
The field of human resources (HR) is rapidly evolving. HR professionals today face increasing demands to deliver strategic value in a dynamic, global business environment. At the same time, exciting new technologies like large language models (LLMs) are emerging that promise to transform business operations. In light of these trends, HR leaders should rethink their strategies and embrace AI-powered technologies like LLMs to enhance all areas of human resources, from talent acquisition to employee experience.

The Strategic Potential of HR

Forward-thinking organizations understand HR’s potential to drive business performance when aligned to company goals. As one executive commented in Deloitte’s 2022 Global Human Capital Trends report:
HR leaders who lean into being architects of the organization and stewards of the employee experience help unleash productivity, innovation, and growth.
Rather than focusing narrowly on administrative functions, today’s HR departments have a huge opportunity to:
- Attract, develop, and retain top, diverse talent
- Anticipate and develop skills for the future
- Promote a culture aligned to business objectives
- Design positive employee experiences
- Make data-driven decisions on the workforce
The challenge? Operational burdens often prevent HR from fulfilling this strategic mandate. LLMs present new possibilities for optimizing HR processes through automation and AI augmentation.
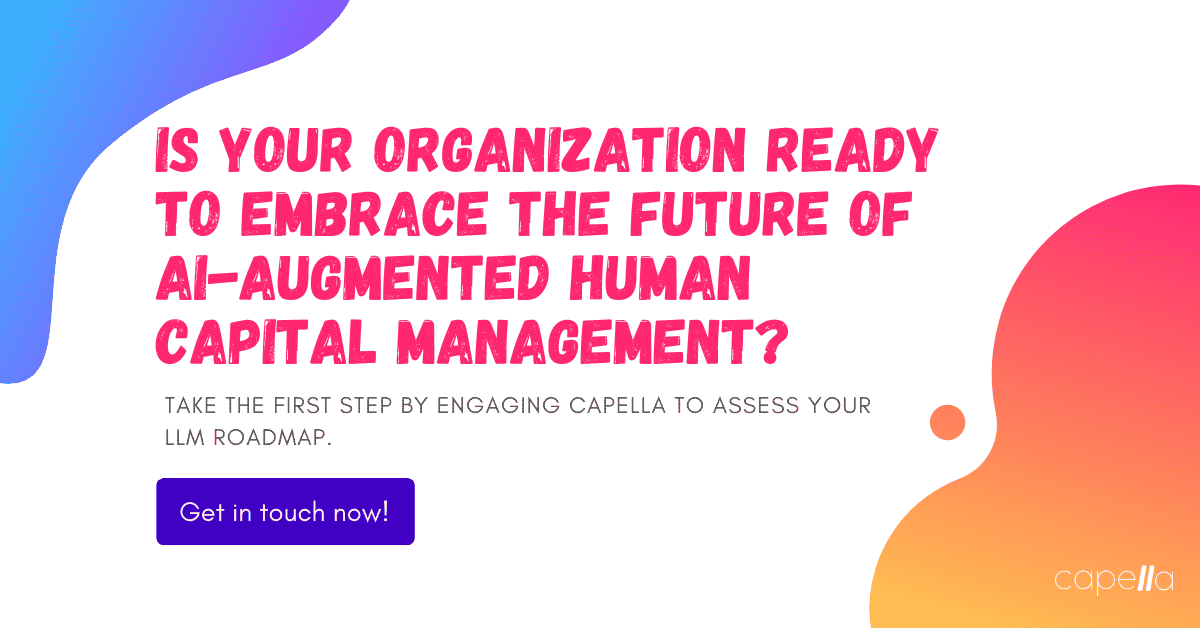

What Are LLMs and How Can They Enhance HR?

LLMs like OpenAI's GPT, Anthropic’s Claude, Google’s Bard, or Microsoft’s Sydney are AI systems trained on huge text datasets, allowing them to generate written content, answer questions, and more. These models hold exciting implications for streamlining business workflows.
