
We’re standing on the precipice of a new era. The future is here, and it’s powered by Artificial Intelligence (AI). Yet, for many companies, the question isn’t about whether AI will dominate their industry—it’s whether their business is prepared for it. How can they integrate AI effectively? How can they benefit from it for lasting competitive advantage?
In the next few years, AI will reshape industries in ways we can’t fully predict. But that’s not an excuse to sit on the sidelines. The real question is: How can you make sure your business is not just surviving, but thriving in this AI-driven world?
Let’s face it: Many businesses still treat AI like a buzzword. It’s easy to see why—AI is complex. It requires a combination of technical infrastructure, clear strategic thinking, and, above all, a mindset shift. It’s not just about adding shiny tech for the sake of innovation. It’s about understanding how to integrate AI thoughtfully to solve real, pressing problems and create sustainable value.
Here’s a quick rundown to help you assess your business’ readiness for AI integration. Whether you’re looking to optimize operations, enhance customer experiences, or revolutionize decision-making, this checklist will guide you through the essential steps. Let's jump in.
1. Is Your Data Foundation Solid?
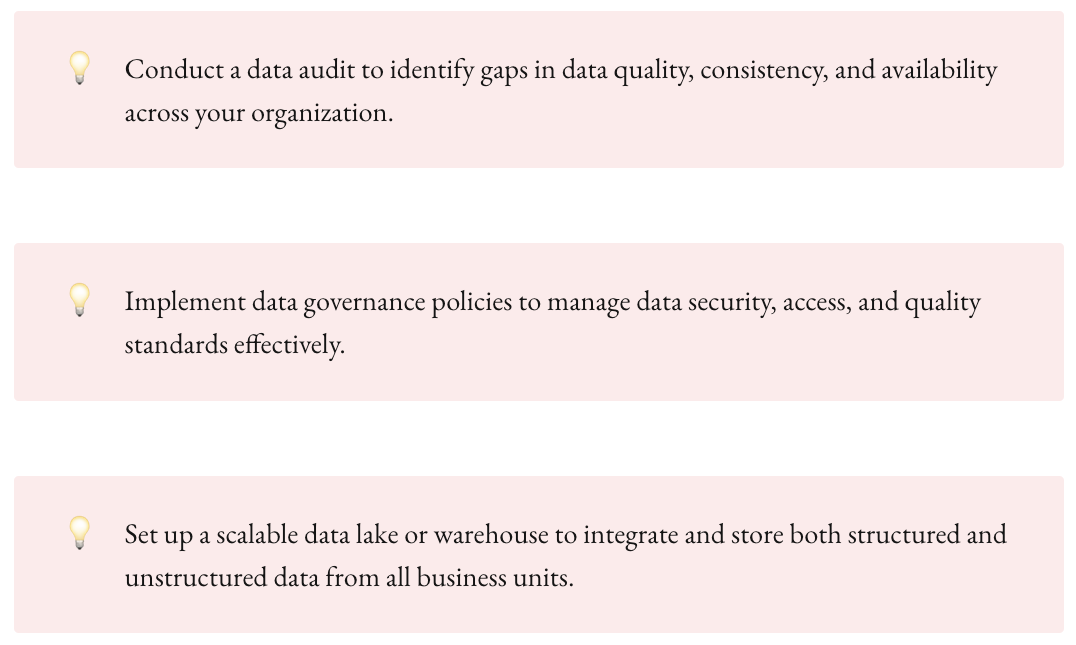
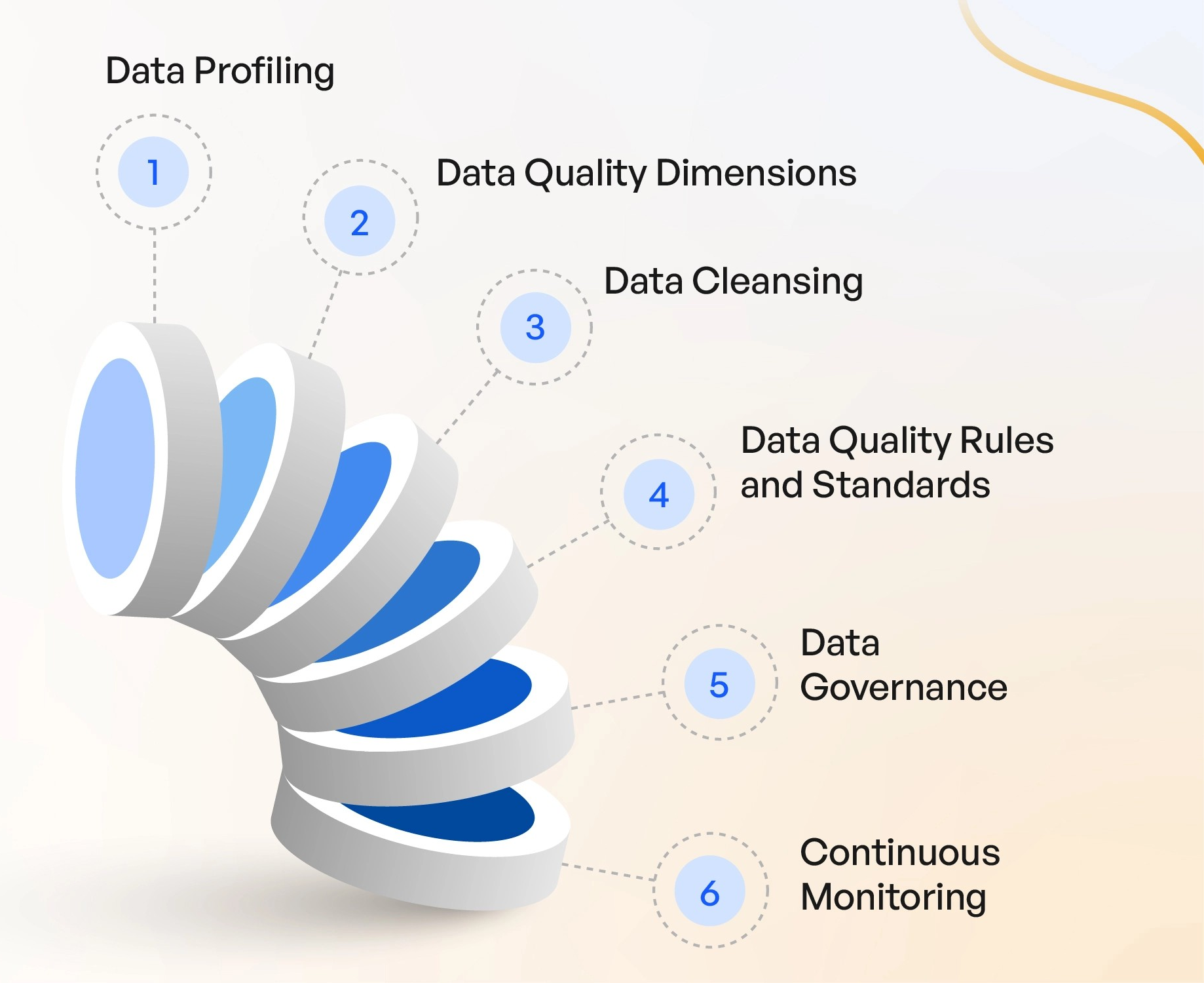
AI thrives on data. But not just any data—clean, structured, and high-quality data. Without a solid data foundation, AI implementations are a shot in the dark. This means ensuring your data is not only available but also accessible, consistent, and accurate.
Here are a few key things to ask yourself:
- Data Quality: Are your data sources reliable? Is your data accurate, complete, and free of biases that could skew AI models?
- Data Availability: Is your data siloed, or do you have systems in place that allow for seamless integration and aggregation?
- Data Governance: Are there clear policies and protocols for data access, security, and privacy?
