
Welcome to the exciting world of embedded analytics – a solution that has the potential to revolutionize the way you work with data and transform your business processes. By seamlessly integrating analytics capabilities into your existing applications and workflows, you can access real-time insights, make informed decisions, and stay ahead of the competition in today's fast-paced, data-driven environment.
At Capella, we specialize in helping businesses like yours make the most of their data. Our highly experienced talent pool and cutting-edge development expertise make us the perfect partner to help you harness the power of embedded analytics and streamline your workflows. In this guide, we will explore the benefits of embedded analytics, share practical tips on implementation, and offer best practices to ensure success.

1. Embedded Analytics:
1.1. The Rise of Data-Driven Decision-Making
In today's rapidly evolving business landscape, data-driven decision-making has emerged as a crucial factor in gaining a competitive edge. Organizations are now leveraging vast amounts of data to make informed decisions, optimize processes, and enhance customer experiences. The shift towards data-centric approaches has led to the need for tools and solutions that can effectively manage, analyze, and utilize this data.
1.2. The Need for Embedded Analytics
Embedded analytics is a powerful tool that allows businesses to harness the full potential of their data by seamlessly integrating data-driven insights into existing workflows and applications. By doing so, organizations can make data-driven insights more accessible to decision-makers, enhancing the overall decision-making process. As the demand for data-driven insights grows, the importance of integrating embedded analytics into workflows and applications becomes paramount.

2. Embedded Analytics: Core Concepts and Terminology
2.1. Defining Embedded Analytics
Embedded analytics refers to the integration of data analysis and visualization capabilities directly within business applications, processes, and workflows. This approach enables users to access relevant insights without leaving their primary application or workflow, thus improving efficiency and productivity.
2.2. The Components of Embedded Analytics
Embedded analytics can be broken down into several components, including:
- Data Integration: The process of collecting, cleaning, and preparing data from various sources for analysis.
- Data Analysis: The application of statistical and analytical techniques to extract meaningful insights from the data.
- Data Visualization: The presentation of data in graphical or visual formats to facilitate understanding and interpretation.
- Interactive Dashboards: Customizable, interactive interfaces that display key metrics and insights, enabling users to explore and interact with the data.
