
The buzz around Artificial Intelligence (AI) is hard to ignore. It's everywhere. From self-driving cars to smarter search algorithms, it promises to reshape the world as we know it. But here's the thing: while the headline-grabbing headlines focus on the glitz and glamour of AI’s futuristic potential, few address the raw, gritty reality of how it can be practically applied to solve real-world business problems.

Operational bottlenecks are one of the biggest challenges companies face, and they can significantly hinder growth. But what if you could not only identify those bottlenecks but eliminate them entirely? What if AI could not just automate routine tasks but actively improve your processes, making them smarter, faster, and more efficient? That’s not science fiction. It's happening right now.
In this post, I want to walk through how AI can help you move from bottlenecks to breakthroughs, making your operations smoother and setting the stage for scalable growth.
Bottlenecks in Operations
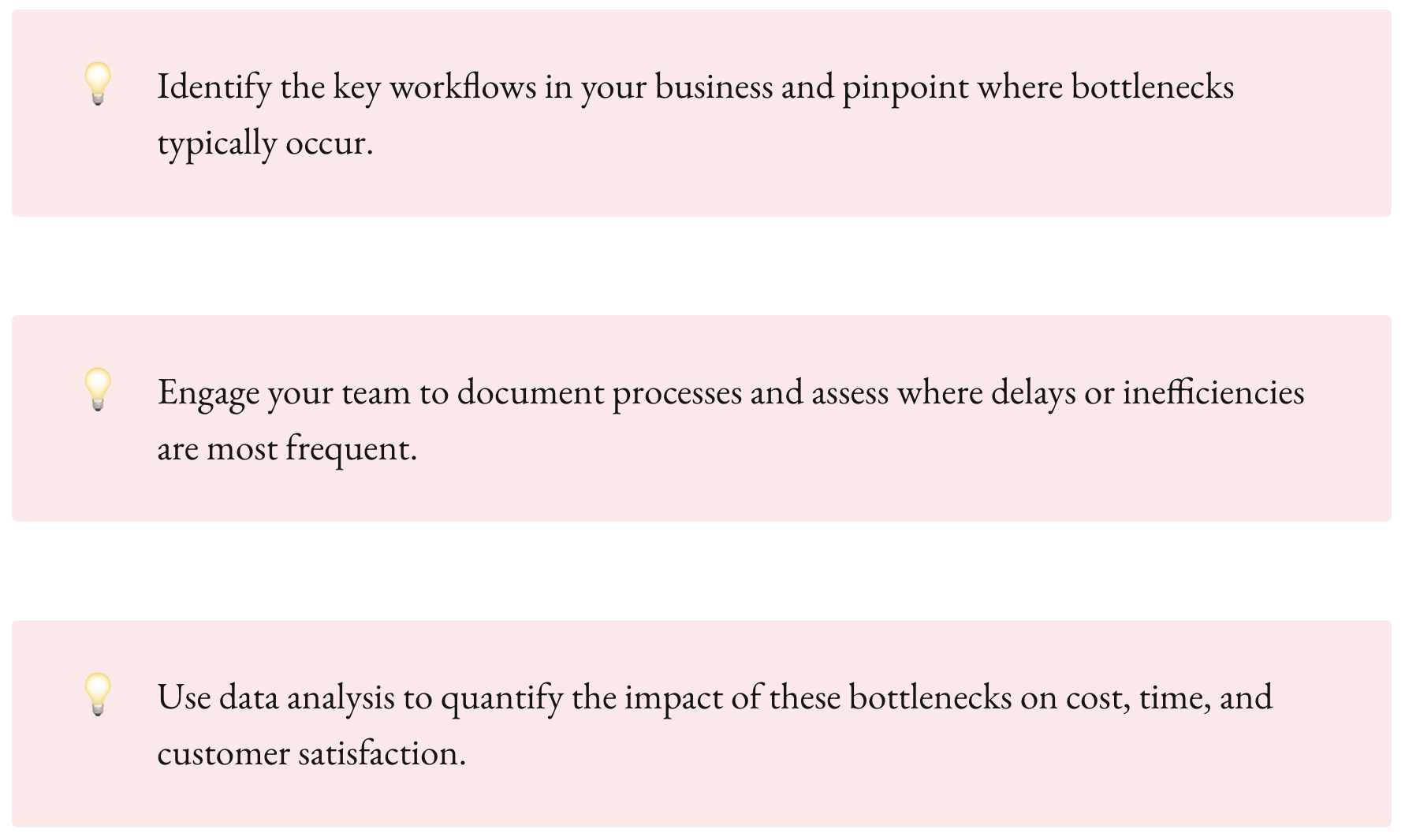
Bottlenecks—those pesky points in your workflow where things slow down, grind to a halt, or just don’t scale as fast as you’d like. They are silent killers, often overlooked until they’ve already caused significant damage. I’m sure you’ve seen it: a critical task that takes far longer than expected. A decision-making process that’s mired in inefficiencies. A backlog of work that never seems to clear.