AI systems need vast amounts of high-quality data to function. But garbage data leads to garbage outputs. You must invest in robust data management practices. This doesn’t just mean cleaning up your current datasets—it also involves setting up systems that can capture fresh, relevant data continuously.
Tip: Implement a data lake architecture. This allows you to aggregate and store structured and unstructured data from multiple sources, making it easier to prepare for future AI initiatives.
Real-Life Example
Take a manufacturing company using AI to predict machine failures. If the data on past failures is incomplete or inaccurate, the AI model won’t be able to identify patterns correctly, leading to unreliable predictions and unnecessary downtime. Data is the lifeblood of AI, and without clean, organized data, your AI efforts will struggle.
2. Are You Empowering the Right Teams?
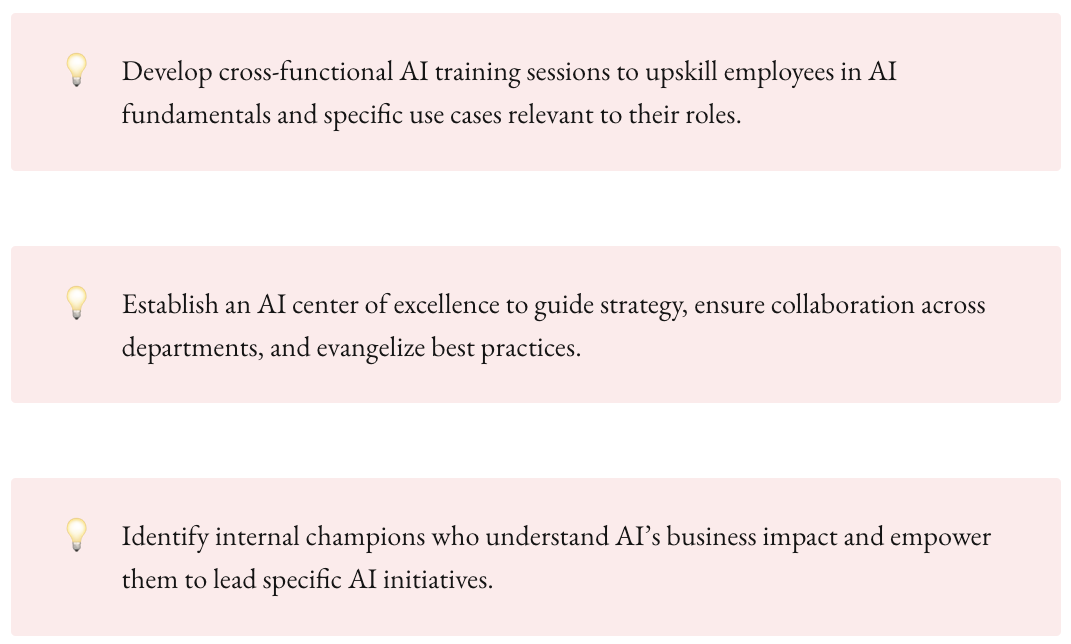

Successful AI integration is about more than just technology. It’s about the people driving the change. Who are the internal champions of AI? Do you have a team in place that understands the technical, strategic, and ethical implications of AI?
- AI Expertise: Do you have data scientists or AI specialists on staff, or are you working with trusted partners who can guide your initiatives?
- Cross-Functional Collaboration: Have you broken down silos between departments like IT, marketing, customer service, and operations? AI needs input from all areas of the business to be effective.
- AI Leadership: Is there clear leadership, someone who understands both AI and business strategy, driving AI initiatives?
The technology itself isn’t the bottleneck. It’s getting the right talent and vision in place. AI implementations often fail because they’re not backed by an empowered, well-trained team that understands the business needs. Investing in AI training for your employees can go a long way toward building a culture that embraces innovation.
