.jpg)
As businesses rely more and more on data-driven decisions, ensuring data quality becomes an essential task. Data quality management is the process of verifying data accuracy, completeness, and reliability.
Strategies, policies, and procedures are developed and implemented to achieve the highest quality standards. These ensure data meets the needs of all stakeholders and reduce the risk of errors. High-quality data serves as a foundation for informed decision-making and boosts business success. Therefore, businesses of all sizes need to prioritize Data Quality Management.
Don't wait any longer to elevate your Data Quality Management. Reach out to Capella and start transforming your business today!
What Is Data Quality?
Data quality guarantees that data is accurate, trustworthy, and pertinent to its intended use, which is necessary to make well-informed decisions. The ongoing data quality management process involves identifying and addressing issues impacting data quality, such as inaccuracies, duplication, outdated information, inconsistent formats, poor integration, lack of governance, hardware or software failures, and insufficient security.
To maintain data accuracy and reliability, organizations must continuously monitor and evaluate, set up metrics, conduct post-mortems, educate employees, establish consistent procedures, and perform audits.
By proactively implementing appropriate strategies and processes, organizations can maximize their data's value and utilize it effectively for decision-making.
.jpg)
Dimensions Of Data Quality
There are four elements to measure the quality of data:
- Accuracy: Closeness of data to real-world values. Enhance accuracy by validating data, verifying it against known values, and ensuring correct data entry.
- Completeness: Presence of all necessary data. Improve completeness by capturing all data and ensuring its accuracy.
- Consistency: Uniformity of data across different sources or systems. Boost consistency by establishing standards and processes for data entry and storage.
- Timeliness: Up-to-date status of data. Increase timeliness by regularly updating data and maintaining its currency.
Importance Of Data Quality
The significance of data quality is crucial for businesses to make informed decisions and maintain competitiveness. Data quality management ensures data accuracy, consistency, and reliability. Its benefits include:
- Improved efficiency: High-quality data streamlines processes and accelerates decision-making with accuracy, facilitating improvement identification and necessary changes.
- Increased customer satisfaction: Accurate data enables customers to make informed decisions, while poor data quality can result in dissatisfaction.
- Reduced costs: Accurate data saves money by avoiding costly errors and minimizing time spent on data cleansing, as poor data quality can lead to wasted resources and missed opportunities.
- Improved decision-making: High-quality data allows organizations to identify trends and patterns, leading to better decisions.
- Improved compliance: High-quality data assists organizations in adhering to regulations and industry standards, preventing costly fines and penalties due to non-compliance.
.jpg)
Common Sources Of Poor Data Quality
The common sources of poor data quality can be broken down into several categories.
Human Error
This is a prevalent cause of poor data quality, arising from inadequate training, misunderstandings, or data entry mistakes. These errors can lead to incorrect data entry, formatting, analysis, or duplication.
To mitigate these risks, implement clear processes, train staff on data entry and analysis, and establish a system for error detection and alerts, allowing for prompt identification and correction.
Incomplete Or Missing Data
This can result from human error, technical issues, data entry or processing errors, or data security breaches. Such data issues can hinder informed decision-making, lead to erroneous conclusions, and cause wasted resources.
To combat this, organizations should establish data quality metrics, conduct post-mortem reviews, educate employees on data entry and processing, implement consistent procedures, perform data quality audits, and ensure data security to prevent unauthorized access or manipulation.
Data Duplication
This arises when data is stored in multiple places, causing confusion and inconsistency. It can result from manual entry errors, data migration, integration issues, or incorrect data mapping and transformation.
Data duplication leads to data loss, inconsistency, and inaccurate reporting. Organizations should establish accurate and consistent data entry processes to prevent it and utilize data quality tools to detect and correct duplication issues.
Outdated Or Irrelevant Data
This can result from changes in data requirements or sources and data entry errors. Such data leads to inaccurate results and confusion during analysis.
To ensure data accuracy and reliability, organizations should regularly review and update their data, verify its accuracy and completeness, and implement automated processes for detecting outdated or irrelevant data.
Additionally, data quality checks should be carried out, and data quality management processes, such as data cleansing and validation, should be implemented. These measures will ensure that organizations maintain accurate, reliable, and up-to-date data.
Inconsistent Data Formats
These occur when data from different sources is stored in varying formats, complicating analysis and interpretation. This issue can lead to incorrect results and data integrity problems.
Organizations should standardize input, output, and storage data formats to address this, ensure consistent data transformations, and confirm compatibility with applications or platforms during import/export.
By establishing consistent data formats, organizations can maintain accurate, reliable data that is easily accessible and analyzed.
Poor Data Integration
This can result in inaccurate, incomplete, or inconsistent data. The problem can arise from a lack of understanding of data usage, incorrect mapping of data fields, or insufficient data standardization or governance.