Specific benefits of leveraging LLMs for human resources include:
Talent Acquisition
- Screen resumes and surface best candidates
- Automate interview scheduling
- Power chatbots for candidate Q&A
- Generate job postings optimized for each role
Learning & Development
- Deliver personalized recommendations for training based on employee goals, skills gaps
- Synthesize insights on trending skills to inform program development
- Source relevant content for curating training materials
Diversity, Equity & Inclusion (DEI)
- Audit existing policies and programs to identify DEI opportunities
- Research and draft inclusive policy guidelines on topics like family leave, remote work, etc.
- Analyze employee satisfaction survey results through a DEI lens
Total Rewards
- Monitor compensation benchmarking data and suggest optimization opportunities
- Review and enhance job architecture framework
- Provide competitive intelligence on benefits trends
Workforce Management
- Generate reports and dashboards with key workforce metrics
- Continuously synthesize internal data, external research to surface insights
- Forecast hiring needs based on growth plans
General HR Operations
- Respond to common employee queries through HR chatbots
- Populate knowledge base articles and FAQs
- Automate document generation for offers letters, contracts etc.
- Schedule meetings and interviews based on calendars and availability
The above reflects just some of the key ways LLMs can optimize HR processes from start to finish. Next we dive deeper into implementation considerations.

Putting LLMs to Work: Key Success Factors

While LLMs hold promise for augmenting talent management, simply plugging them in will not automatically generate value. Though powerful, these models have limitations requiring human oversight and governance.
Here are the best practices HR leaders should consider:

Get clear on goals and success metrics
HR cannot leverage AI simply for technology’s sake. Begin by identifying priority business goals, challenges LLMs can address, and how improved outcomes will be measured. Be specific – “enhancing recruitment” is too vague. Instead define targets like:
- Reduce recruitment cycle time from 100 to 60 days
- Double applicant conversion rate from 10% to 20%
- Lower recruiter admin work by 20% to increase strategic focus
Audit data readiness
LLMs are only as good as the data they are trained on. Review existing HR data quality – is relevant data captured consistently across systems? Identify gaps that need to be addressed through cleanup projects or process improvements before layering on AI.
Start small, scale thoughtfully
Pilot LLMs on targeted, high-impact use cases rather than attempting full-scale automation out of the gate. As comfort builds, scope can expand gradually across the function. Maintain realistic expectations during initial phases.
Monitor closely
Test LLM output rigorously before acting upon recommendations. Audit samples frequently for errors that could compromise data integrity or lead to biased outcomes. Watch closely for changes in model behavior over time.
Enable hybrid intelligence
LLMs best empower professionals when used collaboratively. HR should champion human-AI augmentation over full automation. Clearly define roles for technology versus people. For example, tap AI for pattern recognition from volumes of data, while keeping humans in the loop for judgment calls.