Tip: Create an AI center of excellence. This cross-functional team can help ensure AI efforts are aligned with broader business goals and are driving real value.
Real-Life Example
Consider a global financial services firm implementing AI for customer service chatbots. The lack of internal collaboration between the AI development team and the customer service team led to mismatched priorities. The chatbot wasn’t delivering on its potential because it didn’t align with the real-world needs of customers. By fostering collaboration, the company was able to align its chatbot AI with actual customer pain points, boosting both efficiency and satisfaction.
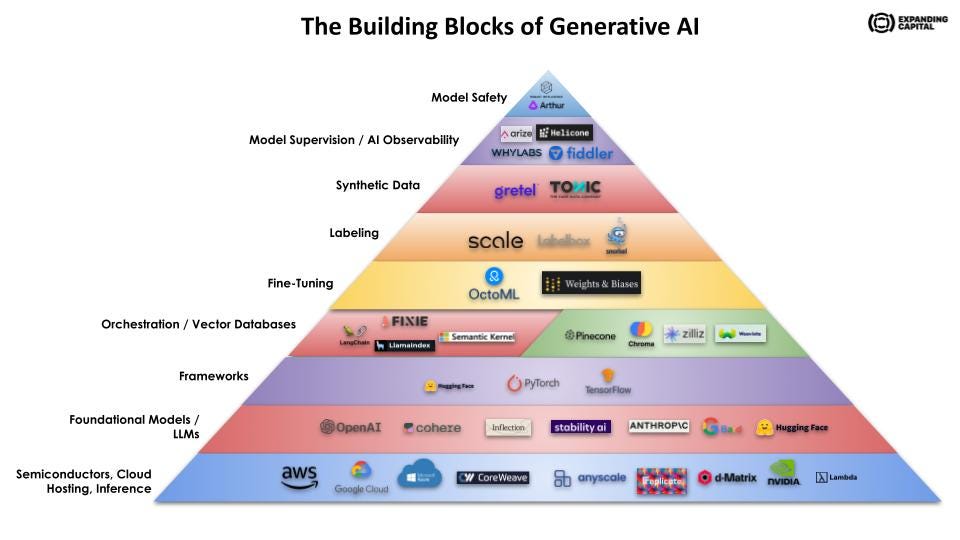
3. Do You Have the Right Technology Infrastructure?
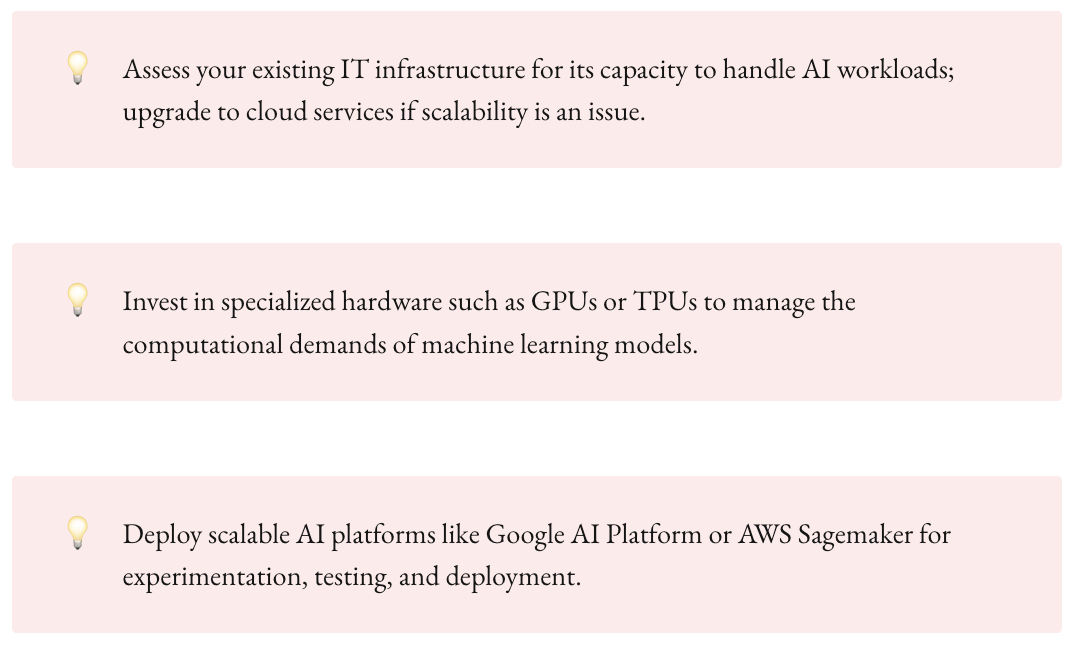
AI demands a specific set of technological requirements. Beyond just your basic IT stack, are you investing in the infrastructure needed to support AI at scale?
- Compute Power: AI models, especially deep learning models, require significant computational resources. Are you using on-premise hardware, or are you tapping into cloud solutions like AWS, Google Cloud, or Microsoft Azure?
- Data Storage: Does your storage solution scale with the increasing volume and complexity of data? Is it designed for fast access to large datasets?
- AI Frameworks & Tools: Are you equipped with the necessary tools, such as TensorFlow, PyTorch, or cloud-based AI services, to build and deploy AI models?
The infrastructure you build must scale with your business needs. This might involve upgrading to cloud infrastructure, adopting specialized hardware like GPUs, or using machine learning platforms to manage and deploy models more effectively.
Tip: Consider cloud-based AI services like AWS Sagemaker or Google AI Platform. These tools provide scalable AI solutions with a pay-as-you-go model, so you can experiment without committing to hefty upfront costs.
