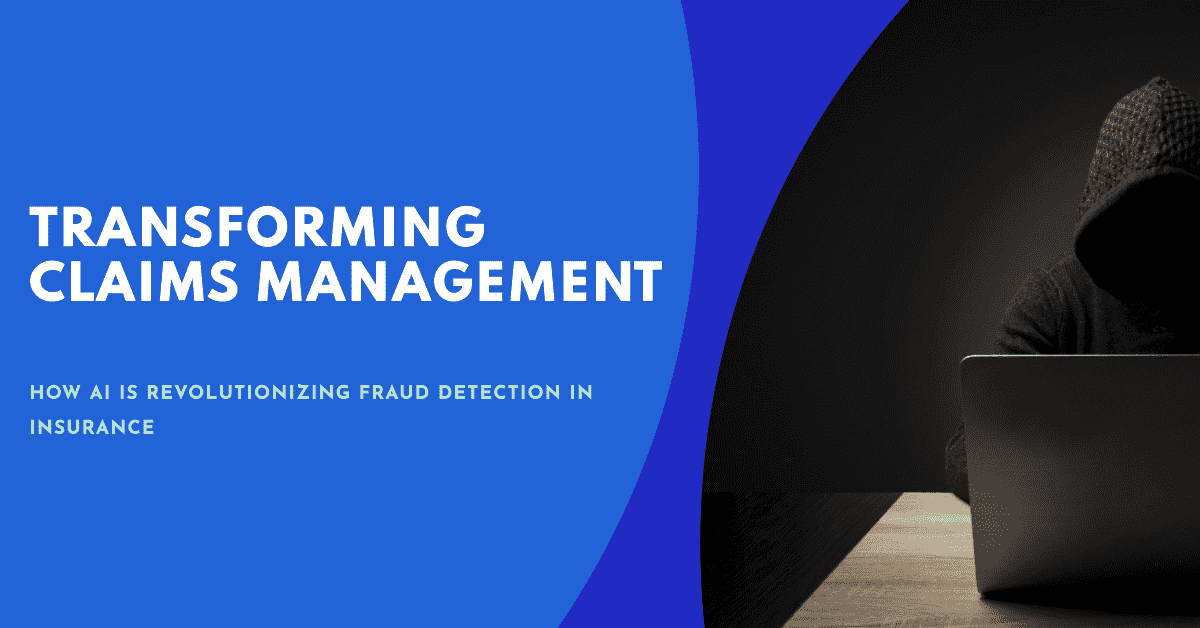
Fraud is a major problem in the insurance industry, costing billions of dollars each year. From staged accidents to inflated claims, fraud adds significant unnecessary costs and can drive up premiums for honest policyholders.
Insurers have a duty to identify and prevent fraud, but with thousands of claims filed each day, manually reviewing each one would be time-consuming and inefficient. Fortunately, advances in artificial intelligence are providing insurers with powerful new fraud detection capabilities.
In this post, we’ll explore:
- The scale and impact of insurance fraud
- Traditional fraud detection techniques and their limitations
- How AI is transforming insurance fraud detection and prevention
- Real-world examples of AI in action across auto, health, and P&C insurance
- Best practices for an effective AI fraud strategy

The Extent and Impact of Insurance Fraud
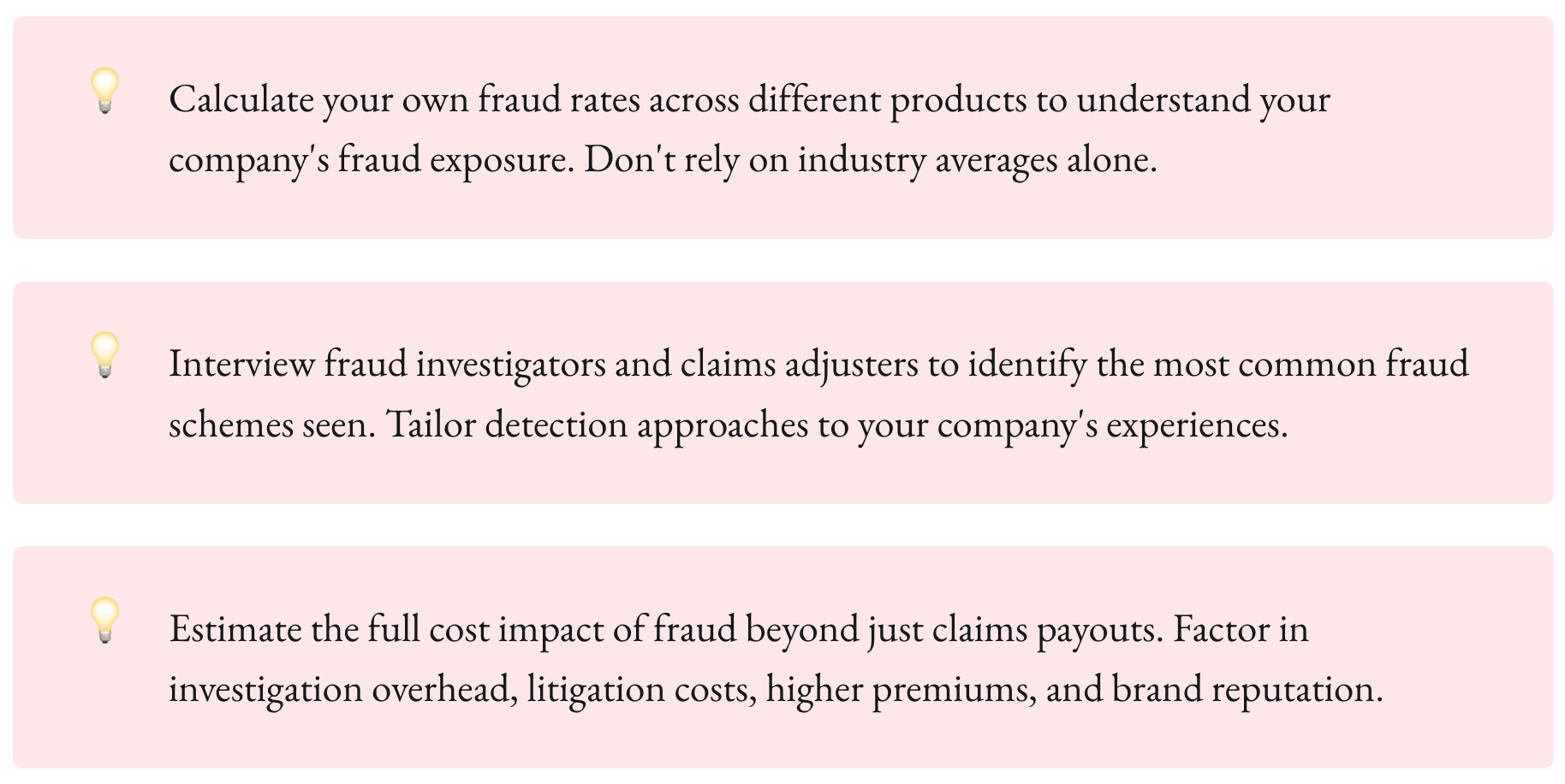
Insurance fraud comes in many forms, from consumers exaggerating claims to elaborate criminal rings staging accidents. No type of policy is immune. According to the Coalition Against Insurance Fraud:
- Auto insurance fraud costs $29 billion per year
- Workers comp fraud costs up to $30 billion annually
- Healthcare fraud costs up to $68 billion per year
For insurers, the costs go far beyond just paying out fraudulent claims. They also must contend with:
- High investigation costs to detect fraud
- Litigation expenses to fight fraud
- Inflated premiums that hurt honest policyholders
- Reputational damage when fraud makes the news
For consumers, fraud results in higher premiums and prices across the board. Insurance fraud is not a victimless crime.