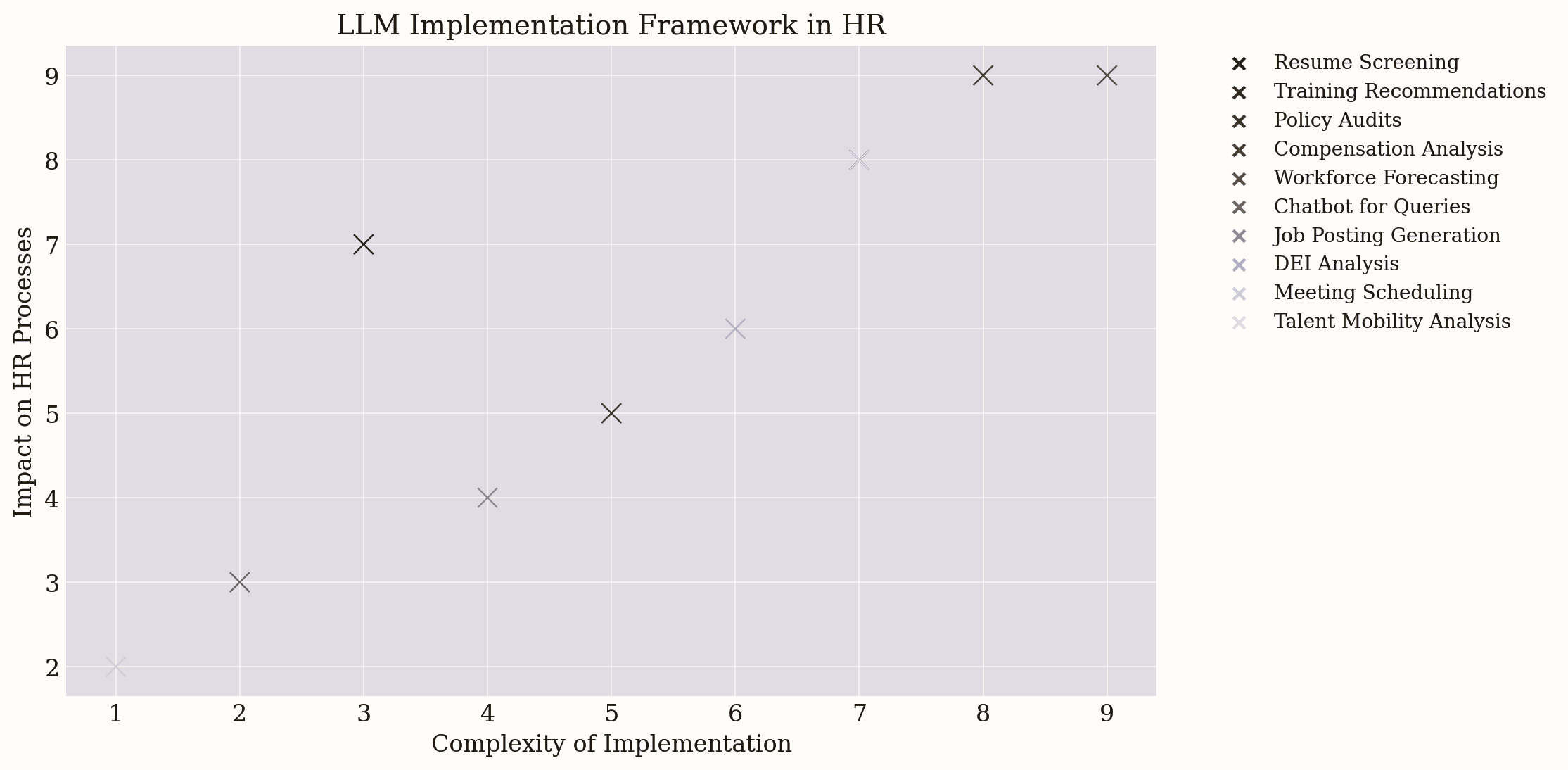
An HR Function Reimagined
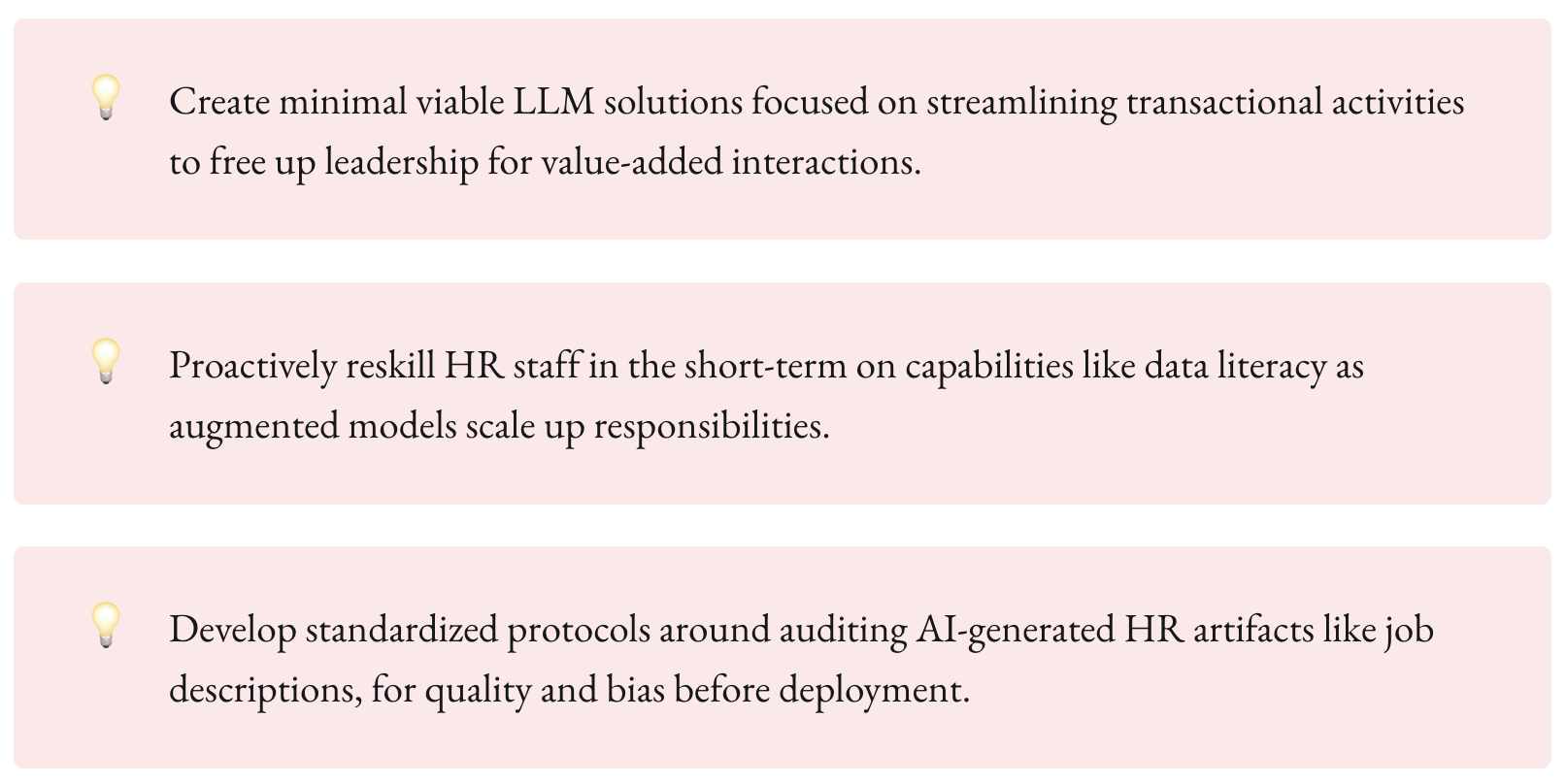
Below we illustrate how LLMs might transform HR across the employee lifecycle:
Attraction & Acquisition
LLMs mine internal mobility data, external wage benchmarking and published research on talent trends to forecast critical workforce needs. The tech identifies niche channels and creative messaging to attract in-demand candidates. It assesses applicants against established criteria, surfaces top talent for further review, and schedules interviews automatically based on best fit and availability.
Recruiters now focus purely on relationship-building, conducting values-based conversations with hires rather than admin tasks.
Onboarding
On their first day, new hires are greeted with a personalized email from their manager, outlining key activities for their ramp-up tailored to their role. Required forms and readings have been bundled into a simple e-packet. Based on calendars and location data, an optimal schedule has been created for 1-on-1s with key connections in their first month.
Learning
Employees receive prompt and relevant recommendations for training programs or microlearning content to enrich their roles. Course assignments adapt dynamically based on project exposure, growth areas and demonstrated strengths.
LLMs track the latest external developments in the company’s competitive environment, filtering enormous volumes of industry content to identify emergent trends and skilling gaps to inform L&D strategy.