2.3. The Difference between Embedded Analytics and Traditional BI
Traditional Business Intelligence (BI) solutions typically involve standalone tools and platforms that are separate from an organization's core applications and workflows. Users must switch between these BI tools and their primary applications, which can disrupt workflows and hinder productivity. In contrast, embedded analytics integrates data-driven insights directly within applications and workflows, streamlining the decision-making process and minimizing disruptions.
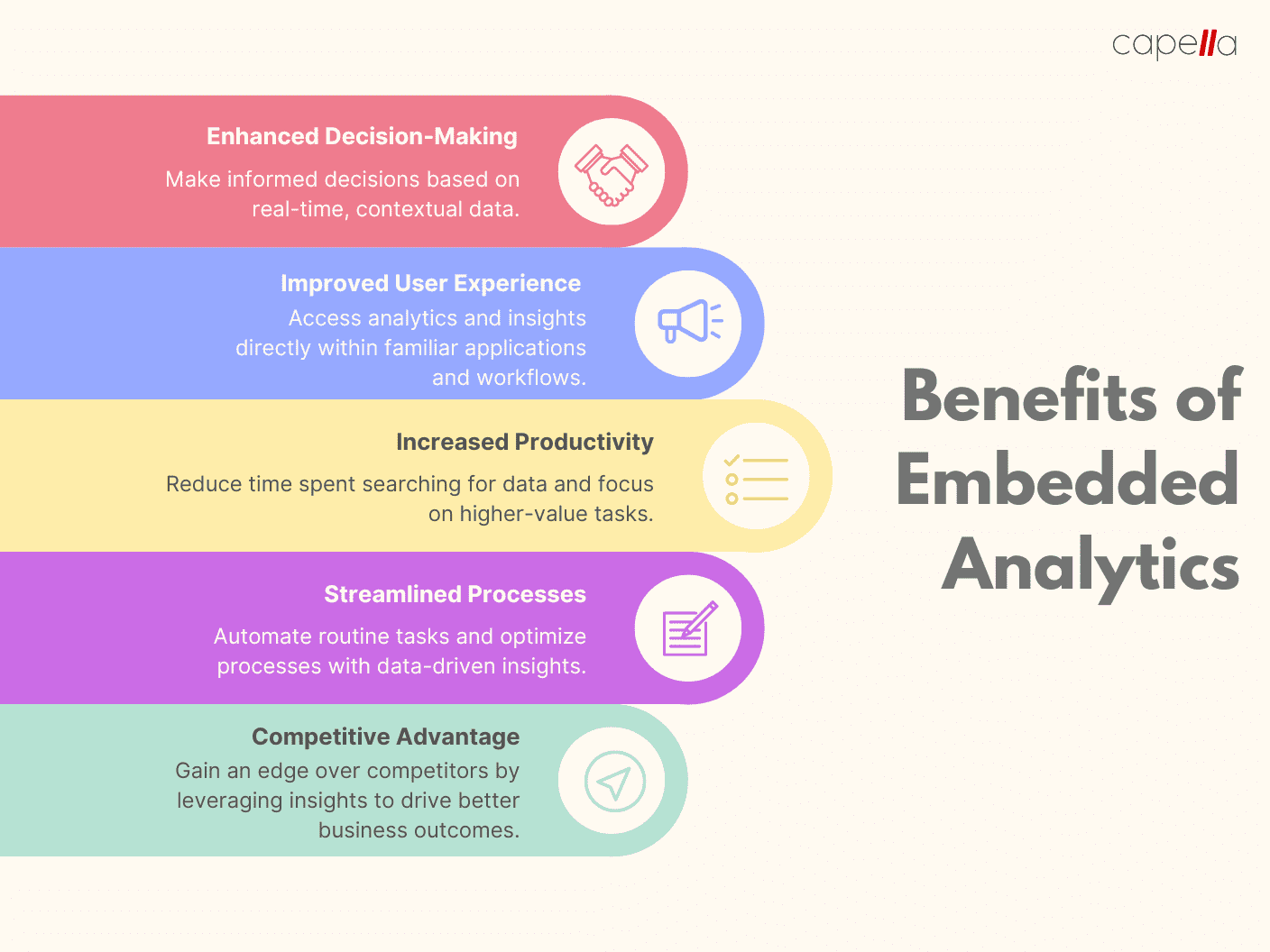
3. The Benefits of Embedded Analytics
3.1. Improved Decision-Making
By providing real-time, context-specific insights directly within applications and workflows, embedded analytics empowers users to make informed decisions quickly and confidently. This leads to better outcomes and a more agile organization.
3.2. Enhanced User Experience
Embedded analytics eliminates the need for users to switch between applications or navigate complex BI platforms, creating a more seamless and efficient user experience. This can also lead to higher user adoption rates, as employees are more likely to engage with a tool that is integrated into their existing workflows.
3.3. Increased Productivity
The integration of data-driven insights into applications and workflows allows users to access relevant information without leaving their primary tasks. This reduces the time spent on searching for data, resulting in increased productivity and allowing employees to focus on higher-value tasks.
3.4. Streamlined Processes
Embedded analytics can help automate routine tasks and processes by providing actionable insights directly within the context of the task. This streamlining can lead to improved efficiency, reduced errors, and faster response times, ultimately enabling organizations to be more agile and adaptive.
3.5. Competitive Advantage
Organizations that effectively implement embedded analytics can gain a competitive advantage by making better decisions, optimizing processes, and providing superior customer experiences. By leveraging data-driven insights, businesses can identify new opportunities, uncover hidden risks, and make strategic adjustments to stay ahead of the competition.

4. Evaluating Embedded Analytics Solutions
4.1. Identifying Organizational Needs and Goals
Before evaluating and selecting an embedded analytics solution, organizations must first identify their specific needs and goals. This may include understanding the types of data they need to analyze, the desired level of customization and flexibility, and the key performance indicators (KPIs) they wish to track. Establishing these requirements upfront will help narrow down the search and ensure that the chosen solution aligns with the organization's objectives.