Real-Life Example
A large retailer wanted to implement a recommendation system to improve online sales. However, their on-premise servers couldn’t handle the compute demands of real-time recommendation models. By shifting to cloud-based infrastructure, the retailer not only improved performance but also reduced the costs associated with maintaining an internal server farm.

4. Do You Have a Clear AI Strategy?
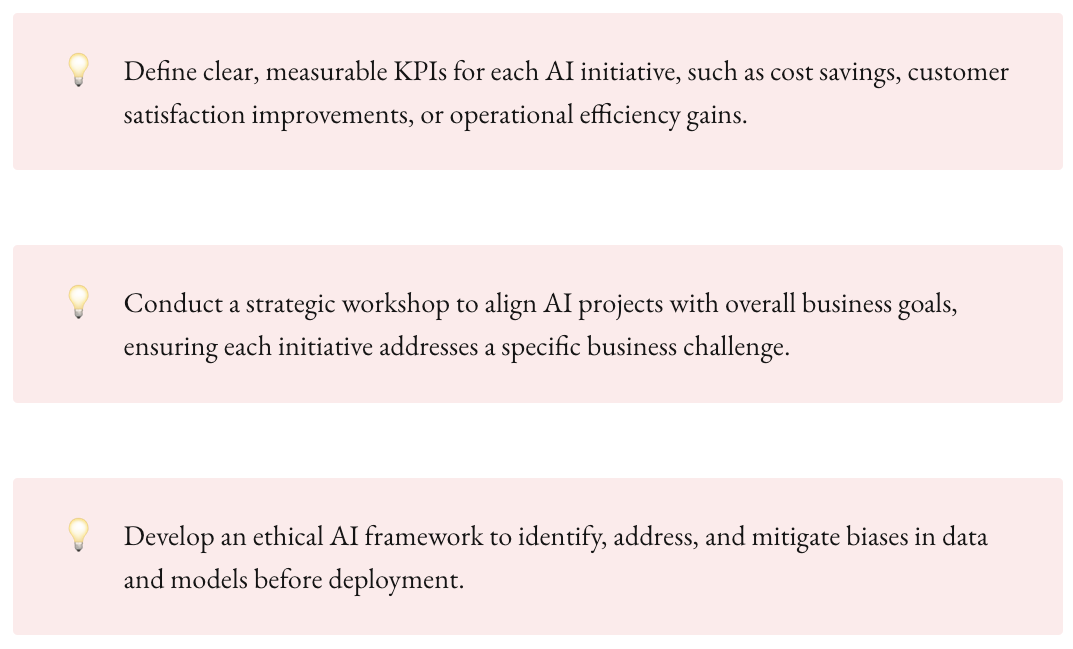
AI isn’t a plug-and-play solution. It requires thoughtful planning and alignment with business goals. Without a clear strategy, you risk pursuing AI projects that lack focus or real business impact.
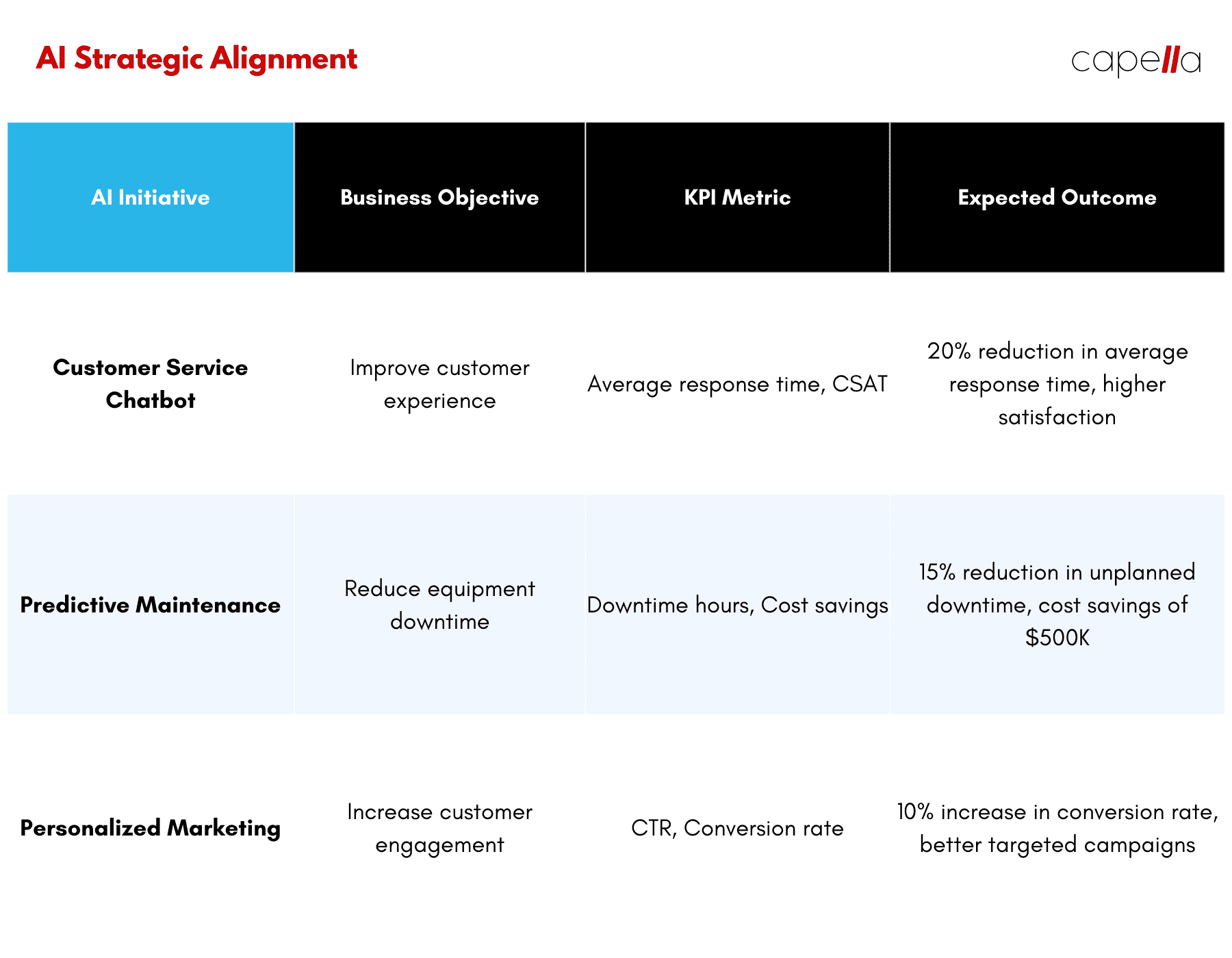
Consider these questions:
- Strategic Alignment: Have you clearly defined the problem AI will solve for your business? Is the AI initiative aligned with your broader corporate strategy?