Organizations should implement a comprehensive data integration strategy encompassing data mapping, standardization, and governance to guarantee high-quality data. Monitoring and auditing processes should also be included to maintain data accuracy and consistency.
Lack Of Data Governance
This involves creating and implementing policies, procedures, and standards to maintain data accuracy, consistency, and security. Without it, organizations risk relying on unreliable or outdated data and exposing sensitive information to unauthorized access.
A data governance team must be well-versed in data security, privacy laws, management, and analytics to address data quality issues and enforce responsible and ethical data use. Robust and effective data governance is essential for any data-driven organization.
Hardware Or Software Failures
These can negatively impact data quality by causing data loss, corruption, or inaccuracies. Organizations should implement robust backup and redundancy systems to mitigate these risks and regularly update and maintain their hardware and software for optimal performance.
Lack Of Data Security
Data security is crucial for data quality management. Organizations should implement security protocols like access controls, encryption, and data masking to prevent unauthorized access, theft, or manipulation.
Advanced technologies like biometrics, artificial intelligence, and blockchain can enhance protection and add extra security layers.
Top 5 Best Practices For Data Quality
1. Set Up Metrics
This is the initial step in ensuring high-quality data. Metrics help track, measure, and monitor data quality, assessing accuracy, completeness, consistency, and timeliness, while detecting discrepancies.
Establishing metrics involves defining data quality goals, objectives, and standards, ensuring data meets organizational needs. Regularly review metrics to maintain relevance and monitor data quality to guarantee it meets the required standards. Customize data quality metrics to align with the organization's specific needs and goals.
2. Conduct Post-Mortems On Data Quality
Conducting post-mortems on data quality is crucial for maintaining high-quality data. These analyses identify the root cause of past data quality issues and prevent their recurrence by addressing underlying problems, such as poor data governance, inconsistent procedures, or inadequate training.
When conducting a post-mortem, gather data about the issue, related systems, processes, and people involved, and review pre-existing data quality metrics to identify gaps or weaknesses in controls.
Develop a plan to prevent similar issues, including updating metrics, implementing new controls, or providing additional training. Address the root cause and document any changes to data quality processes.
3. Educate Employees
Educating employees on data quality management is vital for maintaining accurate and reliable data. Training should cover data entry best practices, validation techniques, and proper handling procedures.
Employees must understand data quality's importance and its impact on organizational success, including potential consequences like inaccurate reporting, wasted resources, and decreased customer satisfaction. Educating employees ensures optimal data quality and its full utilization.
4. Establish Consistent Procedures
This is important for data quality, involving standardization of data collection, entry, storage, labeling, organization, and formatting.
Validating and verifying data accuracy also helps reduce errors and improve data integrity, providing reliable results. Having documented processes for data handling ensures consistency and swift identification and correction of issues. Implementing a system to monitor data quality and address concerns guarantees the highest data quality.
5. Perform Data Quality Audits
This is crucial for maintaining high-quality data. These audits systematically review and evaluate data, identifying errors, inconsistencies, or gaps in data quality, and assess accuracy, completeness, integrity, and reliability.
Data quality audits aim to ensure data is suitable for decision-making, helping organizations identify and address issues, improve data quality, and optimize data usage effectively.
When performing a data quality audit, organizations should consider the following steps:
1. Establish the scope of the audit: This should be clearly defined and should include the objectives of the audit, the data sources to be audited, and the types of data to be assessed.
2. Collect and analyze data: This is an important step in the data quality audit process. It involves gathering data from various sources and analyzing it to identify any errors, inconsistencies, or gaps in data quality.
3. Identify and document issues: Any issue must be identified and documented to track and address them.
4. Develop an action plan: An action plan should be developed to address any issue. This plan should include a timeline for addressing the issues, and any resources or tools that may be needed.
5. Monitor and evaluate results: Once an action plan has been implemented, organizations should monitor and evaluate the audit results to ensure that the desired outcomes have been achieved.
Performing regular audits can result in accurate, complete, and reliable data. This will help organizations make better decisions and maximize the value of their data.
Where Can Data Quality Concerns Be Addressed?
Addressing data quality issues depends on the problem and the organization's data architecture. Generally, concerns can be tackled in the source system, during the extract, transfer, and load (ETL) process, or at the meta-data layer.
Ensuring data quality in the source system is most effective and involves making changes for accurate and consistent data entry. Fixing issues during the ETL process requires adjusting the process for consistent and accurate extraction, transformation, and loading.
Lastly, addressing concerns at the meta-data layer involves updating data definitions, data types, and data mappings for consistency across sources, ensuring correct mapping in the target system.