What’s worse is that many of these bottlenecks exist because of outdated systems, human error, or poor communication. But here’s the twist: when you rely on these methods, you’re essentially setting yourself up for failure in a world that demands speed, agility, and data-driven precision. In today’s competitive landscape, the slightest operational hiccup can mean lost customers, increased costs, and missed opportunities.
AI, however, has the potential to fix these pain points and deliver substantial improvements in efficiency. But before diving into AI's role, let’s break down the most common types of operational bottlenecks.

Common Operational Bottlenecks:
- Manual Processes and Repetitive Tasks
Whether it’s data entry, customer support ticket triaging, or invoice processing, manual tasks suck up valuable resources. They also create a significant risk for error and inefficiency, especially as your business scales. - Decision-Making Delays
Business decisions often take longer than they should. This is because decision-makers rely on outdated or incomplete data, leading to slow or poorly informed decisions. - Underutilization of Resources
Many businesses have underutilized assets, be it human talent, machinery, or data. Bottlenecks happen when resources are stuck in a holding pattern, waiting for a trigger to activate their potential. - Inventory and Supply Chain Issues
This is a classic case where a slight delay in one part of the chain cascades and disrupts the entire process. Think delayed shipments, overstock, understock, or inefficient routing of goods.
AI is built to address these precisely. Let’s dive into the role it can play.
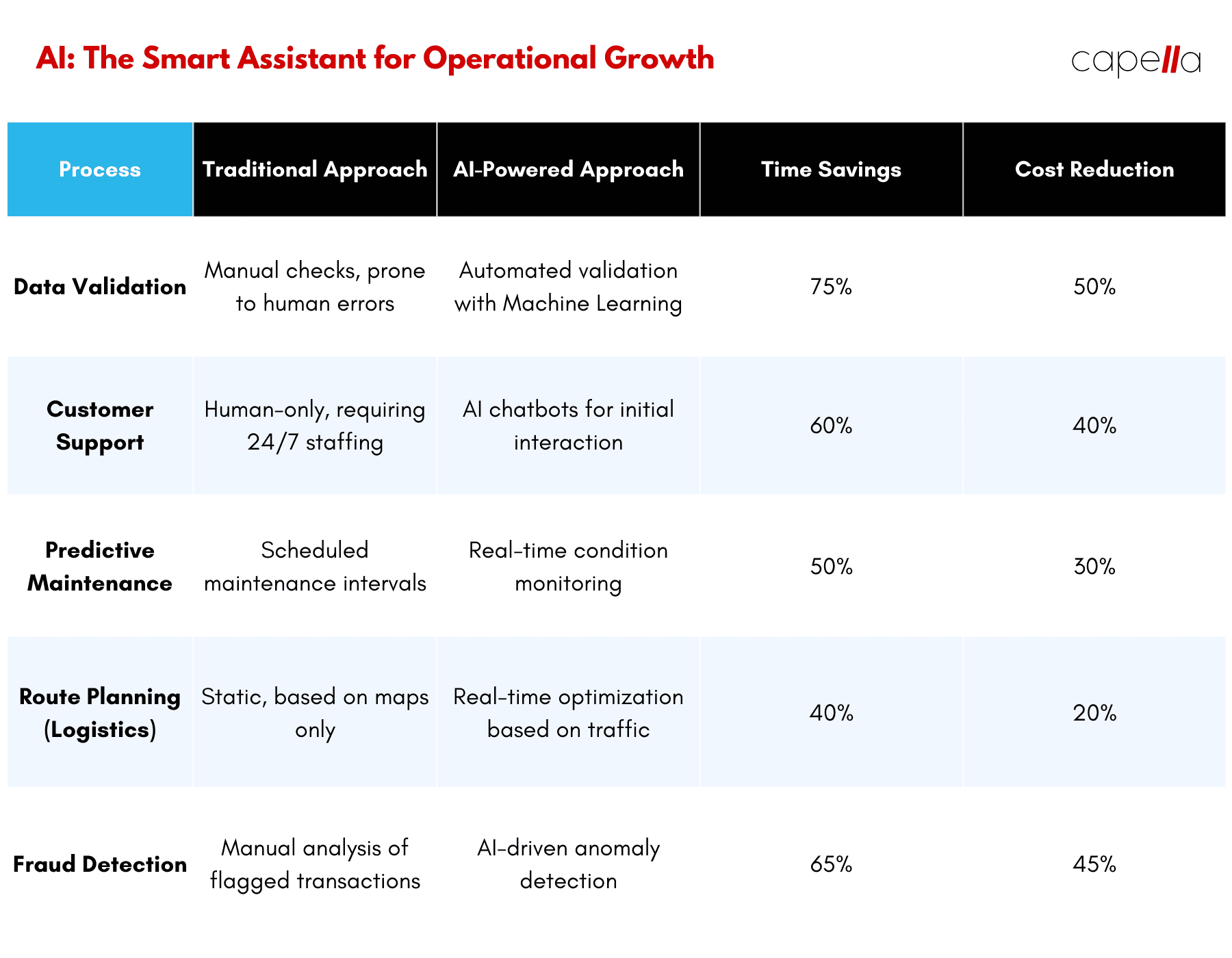
AI: The Smart Assistant for Operational Growth

Now that we’ve laid the foundation, let’s talk about AI. We’ve all heard about how AI is changing the landscape of industries like healthcare, finance, and retail. But its application in operations is equally transformative, albeit less talked about. AI isn't just a shiny tool for improving customer experience. It’s a powerhouse that can reshape how your operations function at their core.
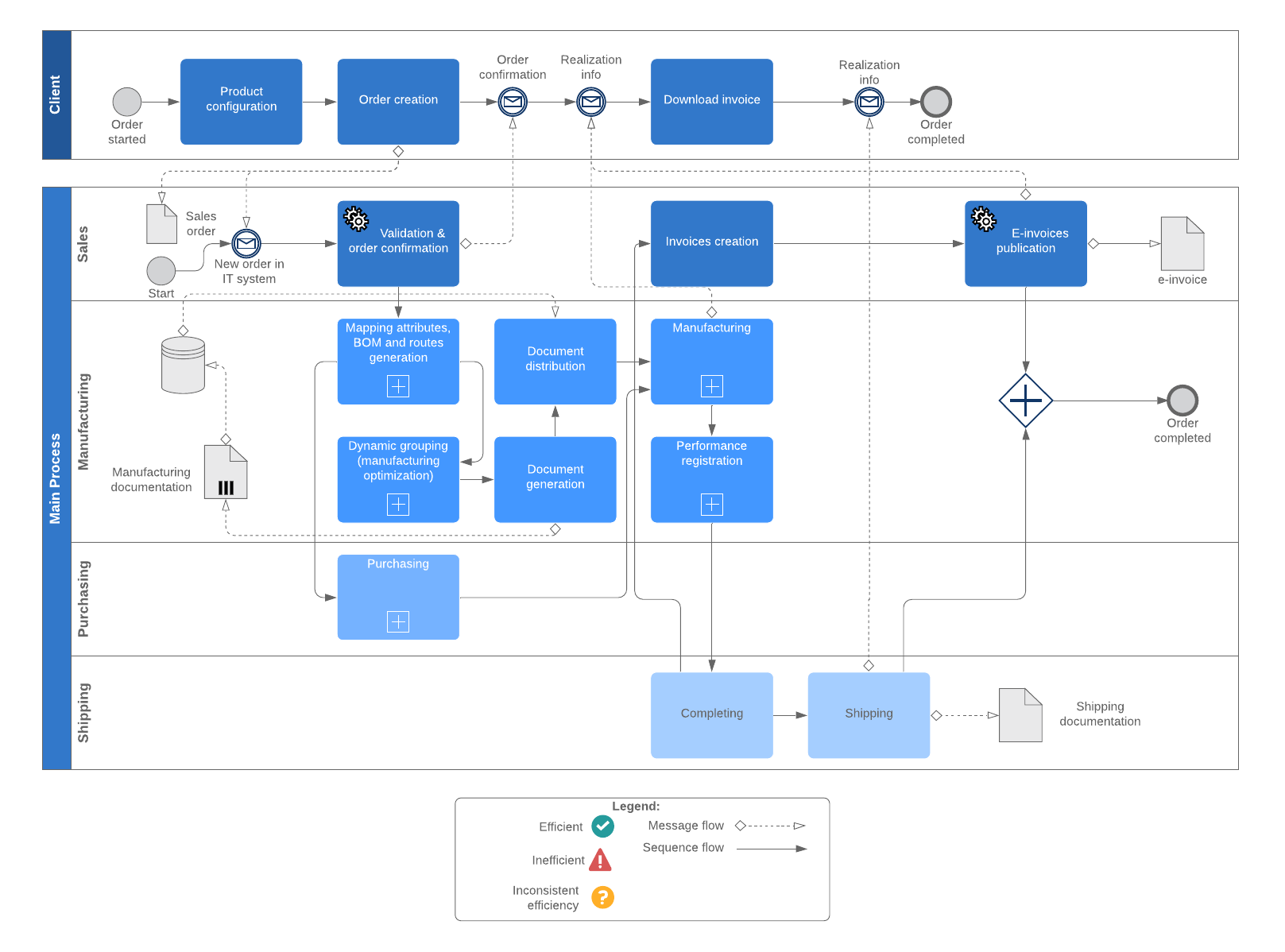
1. Automation and Process Optimization
Automation isn’t new, but AI-powered automation takes things to a whole new level. AI doesn’t just repeat tasks; it learns from data and optimizes the workflow. For example, AI-powered systems can help businesses automate customer support with advanced chatbots that handle complex queries, reducing the burden on human agents and improving response times.

Consider a company like Capella, where data management is central to our operations. One of our biggest pain points was manual data validation. We were using traditional methods to verify and process data, which was time-consuming and prone to error. By implementing an AI-driven data validation system, we reduced the time spent on validation by 75%, freeing up resources to focus on more strategic work.
