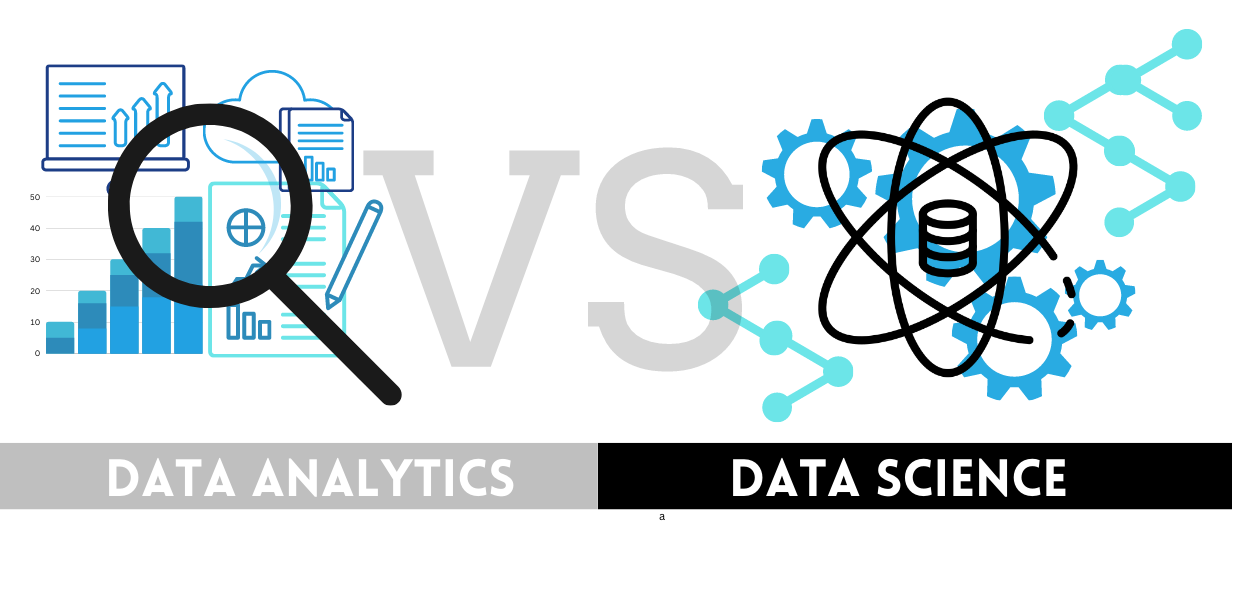
No one can say that we’re lacking in data, especially as industries look to advance their practices and refine their data collection methods. It’s even estimated that more than 90% of our total data as humans has been created in the last two years alone. An astronomical figure to consider, but this growing attention toward understanding data and the patterns that follow has all but entranced us all. But how are we to understand what data reveals if we don’t first understand the limitless potentialities of data science and then data analytics?
For example, scientists have begun using data science and computational biology to better understand how organelles in our cells supply energy to our bodies. These scientists decided to use methods from data science and statistics to identify patterns. They were able to find links between organelles to then create predictive patterns for use in subsequent research.
While this may seem heavy in biological practices, it does suggest that the power of data science and analytics is only expanding in more than corporate settings. This only furthers our understanding of how data can be used to solve problems that were previously mysteries without the use of predictive patterns and set methodology.
Capella Covers: Data Science vs. Data Analytics
- What is Data Science?
- What is Data Analytics?
- Similarities and Differences
- What should I choose?
What Is Data Science?
Data science is a field of study that combines multiple disciplines to gather data and create insights from that information. The entire discipline was first called data analysis in 1962 by John Tukey, but in the following years it became what we know today as data science.
Because it is considered an interdisciplinary field, data science often uses the same tenets of statistics, computer science, and predictive analytics among other fields. Data scientists focus on the study of information and often use predictive methods to extrapolate data for subsequent analysis down the road.

What Is Data Analytics?
Where data science focuses on predicting and capturing data, data analytics examine large data sets to then develop trends. Data analytics is also considered to be a branch of data science. Data science is the larger umbrella that data analytics then falls under. Think of data analytics as the analysis period of the data gathering process. There are generally considered to be four types of analytics including:
- Descriptive Analytics - Examines, describes, and understands what has already happened.
- Diagnostic Analytics - Looks to understand why something happened in the descriptive analysis period.
- Predictive Analytics - Uses past trends and data to predict future data patterns.
- Prescriptive Analytics - Identifies specific actions that a company should take after receiving certain data.
You can see that these types of analytics are based on an order of action. A descriptive analysis is the first, most basic understanding of what happened which then leads to a diagnostic analysis to understand why something happened. Then, the predictive analysis serves to formulate a plan for use that the prescriptive analysis refines for action that a company must take as a result of the total data.

Data Science Vs. Data Analytics
Think of data science as being related to predictive approaches, whereas data analytics is related to trends and forecasting. The data scientist works largely with algorithms, modeling, and ultimately based around critical thinking. They must take data from its most unrefined position to then clean it up for future use.