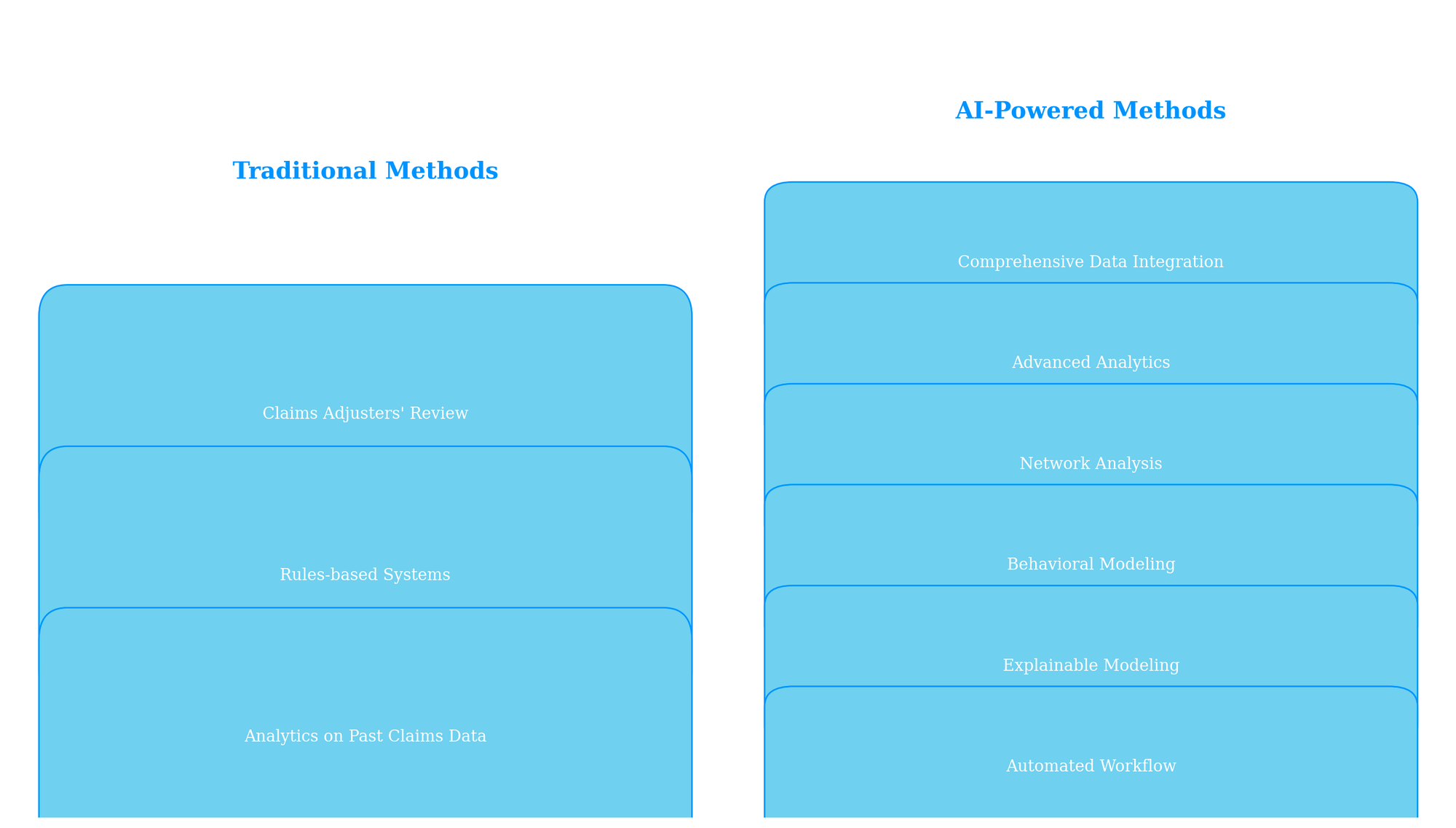
The Limits of Traditional Fraud Detection
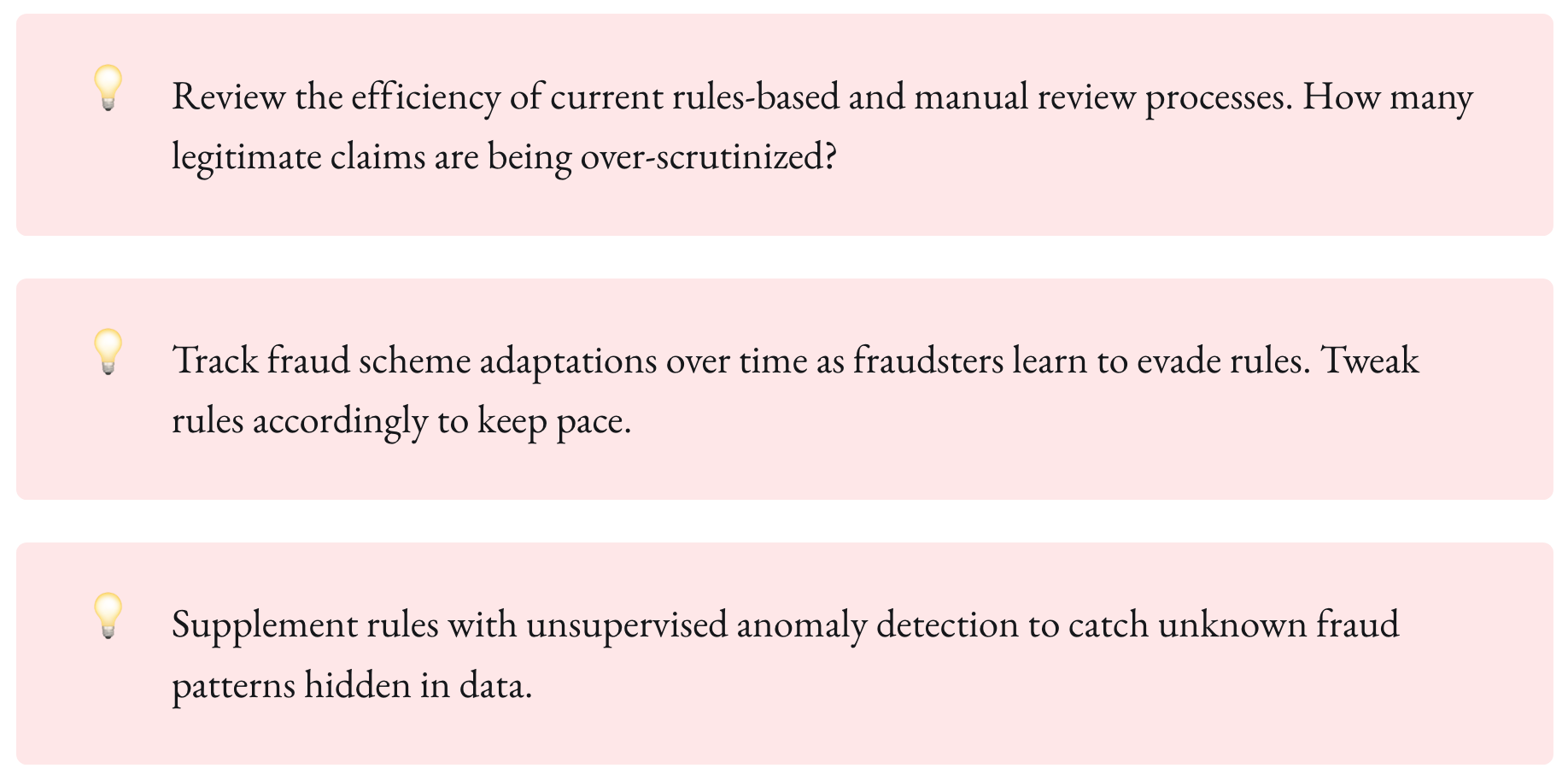
Insurers have long relied on manual red flags and rules-based systems to detect potential fraud. Common traditional techniques include:
- Claims adjusters manually reviewing claims for red flags like inflated damage amounts or suspicious previous claims. This approach depends heavily on individual human judgment.
- Rules-based systems that flag claims according to pre-set rules like deposits over $10k or extremely short time between accident and repair. However, fraudsters can find ways around rules over time.
- Analytics on past claims data to identify factors correlated with fraud like certain geographic regions or policy terms. This approach relies on backward-looking data.
While these techniques provide a baseline level of fraud screening, each has significant limitations:
High manual effort: Claims staff must review many legitimate claims to catch just a few fraudulent ones. This tradeoff reduces efficiency.
Easy to evade: Fraudsters can adapt to predefined rules and red flags over time. Systems based on past data may miss new fraud patterns.
Narrow focus: Traditional methods focus mainly on claims rather than full policy lifecycles. Some fraud only becomes visible based on behavioral patterns over time.
Limited insights: Manual review makes it difficult to spot complex patterns at a systemic level. Rules-based systems have no real intelligence behind flagging.
Reactive approach: Most techniques only detect fraud after a claim is filed rather than proactively preventing it beforehand.
To overcome these challenges, leading insurers have turned to artificial intelligence as the next generation of fraud defense.