Here’s how AI can optimize processes:
- Predictive Analytics: AI can predict delays before they happen. If you have a bottleneck in your supply chain, an AI-powered system can forecast issues based on historical data and suggest alternative routes or suppliers before things go sideways.
- Intelligent Routing: In logistics, AI can optimize delivery routes in real time, taking into account factors like weather, traffic, and vehicle load. This reduces fuel costs and speeds up deliveries.
Let’s take a look at an example: A leading logistics company uses AI to optimize its supply chain. By integrating machine learning algorithms into their operations, i reduced delivery delays by 20%, and their operational efficiency improved by 30%. Their system uses real-time traffic data and historical delivery patterns to suggest the fastest and most cost-effective routes.

2. Faster and Smarter Decision Making
AI doesn't just automate. It empowers better decision-making by providing insights that humans may overlook. AI systems can analyze vast amounts of data and suggest actionable strategies based on predictive modeling and historical trends.
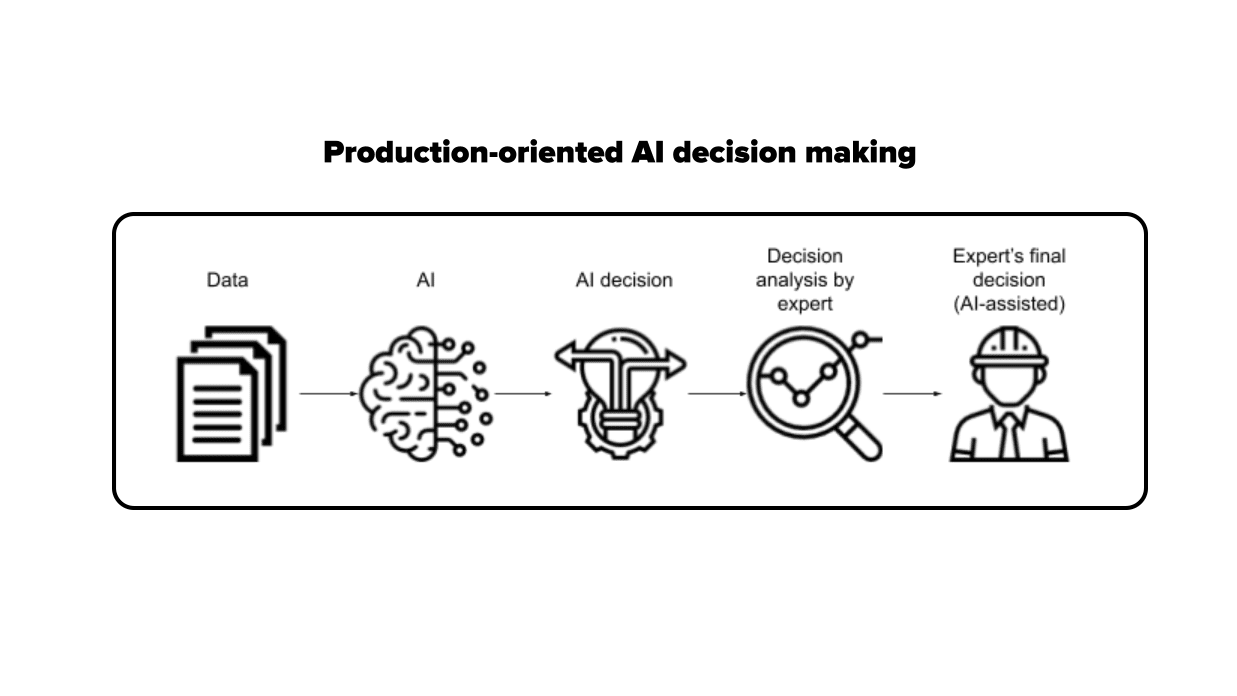