Once the data is better presented and organized, the data analyst can make insightful decisions and forecast potential outcomes for their client or business to use. It’s a seemingly clear hand-off between the two disciplines, but there are still overlaps and fundamental differences to consider as mentioned below.
Similarities: Data Science And Data Analytics
Both fields are similar for a few reasons. Namely, they are both highly sought after career choices through STEM industries. Their similarities often deal with:
- Backgrounds in Statistics, Programming, and Analytics - Both fields use a great deal of programs and rely heavily on an understanding of statistics. However, both must also have an analytical background to be able to take a methodical approach to whatever they are researching.
- Deal with Large Data Sets - Both fields deal with large sets of data, just in ways. A data scientist must be able to take data and create predictive models. A data analyst must be able to take even larger data sets to spot trends and then inform businesses on proper strategies to follow.
- Critical Thinking and Problem Solving Skills - Both disciplines require a high level of critical thinking to examine data.

Differences: Data Science And Data Analytics
Where the differences between data science and data analytics lie occurs in how they function from the start. Their main differences are:
- Initial Approach - Data scientists are always looking for the questions to ask while data analysts receive questions and then provide answers. Think of data science as the first step and data analytics as the second step. One creates the question to ask and the other examines the data to provide an answer.
- Roles and Responsibilities - A data scientist is the predictor, looking to identify problems to then hand off to the data analyst to solve the problem and find trends. One is responsible for spotting/predicting problems and the other is responsible for solving problems and finding trends. They work hand in hand, but they differ in what must be done to come to the conclusion.
- Technical vs. Business-Driven - As mentioned above, data scientists often have more technical background knowledge than data analysts. However, data analysts must have a better understanding of business practices in order to implement appropriate strategies.
Should I Be A Data Analyst Or Data Scientist?
Your decision should be based on your background knowledge. If you are better with math and statistics then data science could be the better route. However, if you have a higher business acumen and like disseminating information, then data analytics could be the better choice there.
While they are both principled in the same fashion, there are key differences in the roles that you could consider. Data scientists often deal with machine learning tools/algorithms and have to keep the potential questions top of mind. They are not necessarily finding the answers first, but they are asking the right questions for the data analysts to then answer. If you find yourself inquisitive about the process of dealing with data and like to write code, this is probably the best avenue.
Data analysts main goal is to interpret data and identify patterns as a result. Companies rely largely on analysts to use data visualization tools and analytical tools to find the answers to the questions that data scientists are asking.
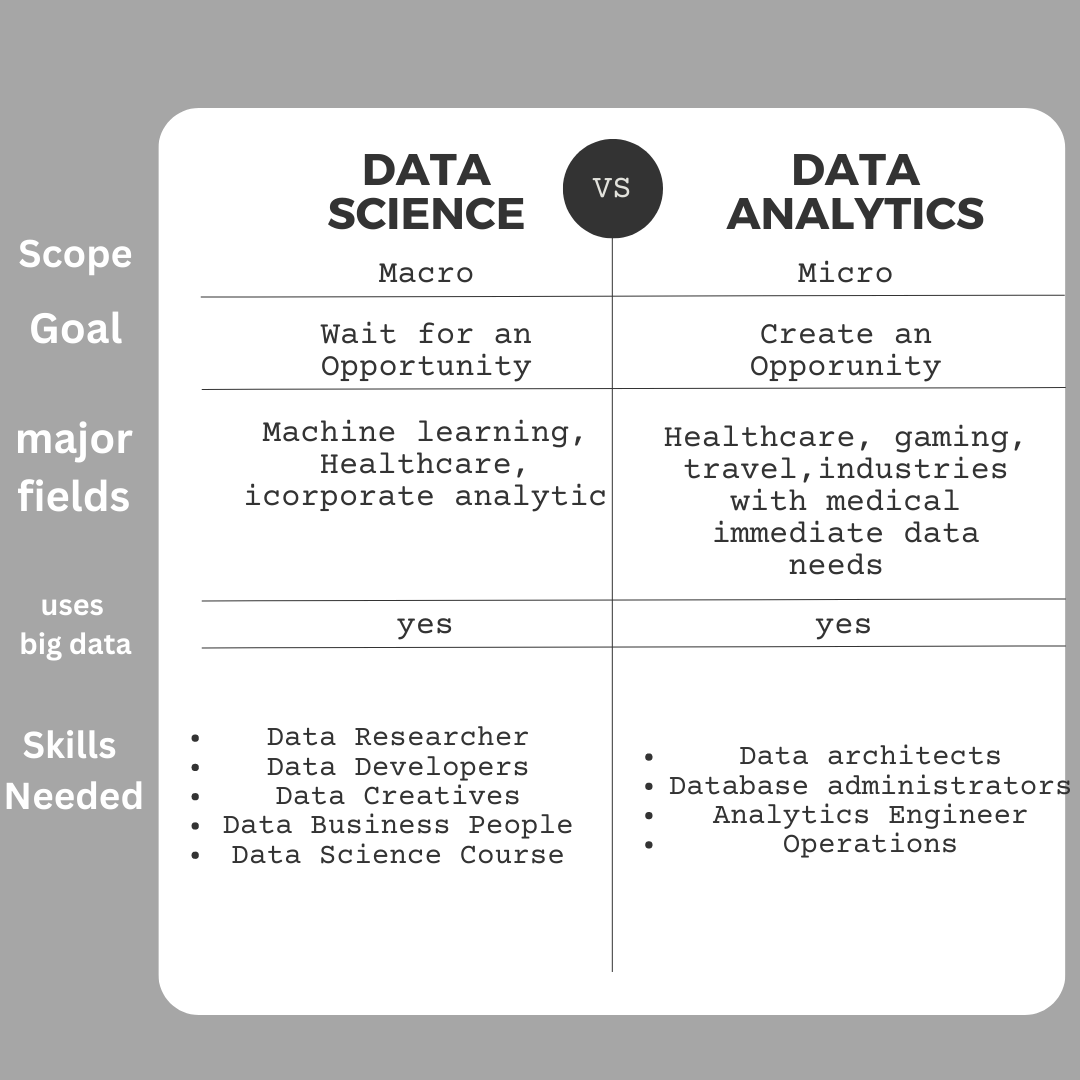
Is One Harder Than The Other?
It’s difficult to say if one is harder than the other, mainly because your assumption is based on skill set. It’s recommended that both have a background in Computer Science, Engineering, IT, Mathematics, and/or Statistics.