- Measurable Outcomes: Do you have KPIs and metrics in place to track AI performance? Are you focused on tangible results like cost savings, revenue growth, or operational efficiency?
- Ethical Considerations: Are you addressing the ethical implications of AI, like bias, transparency, and accountability?
Having a clear AI strategy means aligning AI initiatives with measurable business objectives. It’s not just about adopting the latest technology—it’s about choosing the right problems to solve with AI and defining success in concrete terms.
Tip: Map out specific AI initiatives on a timeline with clear milestones. Use a phased approach to allow for testing, iteration, and refinement.
Real-Life Example
An automotive company set out to implement AI for autonomous vehicles. However, they initially lacked a clear roadmap and focused too much on the technology without understanding the market need. Once they realigned their strategy with customer pain points, such as safety and efficiency, the project gained momentum. They shifted to using AI for predictive maintenance and route optimization, which delivered measurable business value while still progressing toward their long-term vision of autonomy.
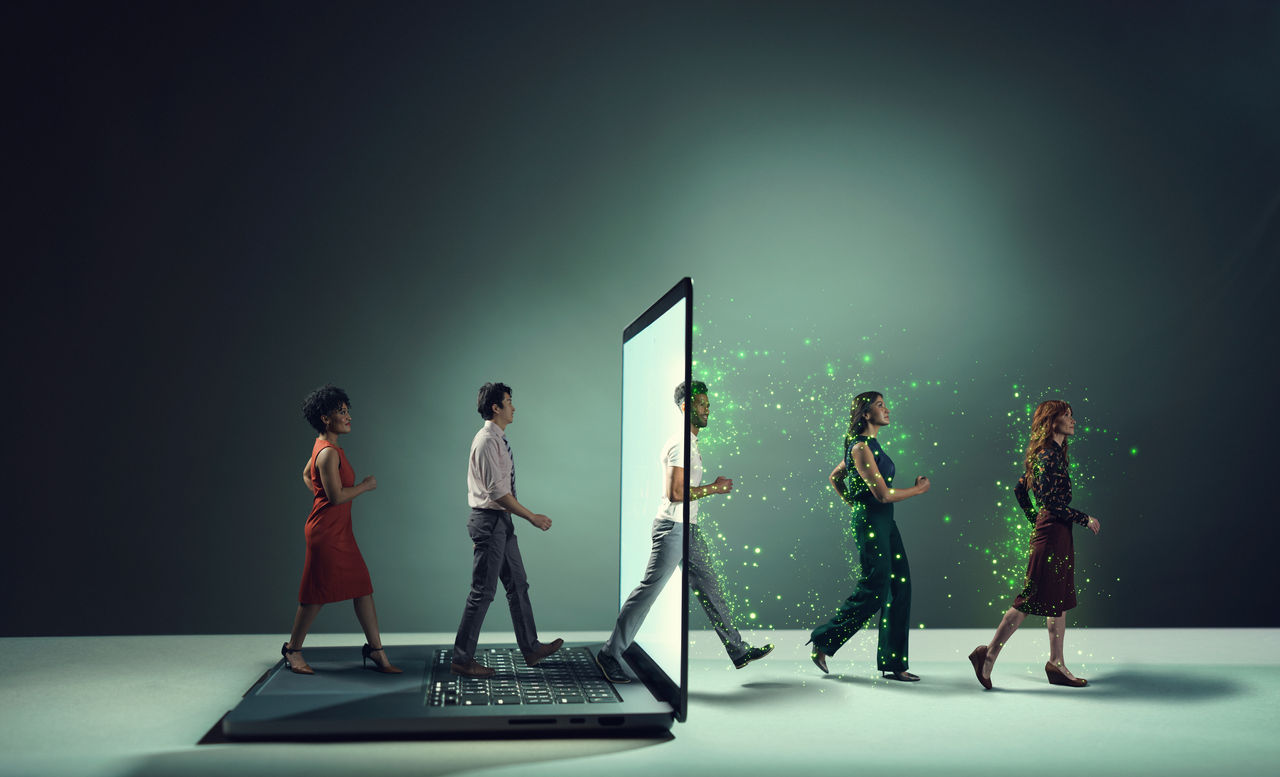
5. Is AI Adoption Part of Your Company Culture?

AI is more than just a technology initiative—it’s a cultural shift. For AI to succeed in your organization, it must be embraced by your employees, especially those at the operational level. This isn’t just about convincing your leadership team; it’s about empowering everyone in the organization to view AI as an enabler of their work.

- Employee Buy-In: Do your employees understand how AI will impact their roles and the company’s long-term success? Are they excited about the potential?
- Change Management: Are you prepared to handle the organizational change that comes with AI adoption? Have you communicated a clear vision and provided proper training?
- Continuous Learning: Does your company invest in ongoing education for employees to stay up-to-date with AI developments?