In industries like manufacturing or e-commerce, where there is a constant stream of data, AI can synthesize this information into actionable insights. By combining data from sales, inventory, customer feedback, and external factors, AI can provide real-time reports that empower managers to make data-driven decisions quickly and confidently.
Consider a case where AI-powered systems in Capella’s operations helped streamline decision-making. With the volume of client data we process, having AI assist in identifying patterns and anomalies in data has been invaluable. This allows us to act quickly and allocate resources to areas that need attention, improving our operational flexibility.
3. Proactive Risk Management
AI also has a crucial role in mitigating risks. Operational risk management has traditionally been reactive—companies identify issues only after they’ve caused damage. AI shifts that paradigm by enabling proactive risk mitigation.
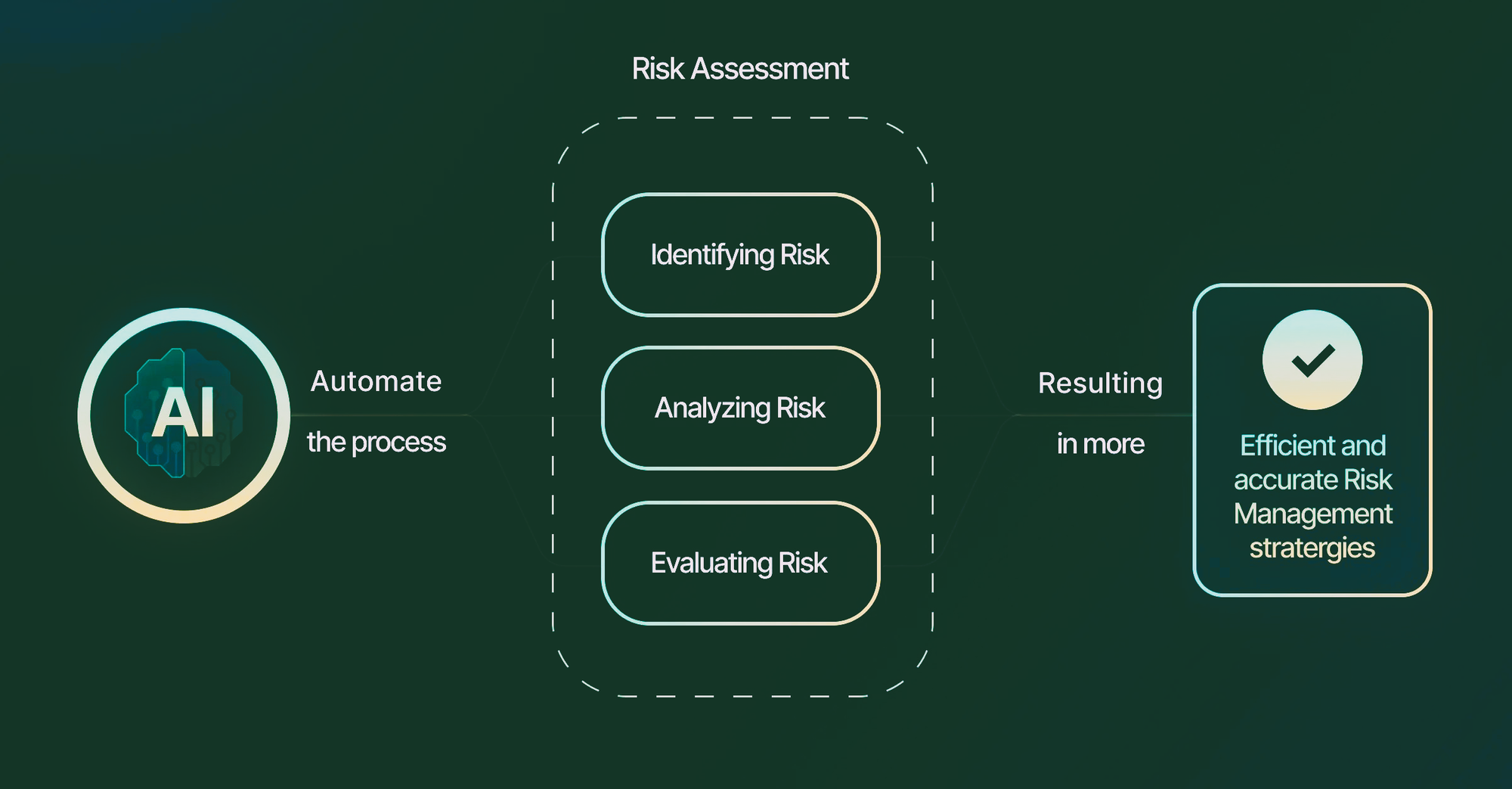
For example, predictive maintenance, powered by AI, can monitor the health of equipment and flag potential breakdowns before they happen. This is particularly valuable in industries like manufacturing or heavy equipment.
Example: A company in the energy sectoremploys AI for predictive maintenance of their power plants. Using machine learning algorithms, they monitor equipment performance in real time and predict when a particular machine will need maintenance, reducing downtime by 40% and saving millions annually.