Data science may be seen as more technical given the need for additional math and programming skills. However, data analysts often thrive when they have a background in statistics. It all depends on the industry, company, and your specific role.
Which Pays More Data Science Or Data Analytics?
Because of the technical nature, data science generally pays a bit more than data analytics. There are largely more entry-level positions for data analysts because data scientists often require additional training and experience with mathematics.
As of April 2022, in the U.S. a data scientist earns an average salary of $122,499. A data analyst can look at an average salary of $71,958. Much of this depends on the industry, job type, and individual experience.
In terms of education, it’s generally recommended that both data scientists and data analysts have at least a bachelor’s degree. There are also opportunities to take online courses to learn skills associated with programming, data visualization, machine learning, large data frameworks, and even communication.
Other Key Considerations Of Data Science And Data Analytics
Data drives countless industries from operations to strategies and even in diagnoses. If you decide to pursue data science or data analytics you can expect to find a position in more than tech. For example, manufacturers use data science to help understand supply chains and distribution efforts. Businesses use data analytics and data science to formulate strategies based on customer buying behavior and industry trends among much else.
A large amount of medical research is now reliant on the methodology used in data science practices. Researchers and doctors can use data to better understand conditions and then treatment according to specific data sets. Similarly, government agencies use data science along with sports as well. Ultimately, data science and analytics is driving our growth into better serving the public.
Now, the next big push revolves around artificial intelligence (AI) and how data science can aid us to better understand how it works. Data scientists used AI as a tool to perform analysis as it builds models to better understand how human’s work and understand things. These efforts can help in healthcare settings by using historical data to predict potential outcomes. They can also help advance the gaming industry, online shopping, and customer service over time.
If you’re looking to become a data scientist or a data analyst, you should begin by researching specific industries to see where there is a need. If you’re interested in a specific field, consider the requirements needed and brush up on skills.
Final Thoughts: Capella Can Help You
If your business is looking to transform data services, Capella can help. With our countless case studies from oil and gas to construction, telecom, and even medical clients - we have the edge you’re looking for no matter the industry.
Check out our work today and let’s discuss why Capella’s low-code platform can work to build enterprise quality software for your business, without reinventing the wheel. Our Low-code Automation Platform works to build apps 10x faster all while reducing maintenance costs by 50% compared to traditional development.
Book a discovery call with us and we’ll explain the Capella Guarantee to deliver your first project in under a quarter. Let’s start by figuring out what is right for you.
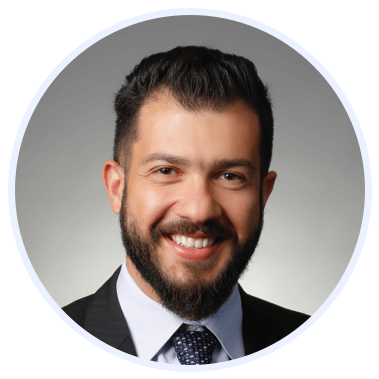
Rasheed Rabata
Is a solution and ROI-driven CTO, consultant, and system integrator with experience in deploying data integrations, Data Hubs, Master Data Management, Data Quality, and Data Warehousing solutions. He has a passion for solving complex data problems. His career experience showcases his drive to deliver software and timely solutions for business needs.