Talent Mobility
Platforms harness unstructured data in performance evaluations, peer feedback and more to map high-potential talent and uncover development areas. System-generated insights feed personalized mobility recommendations matching demonstrated strengths to internal openings.
Employee Experience
Pulse surveys with tailored questions gauge engagement across the employee journey. Sentiment analysis reveals top drivers of attrition risk, while natural language generation automatically creates summaries of critical themes for managers.
Chatbots handle routine employee inquiries around benefits, time-off requests, company policies and more, enabling HR staff to resolve more complex issues.
Total Rewards
Algorithms ingest compensation benchmarking stats, performance data and attrition risks to evaluate pay equity across roles. The system surfaces areas of misalignment with internal parity or external market competitiveness for compensation team review.
Offboarding
Exit interviews are conducted by chatbot, with transcript analysis highlighting recurring themes around departure drivers. These feed into corporate culture initiatives and retention strategy.
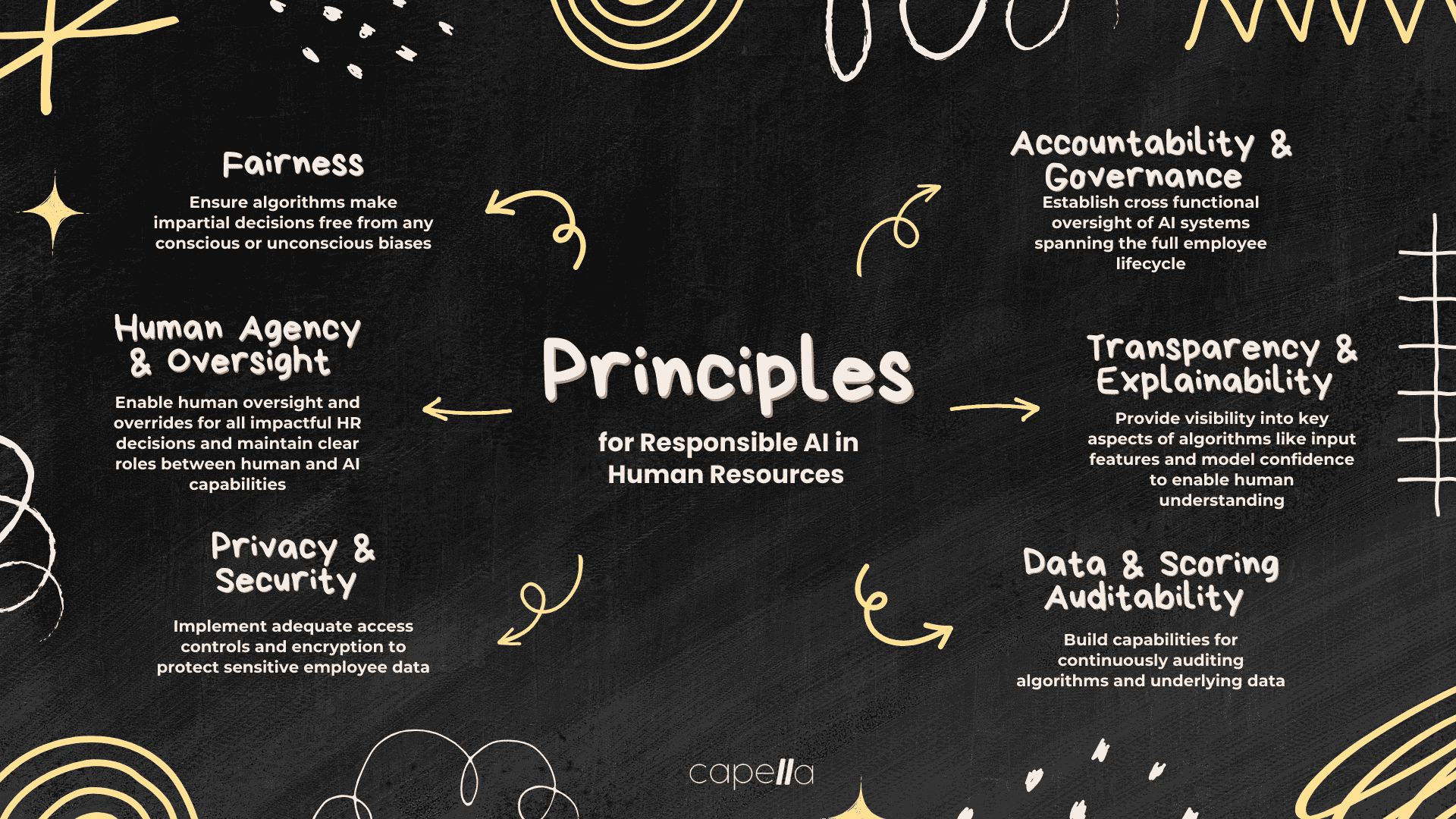

Key Takeaways
Forward-looking HR leaders have a prime opportunity to deliver enhanced strategic value by embracing AI and its powerful implications for transforming talent management. LLMs like Claude and Sydney demonstrate immense promise in this regard. However, achieving the full benefits will require deliberate planning, starting with targeted use cases versus wholesale automation. Maintaining human oversight and governance of AI systems will be critical, enabling collaborative human-machine augmentation versus full replacement of people managers. Leaders who strike the right balance have an opportunity to redefine HR and its role in driving business performance.
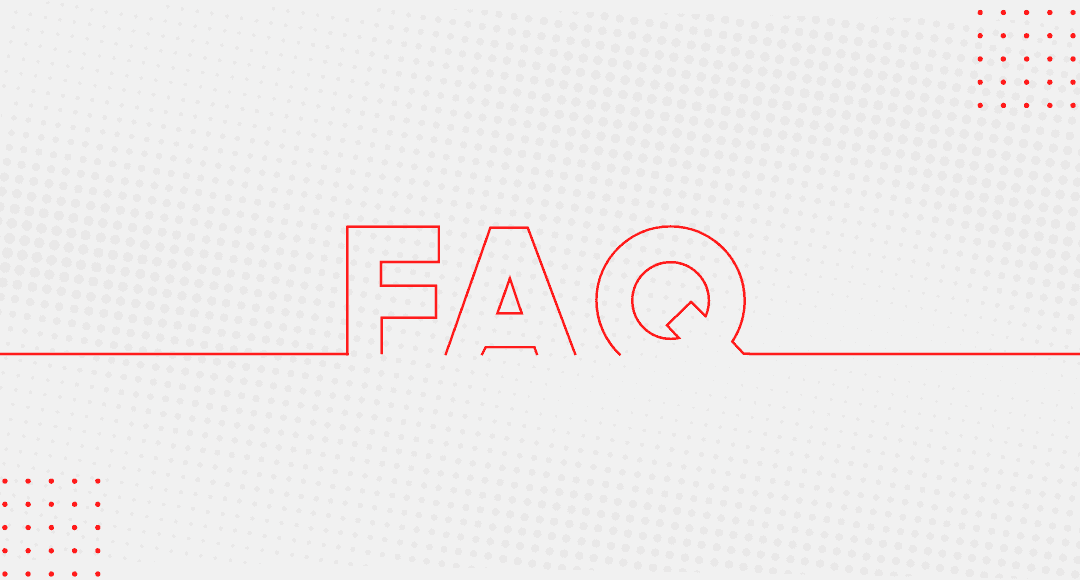
What are some of the key drivers pushing HR to embrace AI and LLMs?
HR teams today face increasing complexity on multiple fronts. Global skill shortages, dynamic business conditions, distributed workforces and more intensify talent acquisition challenges. Meanwhile, professionals expect personalized, digitally-enabled experiences. Legacy HR systems constrain efforts to deliver.