4.2. Assessing Data Infrastructure
Organizations must also assess their existing data infrastructure to determine its readiness for embedded analytics. This involves evaluating the data sources, storage systems, and data processing capabilities to ensure they can support the desired analytics functionality. If necessary, organizations may need to invest in upgrading their data infrastructure to accommodate embedded analytics.
4.3. Evaluating Vendors and Technologies
There are numerous embedded analytics vendors and technologies available in the market, each with its own strengths and weaknesses. Organizations should consider factors such as ease of integration, scalability, customization options, and the vendor's track record in the industry. Additionally, it's essential to assess the vendor's commitment to security and compliance, as well as the quality of their support and training resources.
4.4. Total Cost of Ownership
When evaluating embedded analytics solutions, organizations should consider the total cost of ownership (TCO). This includes not only the initial implementation costs but also ongoing maintenance and support fees, potential infrastructure upgrades, and any required training for staff members. A comprehensive TCO analysis will help organizations make an informed decision by weighing the long-term value and ROI of the embedded analytics solution against its costs.
4.5. Security and Compliance
Data security and compliance are critical concerns for any organization, particularly when implementing embedded analytics. Organizations must ensure that the chosen solution meets industry-specific security standards, complies with relevant regulations (such as GDPR, HIPAA, or CCPA), and provides features to safeguard sensitive data. It's essential to work closely with the vendor to understand their security and compliance policies and ensure they align with the organization's requirements.

5. Best Practices for Implementing Embedded Analytics
5.1. Establish Clear Objectives and KPIs
Setting clear objectives and defining KPIs from the outset is crucial for a successful embedded analytics implementation. By identifying the specific goals they wish to achieve, organizations can better focus their efforts and measure the impact of the solution on their business processes.
5.2. Engage Stakeholders and Encourage Collaboration
Successful implementation of embedded analytics requires collaboration between different teams, such as IT, data analysts, and end-users. Involving stakeholders from the beginning ensures that their needs and perspectives are considered, leading to a more effective solution. Regular communication and feedback loops can also help address potential challenges and identify opportunities for improvement throughout the implementation process.
5.3. Prioritize Data Quality and Integrity
The effectiveness of embedded analytics is highly dependent on the quality and integrity of the underlying data. Organizations should establish processes to ensure data accuracy, consistency, and completeness. This may involve implementing data validation checks, standardizing data formats, and creating a data governance framework to manage data quality throughout the organization.
5.4. Provide Training and Support
End-users may require training and support to effectively use the embedded analytics tools and interpret the insights they generate. Organizations should invest in comprehensive training programs that cater to different user roles and skill levels. Providing ongoing support, such as helpdesk services or online resources, can also help users overcome challenges and maximize the value of the embedded analytics solution.
5.5. Iterate and Optimize
Embedded analytics implementations should be seen as an ongoing process rather than a one-time project. Organizations should continually evaluate the solution's performance, gather user feedback, and refine their approach to optimize the system's effectiveness. Regular updates and adjustments will help ensure that the embedded analytics solution remains aligned with the organization's evolving needs and goals.
6. Case Studies: Successful Embedded Analytics Implementations
6.1. Healthcare: Enhancing Patient Outcomes
A large healthcare provider implemented embedded analytics to improve patient care and outcomes. By integrating real-time data analysis and visualization tools within their electronic health record (EHR) system, clinicians could access relevant patient information and insights directly during consultations. This led to more informed treatment decisions, better resource allocation, and improved patient outcomes.
6.2. Retail: Personalized Customer Experiences
A major retailer used embedded analytics to gain insights into customer behavior and preferences. By embedding these insights into their e-commerce platform, the retailer was able to deliver personalized product recommendations and promotions based on individual customer profiles. This resulted in increased customer engagement, higher conversion rates, and improved customer satisfaction.