An AI-driven culture isn’t built overnight. It requires clear communication, proper training, and fostering a mindset of continuous improvement. Ensure that your team sees AI not as a threat but as a powerful tool that amplifies their skills.
Tip: Implement a change management program to guide employees through the AI adoption process. Offer training sessions, workshops, and opportunities for feedback to ensure a smooth transition.
Real-Life Example
A logistics company introduced AI-powered route optimization software. Initially, drivers were skeptical about the system, fearing it would replace their jobs. By involving drivers in the AI implementation process—showing them how it would make their jobs easier and more efficient—the company gained buy-in and improved operational outcomes.
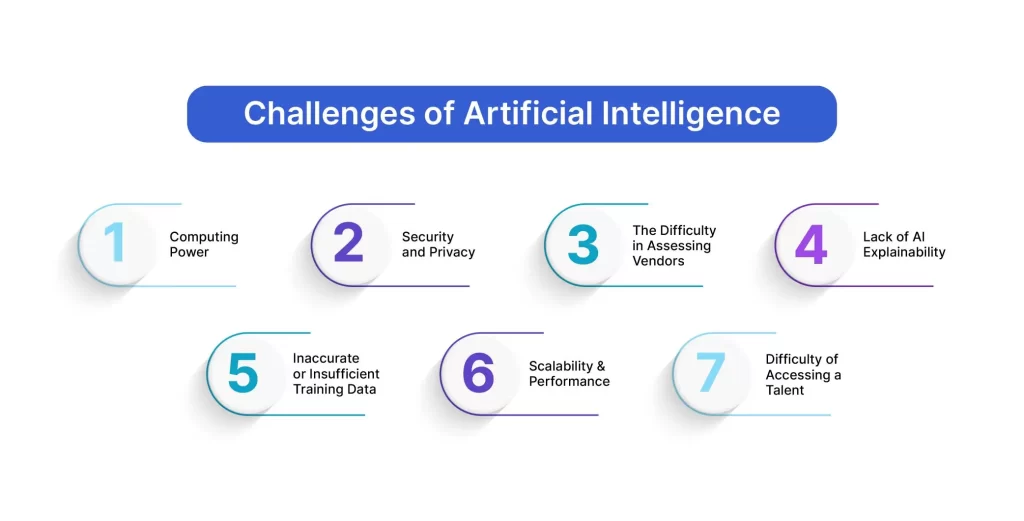
6. Is Your AI Initiative Scalable and Sustainable?
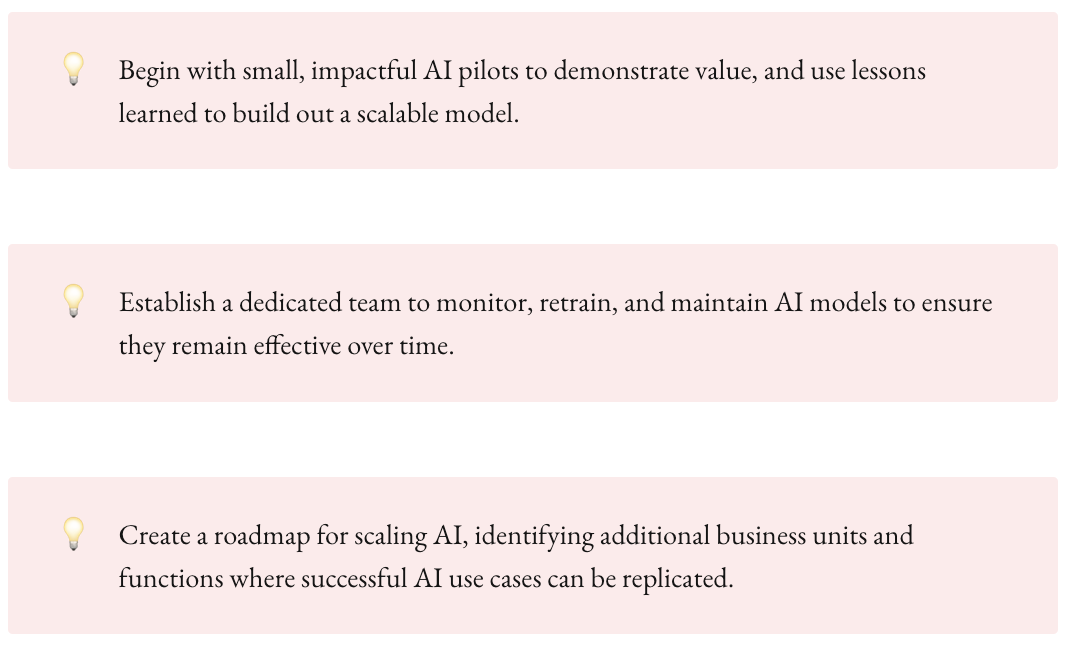
Finally, AI integration needs to be scalable. It’s not enough to implement one AI tool or run one AI experiment; the real potential lies in scaling AI across the organization over time.