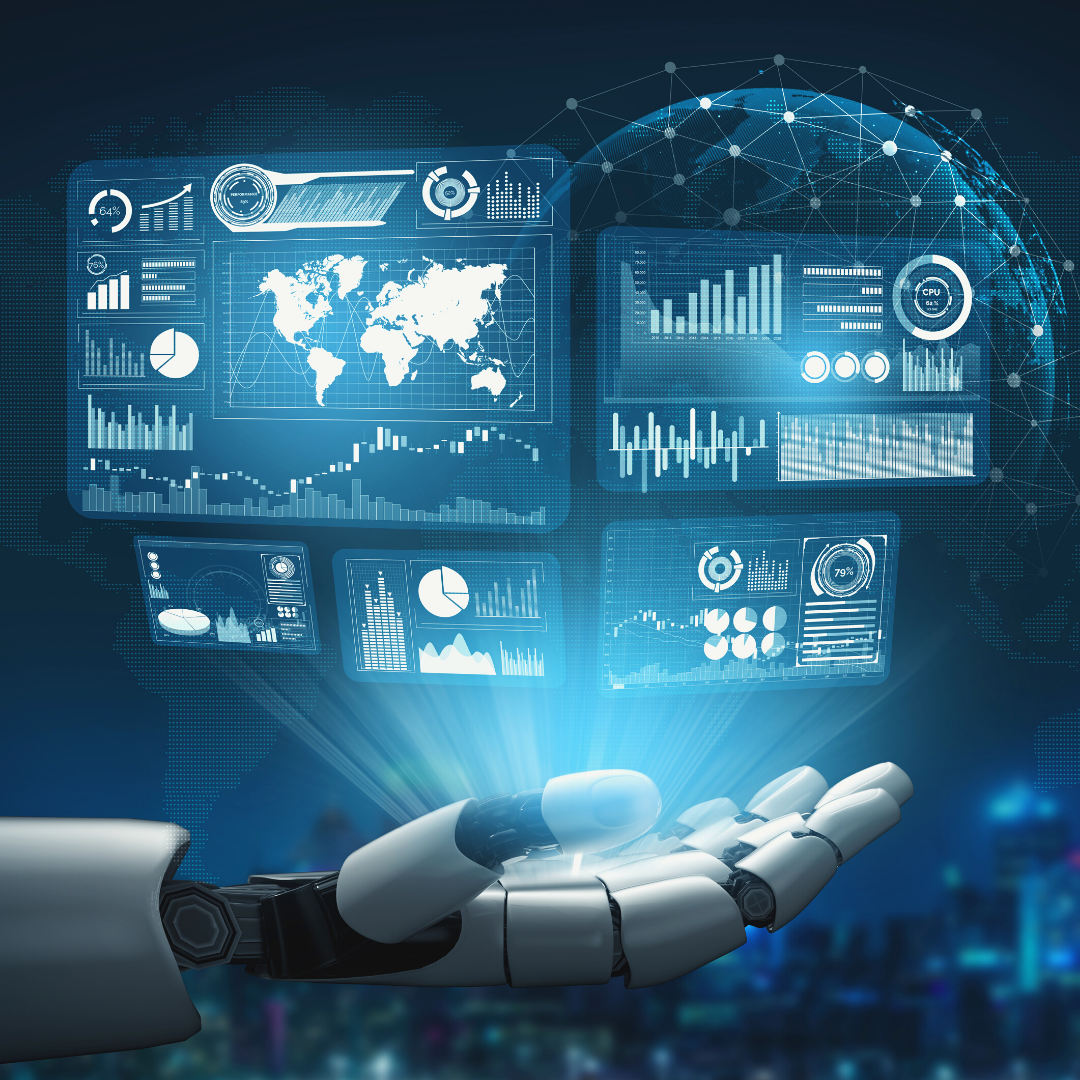
AI-Powered Fraud Detection in Action
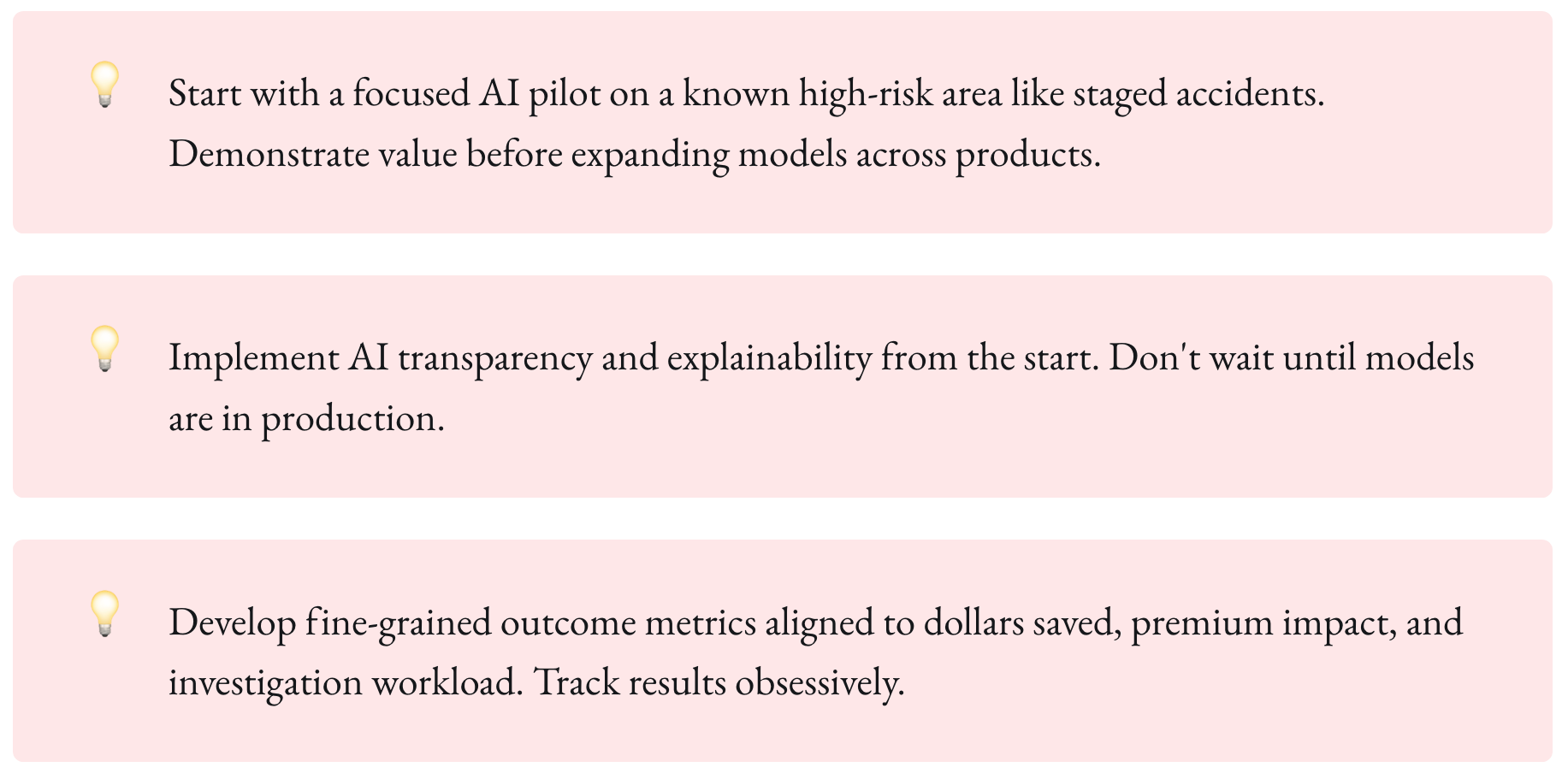
Modern AI fraud solutions move beyond just flagging suspicious claims to holistic protection across the policy lifecycle. Key capabilities include:
Comprehensive Data Integration
AI systems pull data from across core insurance systems, third-party data sources, and unstructured data like claims notes and call center transcripts. This provides a 360-degree customer view.