6.3. Manufacturing: Streamlining Supply Chain Management
A global manufacturing company integrated embedded analytics into its supply chain management system to optimize production planning and inventory control. By providing real-time visibility into demand patterns, production capacities, and inventory levels, the company was able to make more informed decisions regarding production scheduling and resource allocation. This led to reduced lead times, minimized stockouts, and significant cost savings.
6.4. Finance: Real-Time Risk Assessment
A leading financial institution implemented embedded analytics to enhance its risk assessment and management processes. By incorporating real-time data analysis and visualization tools into its core banking platform, the institution could quickly identify potential risks, such as credit defaults or fraudulent transactions, and take proactive measures to mitigate them. This enabled the financial institution to maintain a more accurate risk profile, improve its regulatory compliance, and reduce potential losses.
The embedded analytics solution also allowed the institution to provide personalized financial advice to its clients by analyzing their financial history, goals, and risk tolerance. This resulted in more tailored investment recommendations, increased client satisfaction, and higher customer retention rates.
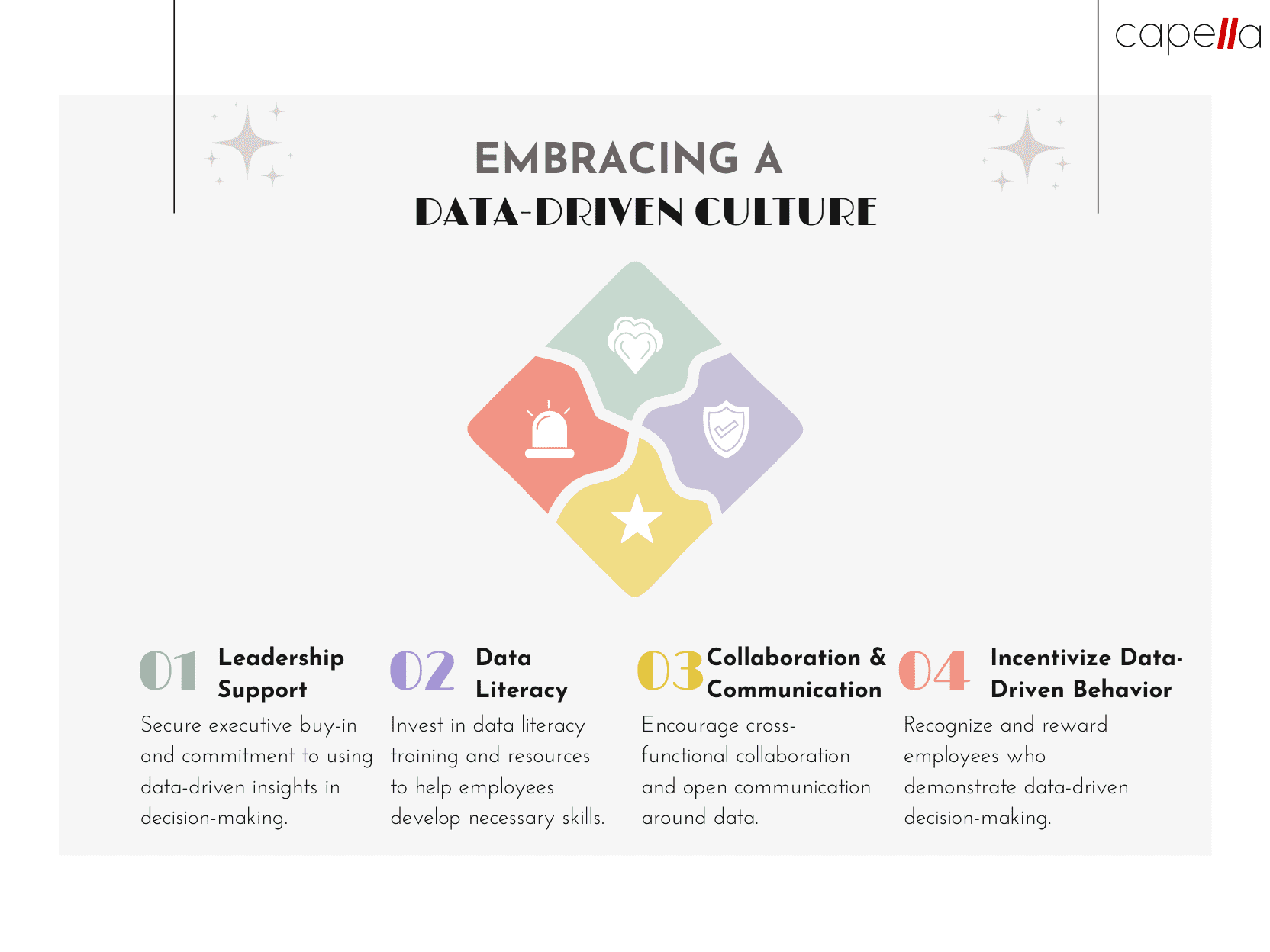
7. Conclusion
7.1. The Growing Importance of Embedded Analytics
As the business landscape becomes increasingly data-driven, the need for tools that enable quick and informed decision-making is paramount. Embedded analytics has emerged as a powerful solution for organizations seeking to harness the full potential of their data by integrating insights directly into their applications and workflows. With its ability to improve decision-making, enhance user experience, increase productivity, and streamline processes, embedded analytics is poised to become a critical component of modern business operations.
7.2. Taking the First Steps Towards Implementation
Implementing embedded analytics requires careful planning, assessment of existing infrastructure, and collaboration across various teams within an organization. By following best practices, evaluating potential solutions based on organizational needs and goals, and prioritizing data quality and security, businesses can successfully incorporate embedded analytics into their workflows and applications. As more organizations recognize the value of data-driven insights, those that adopt embedded analytics will be well-positioned to maintain a competitive edge and drive long-term success.
7.3. Overcoming Implementation Challenges
Successfully implementing embedded analytics may involve overcoming certain challenges, such as data silos, integration complexities, and change management. Organizations should address these issues proactively by:
- Breaking Down Data Silos: Facilitating data sharing and collaboration across departments and teams is critical for deriving meaningful insights. Establishing cross-functional teams and implementing data governance policies can help break down data silos and ensure data consistency throughout the organization.
- Simplifying Integration: Integrating embedded analytics into existing applications and workflows can be complex, particularly if dealing with legacy systems. Organizations should consider leveraging APIs, microservices, and other modern integration methods to simplify the process and reduce the time-to-value of their embedded analytics solution.
- Managing Change: Introducing embedded analytics may require changes in existing processes, workflows, and user behavior. A well-planned change management strategy, including clear communication, training, and support, can help ease the transition and ensure successful adoption.
7.4. Staying Ahead with Emerging Technologies
As technology continues to evolve, organizations must stay informed about emerging trends and technologies that could enhance their embedded analytics capabilities. Some areas to watch include:
- Artificial Intelligence (AI) and Machine Learning: Incorporating AI and machine learning techniques into embedded analytics can help organizations uncover deeper insights, automate complex analysis, and make more accurate predictions based on their data.
- Natural Language Processing (NLP): NLP enables embedded analytics tools to understand and process human language, allowing users to interact with data using conversational interfaces. This can make data analysis more accessible to non-technical users and improve overall user experience.
- Internet of Things (IoT) and Real-Time Analytics: As the IoT continues to grow, businesses can leverage real-time analytics to process and analyze the vast amounts of data generated by connected devices. Embedded analytics solutions that can handle real-time data can provide organizations with up-to-the-minute insights, enabling them to react quickly to changing conditions and emerging trends.
- Augmented Analytics: Augmented analytics combines AI, machine learning, and NLP to automate data analysis and interpretation, making it easier for users to derive insights and make data-driven decisions. By incorporating augmented analytics capabilities, embedded analytics solutions can further enhance user experience and improve decision-making processes.
- Data Privacy and Ethical Considerations: As data-driven decision-making becomes increasingly prevalent, organizations must ensure they adhere to data privacy regulations and ethical guidelines. Embedding privacy-preserving analytics techniques, such as differential privacy and federated learning, can help organizations protect sensitive data while still deriving valuable insights.