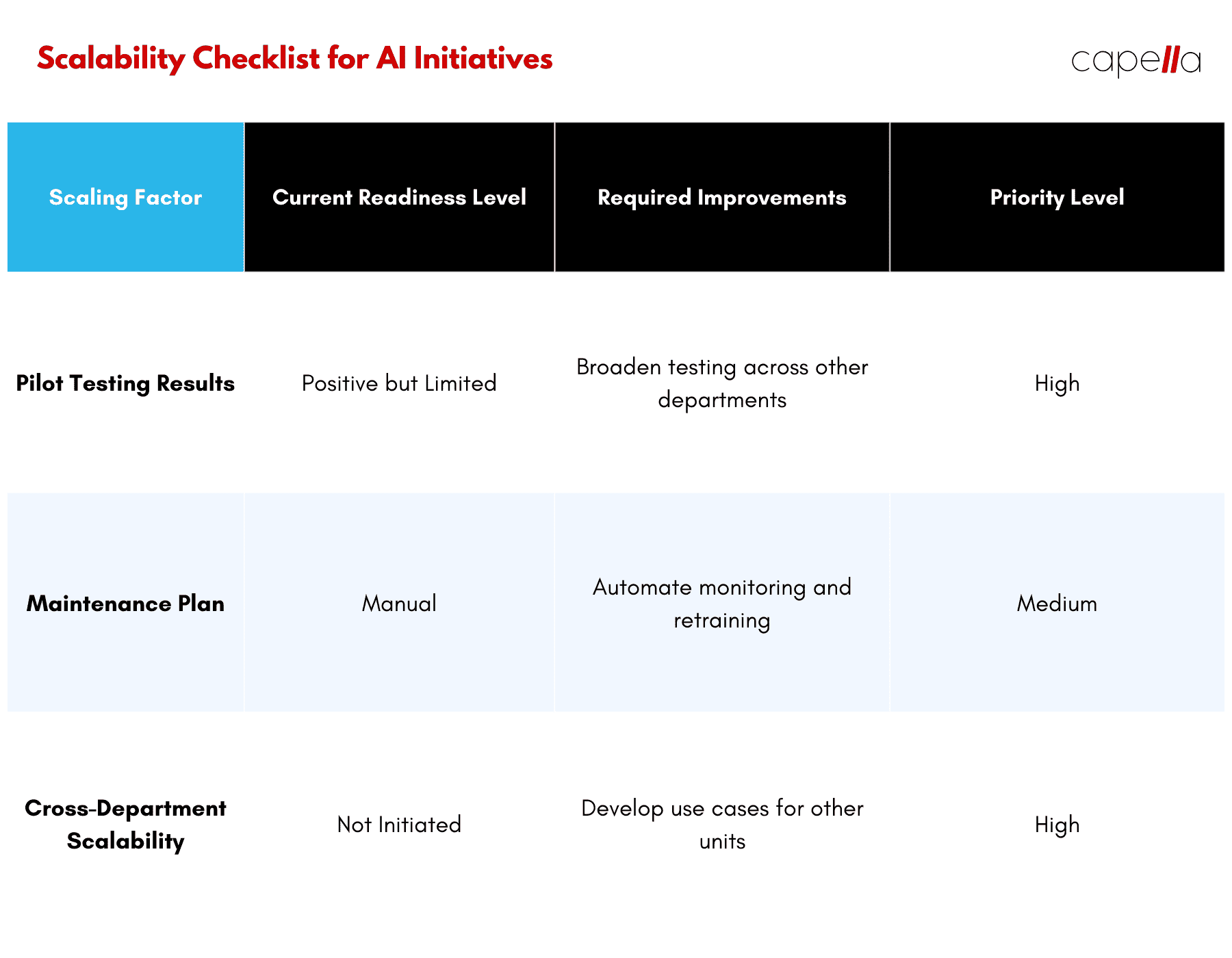
- Scalability: Can your AI solutions scale with your business? Are they adaptable to different departments, teams, or use cases?
- Sustainability: Have you built long-term plans for maintaining AI systems, including monitoring, retraining, and updating models?
- Iterative Improvement: Are you adopting a continuous improvement approach to AI, where models are constantly refined based on new data and feedback?
Scalability is one of the most common bottlenecks in AI integration. Early successes often don’t translate to broader success because systems aren’t built to scale. This is where ongoing investment in technology and talent becomes essential.
Tip: Create a roadmap for scaling AI across multiple use cases. Start small, test, and build upon successes to expand your AI footprint gradually.
The future of business is AI, but only for those who are ready for it. AI isn’t just a tool; it’s a new way of doing business. Getting AI integration right takes more than just buying the latest technology. It takes a strategic approach to data, people, processes, and infrastructure.
As you assess your business’ readiness, remember: AI is not a destination; it’s a journey. Prepare now, and you can ensure your company doesn’t just survive the future—it thrives in it.
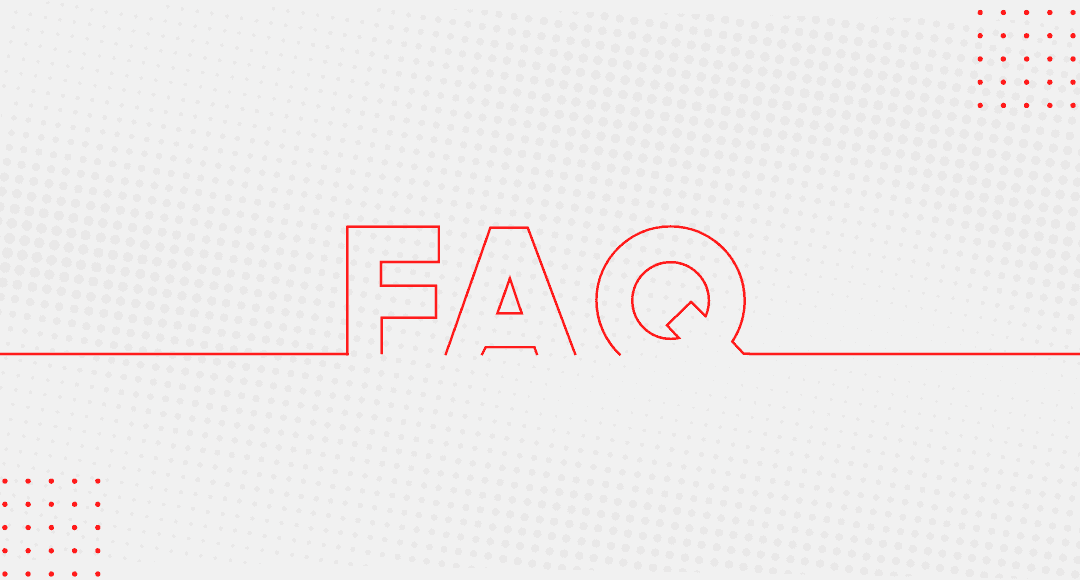
1. Why is data quality so crucial for AI integration?
Data quality is the foundation of successful AI initiatives. If the data you feed into an AI system is incomplete, inconsistent, or biased, the outputs will reflect those flaws. For example, poor data quality can lead to incorrect predictions, reduced model accuracy, or, worse, biased decision-making. High-quality data ensures that AI models learn from accurate, relevant information, thereby generating actionable insights that drive value. To prepare for AI integration, businesses need to clean, normalize, and enrich their data continuously to maintain its integrity and usefulness.