Advanced Analytics
Sophisticated machine learning algorithms uncover complex relationships and patterns associated with fraud, far beyond rules-based approaches. Models are continually updated as new data comes in.
Network Analysis
By analyzing connections between claimants, providers, addresses, etc., AI can uncover fraud rings spanning multiple polices and individuals. This network perspective is crucial for organized fraud.
Behavioral Modeling
AI looks at customer behavior before, during, and after applying for insurance to detect patterns like churn risks. This proactive approach provides visibility earlier in the process.
Explainable Modeling
Many AI models are “black boxes”, making it hard to understand why a claim is flagged. Explainable AI provides visibility into model logic and why certain claims are high-risk.
Automated Workflow
Once AI flags a suspicious claim, insurers can automatically initiate fraud investigations and send claims to specialized teams rather than front-line adjusters.
Let’s look at real-world examples of how AI is transforming fraud defense across different insurance lines:
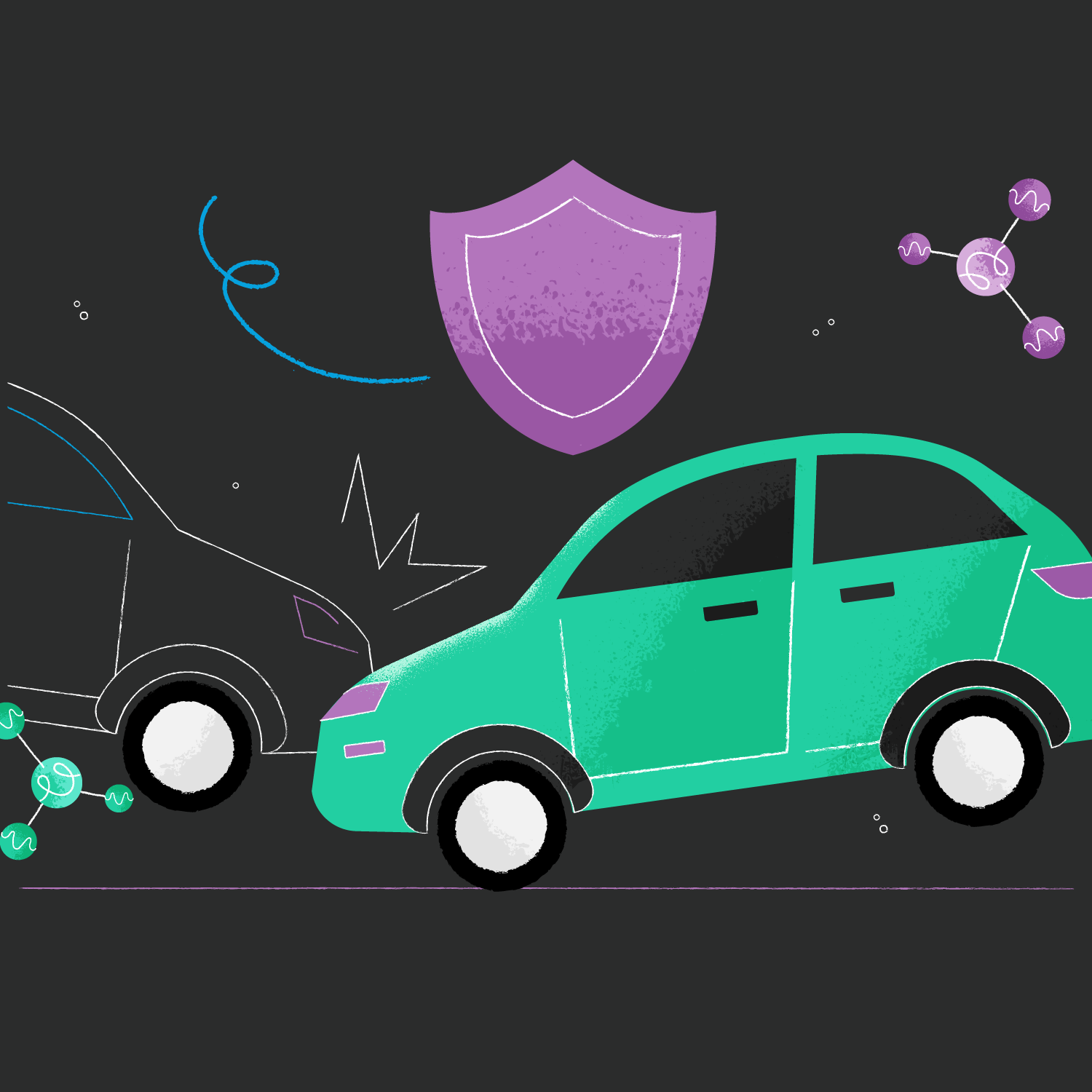
Auto Insurance Fraud Detection
For auto insurers, a major pain point is staged accident rings that repeatedly file inflated claims across different policies. AI helps insurance companies stay steps ahead:
- Detect connected claims: By analyzing metadata like addresses and phone numbers, AI can detect clusters of claims associated with fraud rings. This allows investigators to study claims holistically rather than in isolation.