Ready to transform your data quality management? Reach out to our team now! Visit our page and let us guide you on maximizing your organization's data potential. Don't wait – contact us today!
1. Address The Issue In The Source System
Addressing data quality issues in the source system is important. It involves identifying and correcting problems before they propagate downstream. This can include manual error correction, data updates, or implementing automated processes to ensure accuracy and integrity.
The goal is to maintain up-to-date and accurate data in the source system, stored in the correct format for effective downstream use. Proper data backup and security measures in the source system also reduce the risk of data loss or corruption.
2. Fix The Issue During The ETL Process
The Extract, Transform, Load (ETL) process is vital for data quality management. It involves extracting data from multiple sources, transforming it into a consistent format, and loading it into a target database.
High-quality data is essential before loading. Defining and applying data quality rules and standards during extraction, transformation, and loading ensures data quality. Automated tools and manual checks can monitor the ETL process to detect errors and inconsistencies.
Addressing data quality issues during the ETL process helps maintain high-quality data within organizations.
3. Fix The Issue At The Meta-Data Layer
This is crucial for data accuracy and reliability. Meta-data includes information about data structure, format, and source. Inspecting meta-data allows for identifying and correcting errors or inconsistencies by creating rules and checks for accurate data representation.
These techniques ensure data consistency across sources and prevent potential problems.
Cloud And The Future Of Data Quality
Cloud computing has transformed how businesses store, manage, and analyze data by offering scalability, flexibility, and cost-effectiveness. It allows organizations to deploy tailored applications and services, maintaining reliable, up-to-date data.
With access to powerful analytics tools, businesses can identify and address data quality issues, improving accuracy and relevance. Cloud computing ensures data security and enables easy storage and processing capabilities scaling as data needs grow, maximizing data value and usage potential.
Final Thoughts
Data quality is crucial for organizational success, and a comprehensive management plan is necessary to ensure accuracy, reliability, and timeliness. Best practices include setting up metrics, conducting post-mortems, employee education, establishing consistent procedures, and performing audits.
Addressing data quality concerns can occur in the source system, during the ETL process, or at the meta-data layer. Following these practices helps organizations maintain high-quality data, maximizing its potential as technology evolves.
Don't miss the opportunity to enhance your data quality management! Visit our contact page and get in touch with our experts today. Let us help you unlock your organization's full potential with high-quality data.
Want to learn more about data quality? You can look at these blogs to gain more knowledge:
- 10 Tips from Experts On How To Improve Data Quality
- Data Management Tools Roundup: Comparing the Market Leaders
- The Data Management Tools Spectrum: Finding Your Perfect Fit
FAQs
What is the main goal of data quality?
The main goal of data quality is to ensure that data is accurate, consistent, and complete. It is important to have high-quality data in order to make informed decisions and ensure that data is reliable and trustworthy. Data quality management involves processes and procedures designed to ensure that data is accurate, consistent, and complete.
What is an example of data quality control?
Data quality control involves a set of processes and procedures designed to ensure data is accurate, consistent, and complete. Examples of data quality control include validation, cleansing, auditing, and profiling.
Data validation is the process of ensuring that data is valid and meets certain criteria.
Data cleansing is the process of removing or correcting inaccurate or incomplete data.
Data auditing is the process of examining data to ensure accuracy and completeness.
Data profiling is gathering information about the structure and content of data.
What are the responsibilities of a data quality manager?
The responsibilities of a data quality manager include developing and maintaining data quality policies and procedures, monitoring data quality, and ensuring compliance with data quality standards.
They are also responsible for identifying and addressing data quality issues, developing and implementing data quality improvement initiatives, and providing training and guidance on data quality best practices.
What are the 4 pillars of data quality?
The four pillars of data quality are accuracy, completeness, consistency, and timeliness.
Accuracy refers to data that is accurate and free from errors.
Completeness refers to data that is complete and contains all the necessary information.
Consistency refers to data that is consistent across different sources.
Timeliness refers to data that is up to date and reflects the most recent information.
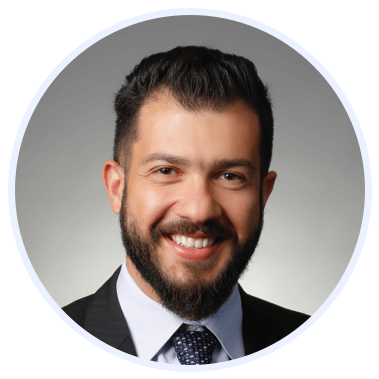
Rasheed Rabata
Is a solution and ROI-driven CTO, consultant, and system integrator with experience in deploying data integrations, Data Hubs, Master Data Management, Data Quality, and Data Warehousing solutions. He has a passion for solving complex data problems. His career experience showcases his drive to deliver software and timely solutions for business needs.