2. What kind of infrastructure do I need to implement AI effectively?
AI requires a robust infrastructure that includes high compute power, ample data storage, and suitable tools and frameworks. High compute power, often in the form of GPUs or cloud services, is needed to train complex machine learning models. Data storage should be scalable to handle growing volumes of data, both structured and unstructured. Tools like TensorFlow or cloud-based platforms like AWS Sagemaker are essential for model development and deployment. Having this infrastructure in place ensures that your AI systems can handle the computational and data challenges they are likely to face.
3. How do I align AI projects with my business strategy?
AI projects should always align with well-defined business objectives to deliver meaningful impact. Start by identifying key business challenges, such as reducing operational inefficiencies, improving customer experience, or increasing revenue. Next, determine how AI can contribute to these goals. For example, if operational inefficiency is an issue, consider implementing AI for predictive maintenance. Align each AI initiative with clear KPIs, such as cost reduction or improved customer satisfaction, so the value and progress of the initiative can be tracked effectively.
4. How do I build an AI-savvy workforce?
Building an AI-savvy workforce requires investment in training, hiring, and internal collaboration. Start by providing foundational AI training to employees across all departments, not just IT. This helps in building general awareness of AI’s capabilities and limitations. Next, hire specialized talent, such as data scientists, machine learning engineers, and AI strategists. Fostering cross-functional collaboration is also key; business and technical teams must work together to design AI solutions that address real business needs, rather than operating in silos.
5. What are the ethical considerations for AI implementation?
Ethical considerations in AI include bias, transparency, and accountability. AI models are only as unbiased as the data they're trained on; thus, it’s important to proactively identify and mitigate biases during the data collection and model training phases. Transparency is about making AI processes understandable to stakeholders; black-box models should be avoided where interpretability is crucial. Accountability means having policies in place to ensure that AI models are used responsibly, with mechanisms to address any issues or unintended consequences.
6. How do I ensure employee buy-in for AI initiatives?
Employee buy-in is essential for the success of AI integration. This begins with education—employees need to understand how AI will positively impact their work. Transparency about the goals and expected outcomes helps demystify AI and makes employees feel involved in the transformation. Creating a change management program that includes frequent workshops, Q&A sessions, and hands-on training can further bridge the gap. Also, involve employees early in pilot projects, showing how AI can assist rather than replace their roles.
7. What are some practical examples of AI use cases across industries?
AI use cases are diverse across industries. In manufacturing, AI can predict machine failures through predictive maintenance, which reduces downtime. In retail, AI can drive personalized marketing by analyzing customer behavior to recommend relevant products. In healthcare, AI can help analyze medical images to identify potential health issues earlier than a human expert might. Understanding specific use cases within your industry can help you better identify areas where AI can deliver immediate value.
8. How do I determine if my AI initiatives are scalable?
To determine if AI initiatives are scalable, evaluate the infrastructure, data, and processes involved. Scalability means your AI system should be able to handle increased data volumes and additional use cases without significant rework. Start by running small-scale pilot projects; analyze their success and use the results to inform broader implementations. The technology stack must be flexible, ideally working with cloud solutions that can scale up on-demand. Also, standardize your approach to data management and model retraining to ensure AI remains effective over time.
9. What is the best way to pilot AI in a large organization?
The best way to pilot AI in a large organization is to start with a small, low-risk use case that has measurable outcomes. Choose a project that can deliver quick wins to demonstrate value, such as automating repetitive tasks or enhancing an existing business process with data-driven insights. Set up clear success metrics, gather cross-functional stakeholders, and ensure leadership visibility. Pilots are valuable because they allow experimentation without large-scale commitment, enabling teams to learn what works before scaling across the organization.
10. How do I measure the success of AI initiatives?
Measuring the success of AI initiatives requires setting clear KPIs aligned with business objectives. This could include financial metrics like cost savings or increased revenue, operational metrics like reduced downtime or improved productivity, and customer metrics like satisfaction scores or engagement rates. Regularly compare these KPIs before and after AI implementation to gauge impact. Beyond quantitative metrics, qualitative feedback from users and employees interacting with AI can provide insights into areas for improvement or additional value that may not be immediately apparent in the numbers.
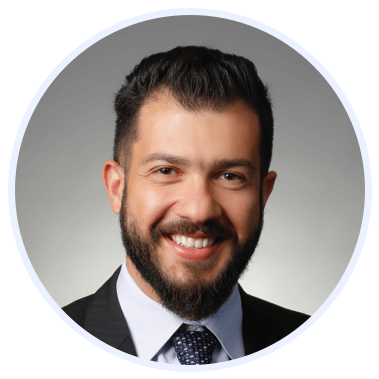
Rasheed Rabata
Is a solution and ROI-driven CTO, consultant, and system integrator with experience in deploying data integrations, Data Hubs, Master Data Management, Data Quality, and Data Warehousing solutions. He has a passion for solving complex data problems. His career experience showcases his drive to deliver software and timely solutions for business needs.