- Uncover staged accidents: Sophisticated image analytics on photos can identify staged damage inconsistent with reported accident details. For example, AI can detect dents made by hand rather than another vehicle.
- Model driver behavior: Telematics data from policyholders’ connected cars allows modeling of driver safety habits. Drastic changes in behavior before accidents suggest potential fraud.
- Stop fraud rings earlier: Many fraud rings test initial claims before ramping up. By detecting even minor linked claims early, AI models can identify these test runs and prevent bigger future losses.
Liberty Mutual saved $15 million on auto claims in one year using AI to identify potentially fraudulent activity much earlier.
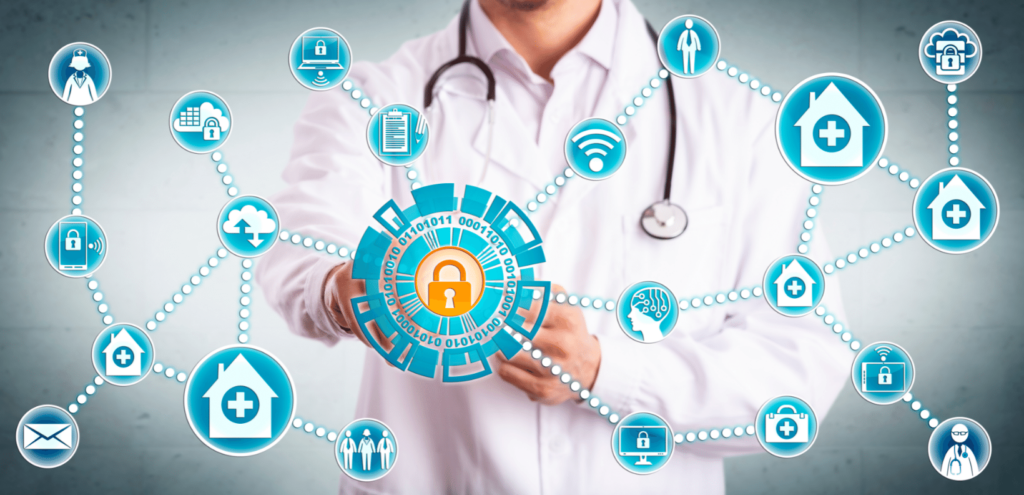
Healthcare Insurance Fraud Detection
For health insurers, common schemes include billing for unnecessary procedures or services never performed. AI helps flag suspicious claims:
- Detect overbilling: By comparing claims to records of diagnosis and treatment, AI can detect cases where too many procedures were billed for a patient’s condition.
- Uncover excessive testing: AI can model the typical cadence of tests needed for medical conditions to detect unusual spikes in testing claims.
- Identify ineligible recipients: AI can cross-check patient age, medical history, and other details against billed treatments to detect patients receiving inappropriate care.
- Stop identity fraud: Analytics on client profiles can identify suspicious personally identifiable information that suggests identity fraud.
In one case, AI helped a health insurer uncover $1.2 million in fraud from a clinic billing for fictitious patients.
P&C Insurance Fraud Detection
In property and casualty insurance, inflated or exaggerated claims are common. AI looks across claims data to identify suspicious patterns:
- Detect premium timing tricks: AI analyzes the timing between policy purchase and claims filing date to identify quick claims that suggest pre-planning fraud.
- Model contractor costs: AI builds profiles of typical costs for services like emergency clean-up to identify inflated contractor invoices submitted across claims.
- Identify previous connected claims: Cross-referencing historical claims data uncovers suspicious repeat claim filers across different properties and events.
- Build risk profiles: Policy applications are analyzed to detect signs of high fraud risk including illogical data combinations, missing information, or answers that deviate from similar applicants.
One insurer saw a 15% drop in auto and property claims costs from using AI to improve fraud prediction by 50% from previous methods.
Best Practices for AI Fraud Prevention
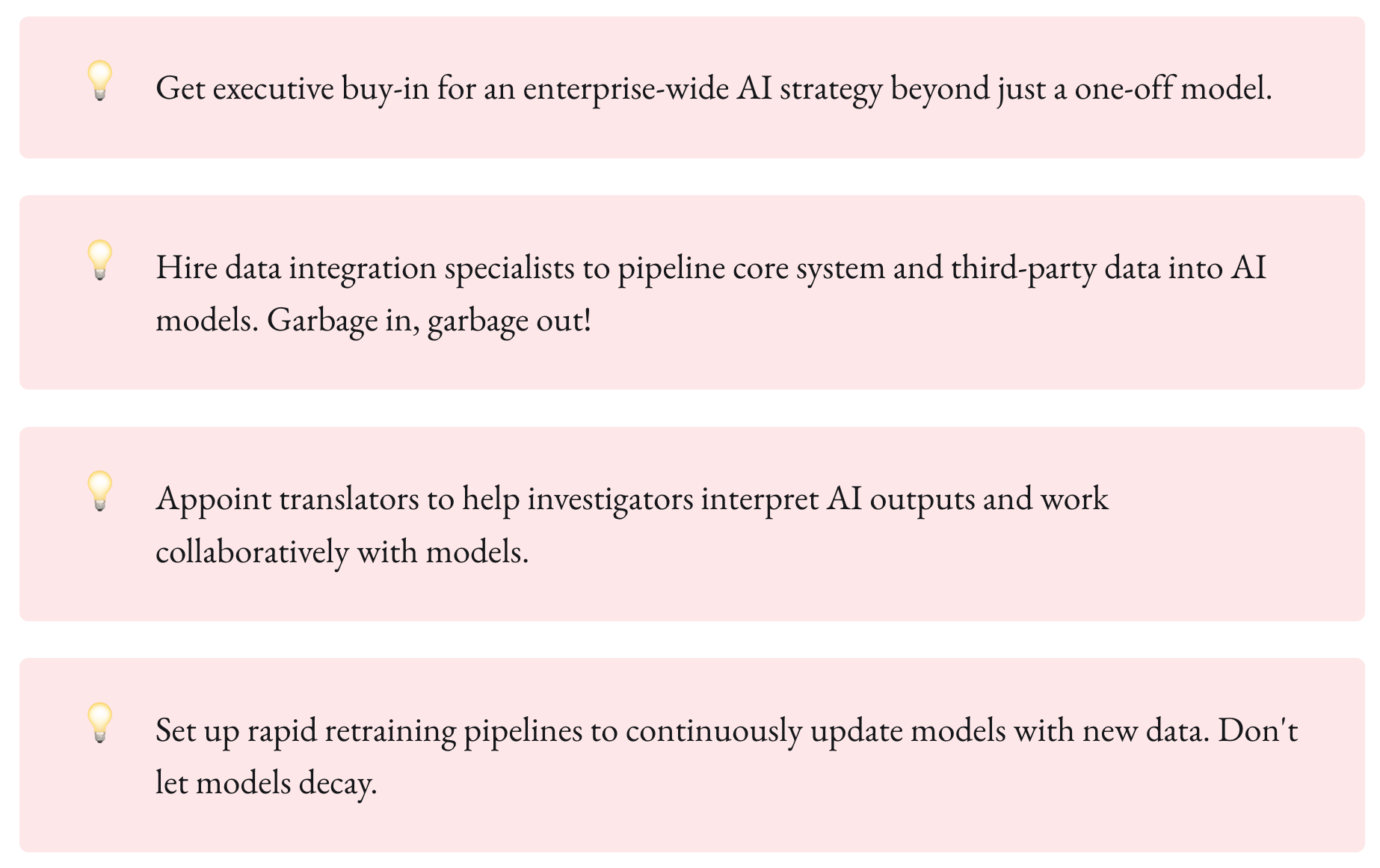
With AI models improving each month, what’s the most effective way for insurers to leverage AI for fraud detection and prevention? Here are a few key best practices:
- Take an enterprise view: Connect AI models to core systems like underwriting, claims, SIU, and third-party data to get the full picture across policy lifecycles.
- Focus models on business outcomes: Optimize AI to directly reduce fraud losses and contain premiums rather than just improving model metrics like accuracy.
- Enable explainability: Interpretability helps investigators understand model decisions and more easily prove fraud. Start with transparent AI models and add explainability modules as needed.
- Iterate models frequently: Fraud patterns change quickly, so regularly retrain models on new data. Cloud-based AI makes model retraining easier to manage.
- Treat models as baselines: AI provides signals not slam dunks. Investigators still need to evaluate flagged claims before formally accusing policyholders of fraud.
- Enhance human intelligence: AI is an assistant, not a replacement. Enable staff to leverage AI via insights dashboards and automated workflows.
Looking Ahead
As AI capabilities continue to evolve, insurance fraud detection will become increasingly proactive. Insurers will be able to stop more fraud before policies are ever issued. Models will expand from claims analysis to holistic profiling of risk across customers, providers, regions, and product lines.
Rather than an isolated tool, AI will become the connective tissue that binds an insurer’s full suite of anti-fraud initiatives together for maximum impact. Companies that embrace AI to elevate their fraud defense will gain a lasting competitive advantage.
Key Takeaways
- Insurance fraud costs billions each year and drives up premiums for honest policyholders
- Traditional fraud detection has high manual effort, is easy to evade, and takes a reactive approach
- AI analyzes comprehensive data, detects complex patterns, and enables holistic fraud prevention
- Real-world examples show AI impact across auto, health, and P&C insurance
- Best practices include enterprise data connections, focusing on outcomes, and enabling human-AI collaboration
The possibilities are exciting as AI transforms insurance fraud detection from a claims-centered cost center to an enterprise profit protection strategy. With vigilance and the right solutions, the future looks bright for turning the tide against insurance fraud.
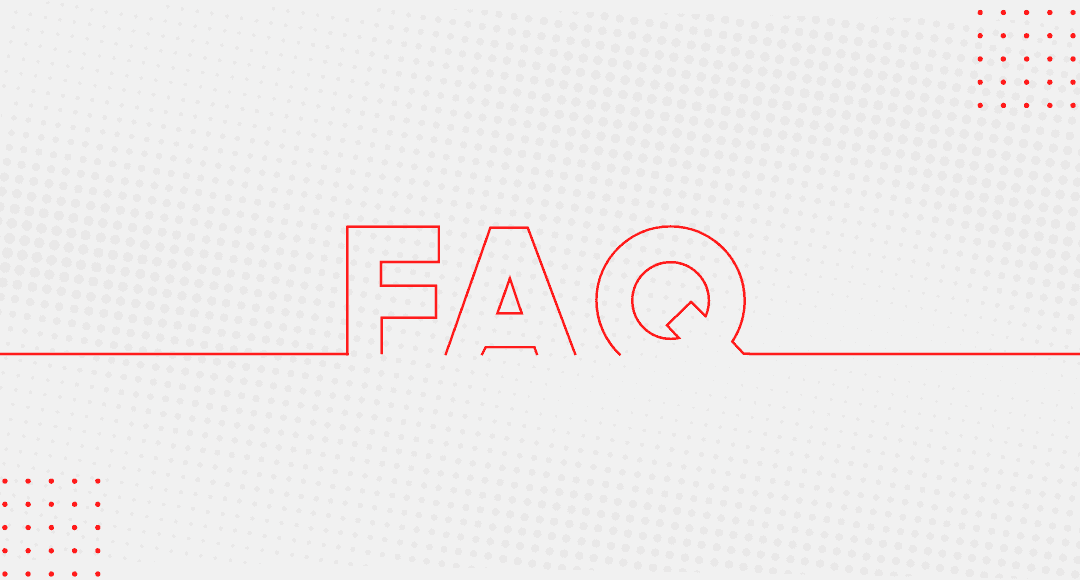
1. How prevalent actually is insurance fraud?
Insurance fraud is extremely widespread, costing the industry tens of billions per year. The Coalition Against Insurance Fraud estimates that fraud amounts to about 10% of property-casualty insurance losses and loss adjustment expenses each year. For health insurance, the National Health Care Anti-Fraud Association estimates 3% of all health care spending in the U.S. is lost to fraud, totaling over $68 billion annually. Overall, fraud costs each family $400-700 per year in the form of increased premiums.