AI Use Cases and Real-World Examples

So, how can AI be applied practically in your operations? Let’s explore a few use cases:
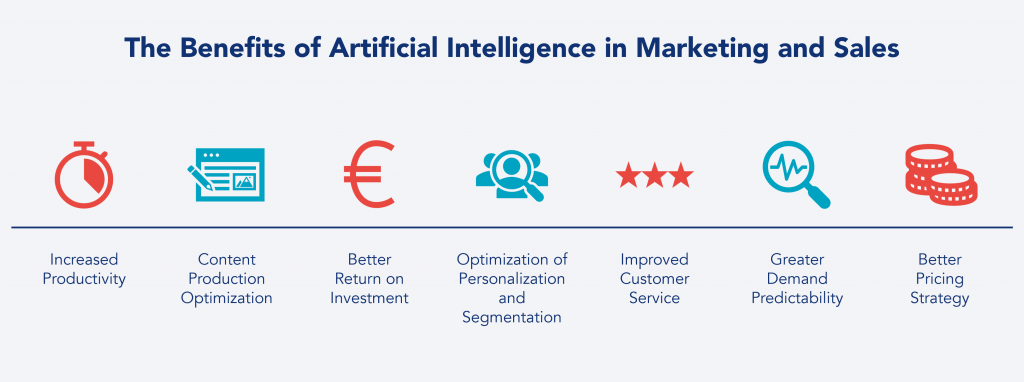
1. Sales and Marketing Optimization
AI can optimize sales funnels and marketing strategies by analyzing customer behavior and predicting future buying patterns. It can segment customers with precision and suggest personalized marketing campaigns based on their interests and past interactions with your brand.
Example: An E-commerce giant uses AI to analyze purchasing patterns and suggest personalized discounts and product recommendations to customers. This AI-driven approach led to a 25% increase in their conversion rates.

2. AI-Powered Fraud Detection
Fraud is a significant concern in many industries, from finance to retail. AI can help businesses detect fraudulent activity faster by analyzing transaction patterns and identifying irregularities in real time.
Example: A financial services company, employs AI to monitor transaction patterns and flag any anomalies that could indicate fraud. Since implementing AI-driven fraud detection, they’ve reduced fraudulent transactions by 60%.

Breaking Down Barriers to AI Adoption

Despite all the potential, the reality is that many companies face barriers when adopting AI. Whether it’s the upfront cost, lack of expertise, or fear of disruption, the challenges can seem daunting.
