns surrounding the use of data poisoning?
Data poisoning does raise troubling questions. Its use to protect intellectual property or rein in harmful AI may be justified, but the same tools could also enable malicious actors to deliberately sabotage AI systems, with catastrophic consequences for safety-critical applications like self-driving vehicles or medical diagnosis. Gradual exposure of AI systems to controlled data poisoning attacks could potentially make them more robust. But mishandling this could seriously undermine public trust. Clear guidelines are needed on acceptable use and oversight of data poisoning.
How might data poisoning influence the policy landscape around AI development?
The growing prominence of data poisoning will likely accelerate policy discussions regarding ownership and usage terms for publicly available data in the age of pervasive AI. Laws will need to balance creators' interests and consent with norms around fair use and AI research access. Data responsibility and provenance standards for tech firms may be mandated as a requirement for operating in key markets. But policymakers will also need to tread carefully - overregulation risks stifling innovation while underregulation leaves societies vulnerable. Multistakeholder consultation and dynamic policies will be key to ensure balanced oversight.
Could data poisoning spark an "AI arms race" between tech firms?
Absolutely. With data being the fuel for AI advancement, whoever can amass the largest high-quality datasets gains an edge. Data poisoning threatens this competitive dynamic, forcing tech firms to double down on security and sophistication of training regimes. This could fuel an escalating arms race dynamic as firms try to one-up each other on robust data sourcing, verifiable training, and resilient models. Less scrupulous actors may even resort to actively poisoning competitors' datasets. Without cooperation on ethical norms and safety, this risks creating unstable dynamics in the AI field.
What societal impacts could widespread data poisoning have?
If left uncontrolled, the normalization of data poisoning could foster a climate of misinformation and mistrust that undercuts human-AI collaboration. Poisoning attacks on speech and language models could amplify harmful biases. HealthCare and financial AI could also be disrupted with dangerous fallouts. But prudent use of data poisoning may also lead to a culture of accountability, transparency and consent around AI training. Responsible disclosure norms must be encouraged. Overall, the societal impacts depend heavily on establishing ethical standards and multistakeholder oversight over this double-edged technology.
Is banning data poisoning a viable solution?
Outright bans on data poisoning are unlikely to succeed. The techniques leverage fundamental weaknesses in how AI models are trained - these cannot be regulated away. Bans will only discourage responsible disclosure and oversight. More nuanced policy and balanced incentives are needed. Accountability should be emphasized more than prohibitions, encouraging positive patterns of cooperation. Independent audits and red team testing of AI systems can also help immunize them against poisoning over time. Rather than bans, the priority should be enabling society to cautiously but effectively harness the power of data poisoning.
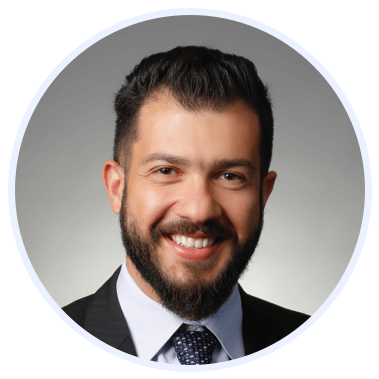
Rasheed Rabata
Is a solution and ROI-driven CTO, consultant, and system integrator with experience in deploying data integrations, Data Hubs, Master Data Management, Data Quality, and Data Warehousing solutions. He has a passion for solving complex data problems. His career experience showcases his drive to deliver software and timely solutions for business needs.