2. What are some of the most common insurance fraud schemes?
Some of the most frequent insurance fraud schemes include:
- Staged auto accidents - Fraud rings deliberately crash cars together and exaggerate vehicle damage to file inflated claims.
- Premium timing tricks - Policyholders wait until just after buying a policy to report an "incident" and quickly file a claim.
- Billing for unnecessary care - Healthcare providers perform and bill for excessive procedures, tests, and services that patients don't actually medically require.
- Identity fraud - Imposters use stolen personal information to file medical claims and receive reimbursement.
- Contractor invoice inflation - Contractors submit exaggerated invoices for emergency services like fire cleanup through home insurance claims.
- Faking injuries - Individuals pretend to suffer injuries from a staged accident and file disability and medical claims.
3. Why do insurers have difficulty detecting fraud?
Insurers struggle to detect all forms of fraud for several reasons:
- Large volume of claims makes manual review infeasible
- Fraud is intentionally hidden and disguised to appear legitimate
- Schemes like staged accidents involve coordination across multiple policies
- Traditional rules only flag basic suspicious attributes not complex patterns
- Past claims data alone misses new types of emerging fraud
- Difficult to prove intent necessitating meticulous investigation
The result is that many fraudulent claims slip through undetected.
4. How can AI technology help insurers improve fraud detection?
AI helps insurers overcome many limitations of traditional fraud detection through:
- Sophisticated analytics - Machine learning detects complex patterns and anomalies invisible to rules-based systems.
- Network analysis - Connections between entities reveal organized fraud spanning policies.
- Behavioral profiling - Analysis of behaviors before, during, and after claims uncovers risks.
- Comprehensive data - AI incorporates both structured (e.g. policies) and unstructured data (e.g. claims notes).
- Hyper-parameter tuning - Advanced models are optimized to balance fraud catches and false positives.
- Continuous retraining - Models stay updated detecting the latest fraud schemes.
5. What AI techniques work best for insurance fraud detection?
Some of the most effective AI techniques include:
- Neural networks excel at finding hidden relationships within claim attributes and metadata that correlate with fraud.
- Decision trees build human-interpretable models with branching logic to segment claims by risk levels.
- Unsupervised learning detects completely new anomalies and patterns unlike past fraud data.
- Text mining extracts insights from unstructured text data like claims notes and call transcripts using NLP.
- Graph analysis maps connections between entities to uncover coordinated fraud networks.
The most advanced fraud AI leverages an ensemble of multiple techniques together.
6. How can insurers start applying AI for fraud detection?
The best practice is to start with a targeted high-impact pilot before expanding AI across all claims. Key steps include:
- Select a focused business problem like auto accidents with clear fraud costs.
- Assemble relevant structured data like claims, policies, repairs as well as any unstructured data.
- Build, train, validate, and continuously improve AI models on this data.
- Integrate models into workflows to automatically flag likely fraudulent claims.
- Track measurable reduction in fraud losses, containment of premiums, and investigation efficiency.
- Iterate and expand to other products applying lessons learned.
7. What risks or pitfalls should insurers be aware of with AI fraud detection?
Some risks to avoid include:
- Treating AI as a "black box" without transparency into why certain claims are flagged.
- Disrupting existing fraud investigation workflows by inserting AI poorly.
- Lacking the expertise to properly validate, maintain, and update models over time.
- Focusing too much on improving model accuracy metrics rather than real business results.
- Not having enough quality training data across diverse fraud cases required for accurate models.
8. How can insurers avoid inadvertently flagging legitimate claims as fraud?
Strategies to minimize false positives include:
- Selecting AI models that apply precise probability scores rather than binary decisions. This allows adjusting the sensitivity threshold to optimize for fraud catches vs mistakes.
- Using explainable AI models with human understandable logic so investigators can assess the reasonableness of flags.
- Maintaining human-in-the-loop review with AI only supporting and not replacing human claims assessment.
- Monitoring model scoring performance across different customer segments to check for accuracy consistency.
- Retraining models rapidly with data on mistaken flags to continuously improve precision.
9. What level of fraud reduction is achievable with AI? How does this impact premiums?
Results vary substantially by insurer, but top performers have achieved up to 30-40% reduction in fraud losses by switching from rules-based systems to advanced AI. For a large insurer, this could translate to over $100M in fraud savings annually and over $1B over a decade. These direct cost savings allow insurers to reduce premiums while maintaining or increasing profits. Leading insurers are already passing AI-enabled savings back to customers in the form of lower premiums.
10. Will fraudsters find ways to evade AI detection?
Like any technology, AI alone will not eliminate all forms of fraud. Sophisticated fraud rings will adapt to avoid detection by AI-driven patterns and rules over time. However, AI makes this cycle of fraud evolution much harder through:
- Faster model updating with new data on emerging fraud patterns
- Continued development of new modeling techniques by AI researchers
- Layering multiple different types of AI analysis for redundancy
- Expanding data sources to make fraud harder to hide
While the "fraud detection race" will continue, AI enables insurers to stay steps ahead of criminals like never before. This game of cat and mouse between insurers' AI capabilities and adaptable fraudsters will endure.
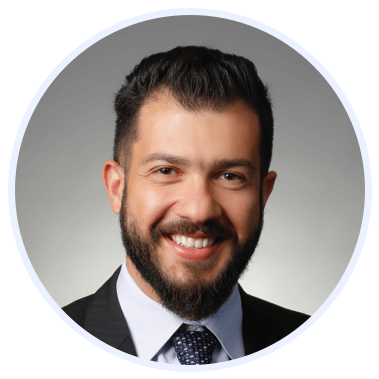
Rasheed Rabata
Is a solution and ROI-driven CTO, consultant, and system integrator with experience in deploying data integrations, Data Hubs, Master Data Management, Data Quality, and Data Warehousing solutions. He has a passion for solving complex data problems. His career experience showcases his drive to deliver software and timely solutions for business needs.