Here’s the thing: AI doesn’t need to be implemented all at once. Start small. Identify a single area in your operations where AI can have the most impact. It could be automating a manual process or improving decision-making with predictive analytics. Once you see results, you can expand its application.
Steps to Implement AI in Your Operations:
- Identify key areas of improvement (e.g., customer service, supply chain, decision-making).
- Collect and clean data—AI relies on high-quality data to provide accurate insights.
- Choose the right tools—You don’t need to reinvent the wheel. Use existing AI tools or platforms that can be easily integrated into your existing systems.
- Train your team—Get your team on board and ensure they understand how to use AI to improve operations.
- Measure and iterate—Track the performance of AI implementations and refine them for better results.
The Future of Operational Growth with AI
The move from operational bottlenecks to breakthroughs is not just about technology. It's about how businesses adapt to a rapidly changing world. AI is the tool that can propel your company forward—by automating processes, improving decision-making, and eliminating risks. The companies that will thrive are the ones that can harness AI to break through their current limitations and unlock new levels of efficiency.
AI is here to stay. It’s no longer a futuristic
concept. It’s a present-day solution to age-old problems. And for those who are willing to embrace it, the potential for operational growth is limitless.
By taking a methodical, intelligent approach to integrating AI into your operations, you’re not just solving problems. You’re setting the stage for long-term success.
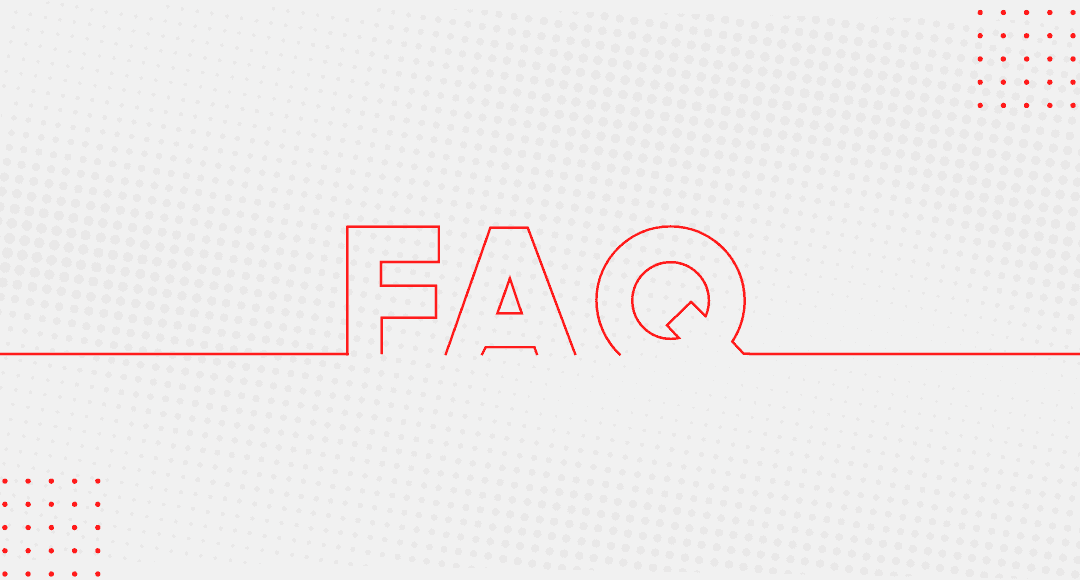
1. How can AI identify operational bottlenecks that may not be visible to human operators?
AI can analyze massive amounts of operational data to detect inefficiencies that may be hidden from human operators. Using techniques like anomaly detection and predictive analytics, AI identifies patterns and deviations that are linked to bottlenecks. For instance, AI might recognize that certain processes always slow down at a particular time of day due to system overuse, allowing companies to proactively adjust resource allocation or workload distribution.
2. What types of businesses can benefit from AI for operational growth?
AI can benefit virtually any business that deals with repetitive tasks, decision-making challenges, resource allocation, or risk management. Industries such as manufacturing, logistics, retail, finance, and healthcare can particularly benefit from AI solutions because they handle large volumes of data and complex workflows, which AI excels at optimizing. Even small businesses can leverage AI for customer support automation and predictive insights without requiring large-scale implementations.