7.5. Embracing a Data-Driven Culture
A successful embedded analytics implementation goes beyond technology and requires organizations to cultivate a data-driven culture. This involves promoting a mindset that values data-driven insights and encourages employees at all levels to use data in their decision-making processes. Key steps to foster a data-driven culture include:
- Leadership Support: Executive buy-in is crucial for creating a data-driven culture. Leaders should demonstrate their commitment to using data-driven insights in their decision-making and encourage others to follow suit.
- Data Literacy: Ensuring that employees have the necessary skills to understand, interpret, and apply data-driven insights is vital. Organizations should invest in data literacy training and provide resources to help employees develop these skills.
- Collaboration and Communication: Encouraging cross-functional collaboration and open communication around data can help break down silos and promote a unified approach to data-driven decision-making. Establishing regular data-driven discussions and meetings can facilitate this collaboration.
- Incentivize Data-Driven Behavior: Recognizing and rewarding employees who demonstrate data-driven decision-making can help reinforce the importance of using data in everyday workflows. Incentive programs, such as bonuses or recognition awards, can be used to motivate employees to adopt a data-driven mindset.
By embracing a data-driven culture and staying informed about emerging technologies, organizations can fully capitalize on the benefits of embedded analytics and maintain a competitive edge in an increasingly data-driven world. As embedded analytics continues to evolve and become more sophisticated, businesses that proactively adopt and adapt to these advancements will be better positioned to drive innovation, optimize processes, and enhance overall decision-making.
7.6. Measuring the Success of Embedded Analytics
To ensure the ongoing success and value of embedded analytics, organizations should establish a robust measurement framework that tracks relevant KPIs and evaluates the impact of embedded analytics on their business processes. This framework should include:
- User Adoption Metrics: Monitoring user adoption rates can help organizations identify areas where additional training or support may be needed. By tracking the number of active users and frequency of use, businesses can gauge the effectiveness of their embedded analytics solution.
- Business Outcome Metrics: Assessing the impact of embedded analytics on key business outcomes, such as revenue growth, cost reduction, or customer satisfaction, can help organizations determine the ROI of their implementation. These metrics can be compared to pre-implementation baselines to evaluate the overall success of the embedded analytics solution.
- Operational Efficiency Metrics: Tracking the impact of embedded analytics on operational efficiency, such as time savings, error reduction, or process improvements, can help organizations optimize their workflows and maximize the value of their data-driven insights.
By regularly monitoring and evaluating these metrics, organizations can identify opportunities for improvement, make data-driven adjustments to their embedded analytics strategy, and ensure long-term success.
Embedded analytics offers organizations a powerful tool to harness the full potential of their data and drive better decision-making. By carefully evaluating potential solutions, implementing best practices, and embracing a data-driven culture, businesses can successfully integrate embedded analytics into their applications and workflows, reaping numerous benefits such as improved productivity, streamlined processes, and enhanced user experiences.
As the importance of data-driven insights continues to grow, organizations that leverage embedded analytics will be well-positioned to maintain a competitive edge, drive innovation, and achieve long-term success in an increasingly data-driven world.
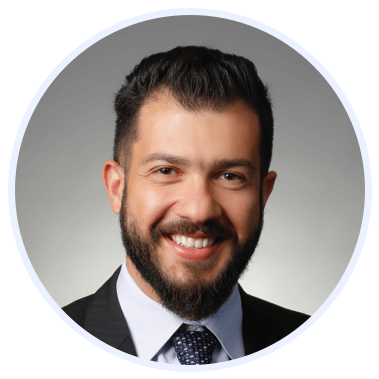
Rasheed Rabata
Is a solution and ROI-driven CTO, consultant, and system integrator with experience in deploying data integrations, Data Hubs, Master Data Management, Data Quality, and Data Warehousing solutions. He has a passion for solving complex data problems. His career experience showcases his drive to deliver software and timely solutions for business needs.