3. What is the difference between traditional automation and AI-powered automation in operations?
Traditional automation follows pre-set rules to perform tasks, which makes it effective for simple, repetitive activities that require no variability. AI-powered automation, on the other hand, learns and adapts to new data. This adaptability allows it to handle more complex processes, make decisions, and continuously improve outcomes. For instance, a traditional rule-based system might route a customer support request to a human agent, while an AI system can determine the complexity of the request and answer it independently or escalate it appropriately.
4. How can AI support more effective decision-making in a company’s operations?
AI supports effective decision-making by providing decision-makers with real-time data analysis, predictive models, and actionable insights. For example, AI-driven dashboards aggregate data from multiple sources and help executives spot emerging trends, anomalies, or bottlenecks before they become problems. Instead of making decisions based solely on historical data or gut instinct, managers can rely on AI to understand future possibilities and allocate resources more strategically.
5. What are some specific AI tools that companies can use for operational growth?
Several AI tools can be used for operational growth, depending on the specific use case. Robotic Process Automation (RPA) tools like UiPath and Blue Prism are helpful for automating repetitive tasks. Tools like Tableau and Power BI, when integrated with machine learning models, help provide deeper insights for decision-making. For predictive maintenance, solutions like IBM Watson IoT or Azure Machine Learning are effective. Natural Language Processing (NLP)-based AI tools such as Drift and ChatGPT can be used to handle customer interactions seamlessly.
6. Can AI be implemented in stages to avoid disruption to current workflows?
Yes, AI can and should be implemented in stages. Companies often start with pilot projects to validate the impact of AI on a small scale before expanding it across the organization. It might begin by automating a single, repetitive process or integrating AI into an existing analytics tool to provide more advanced insights. Gradual implementation helps avoid the shock of large-scale changes and allows employees to adapt comfortably, thus ensuring better adoption rates and minimizing disruption.
7. How does predictive maintenance reduce operational downtime?
Predictive maintenance leverages AI algorithms to analyze equipment data (such as vibration, temperature, and operational hours) and predict potential failures before they occur. This means that instead of relying on scheduled maintenance—which may lead to unnecessary maintenance or unexpected breakdowns—companies can perform maintenance when needed, based on real-time insights. This proactive approach reduces downtime significantly, saving both time and money.
8. What are the typical challenges faced during AI adoption in operations?
Typical challenges during AI adoption include high upfront costs, lack of expertise in AI technologies, data quality issues, and employee resistance. High costs can be mitigated by starting with targeted projects that demonstrate ROI. Lack of expertise can be managed through partnerships with AI vendors or through internal training programs. Data quality issues often need careful handling by creating proper data cleaning mechanisms, and employee resistance can be addressed by involving staff in the AI process, thereby allaying fears of job displacement.
9. How does AI help improve resource allocation within a company?
AI can optimize resource allocation by predicting demand, identifying inefficiencies, and suggesting the best ways to allocate human, financial, and physical resources. For instance, in retail, AI can predict which products will be in high demand during certain seasons, enabling companies to allocate inventory more efficiently. In project management, AI tools can assign tasks to employees based on their workload, skill set, and availability, thus optimizing team efficiency and reducing the likelihood of bottlenecks.
10. What role does AI play in minimizing operational risks?
AI helps minimize operational risks by continuously monitoring operational processes, detecting anomalies, and providing predictive insights. For example, AI-driven fraud detection systems analyze transactions in real-time and flag suspicious activity, significantly reducing financial risks. In manufacturing, AI models monitor production lines for unusual patterns that might indicate potential breakdowns, allowing companies to take corrective measures before issues escalate. This proactive risk management can lead to safer, more reliable operations.
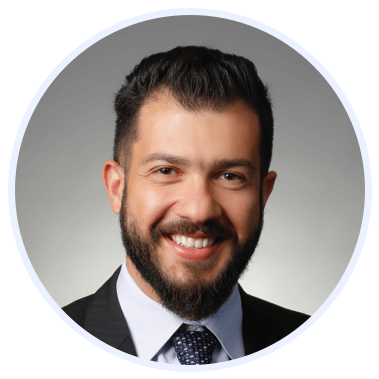
Rasheed Rabata
Is a solution and ROI-driven CTO, consultant, and system integrator with experience in deploying data integrations, Data Hubs, Master Data Management, Data Quality, and Data Warehousing solutions. He has a passion for solving complex data problems. His career experience showcases his drive to deliver software and timely solutions for business needs.