AI-powered solutions like Claude, Sydney and Bard promise to help HR organizations conquer these rising complexities through automating transactional activities, generating strategic workforce insights, and elevating employee experiences. Adoption remains patchy though as teams grapple with risks around data, algorithmic bias and lack of transparency. The opportunity lies in establishing governance to tap benefits while proactively mitigating downsides.
What are some examples of how companies are currently using LLMs for HR workflows?
- Talent acquisition teams are leveraging chatbots to qualify inbound candidates and schedule interviews based on calibrated role requirements and hiring manager availability.
- Learning platforms are integrating capabilities to recommend relevant content based on employee growth goals, project exposure and capability frameworks.
- Rewards teams use market compensation benchmarking analysis for ensuring internal equity and external competitiveness.
- Wellness partners offer personalized health incentives based on analyzed health data, engagement patterns and nudges.
In most cases though, LLMs play an assisting role rather than fully replacing existing systems or processes.
What is responsible AI and why does HR need to care about it?
Responsible AI refers to developing and deploying algorithmic systems ethically to avoid unintended consequences. This means proactively assessing for biases, ensuring transparency, enabling security and setting limits on automation to retain human accountability for people-impacting decisions.
HR in particular interfaces extensively with employees through their full lifecycle. AI risks around biased recruiting, unfair performance scoring, incorrect automation of terminations or improper data use directly impact people. Beyond moral considerations, lack of responsible AI jeopardizes talent retention and company reputation. HR leaders must champion governance frameworks addressing these.
How can HR teams mitigate bias risks with AI?
Bias mitigation should focus on the entire AI lifecycle - the algorithms themselves, training data inputs, as well as auditing mechanisms. Tactics like testing models across representative demographic groups, deliberately enhancing input data diversity, adjusting model reward systems and enabling human overrides of auto-generated decisions can help. Formalizing an oversight process is critical rather than ad hoc monitoring.
What are some key roles and structure considerations for AI Ethics boards overseeing HR projects?
Governing bodies for HR AI should comprise cross-functional leaders spanning technology, compliance, legal and workplace culture. Diversity of thought minimizes blindspots. Data and domain experts enable constructive questioning of model purpose, workflow integration, metrics, assumptions and other factors that impact output.
Centralized standards around transparency can guide teams in documenting choices at each project phase. Though some factors might merit customization based on use case nuance. Separate from these bodies, designated operating teams help implement governance protocols surrounding testing, monitoring, risk reviews and model refinement.
How can HR reskill existing workforces to complement AI capabilities versus compete?
The introduction of AI to elevate employee and candidate experiences will change HR workload composition. While mundane tasks get automated, value-added ones will grow around strategy, consultative interactions and governance.
HR leaders should proactively define role adjustments, and budget for transitional reskilling. Baseline technology literacy needs boosting to work alongside AI systems. Soft skills like creative thinking, storytelling with data and design thinking will gain prominence with tech taking over repetitive analytical parts. Multiple futures may need envisioning to determine alternate models for HR’s structure.
What are some key metrics HR can track to measure LLM success and continue driving adoption?
- Recruitment cycle time reduction
- Higher offer acceptances
- Growth in internal candidate mobility
- Survey score improvements on experience measures like career growth, supervisor effectiveness, culture index
- HR operations cost reduction
- HR transaction volume throughput
The above represent a sample of macro benchmarks that connect use of AI to overarching HR goals around talent competitiveness, retention, productivity and delivering value. Micro-metrics relating to specific workflow efficiencies also matter. Continued funding and support depends on demonstrating quick returns.
How can HR partner with enterprise IT teams in scoping and delivering AI solutions?
Cross-functional collaboration is foundational to ensuring AI/ML projects fulfil intended objectives. HR brings nuanced people insights while IT furnishes technical expertise. Joint ownership in design and deployment stages enables complementary strengths. Governance mechanisms should institutionalize shared accountability between business-side teams like HR and technical staff to maximize value.
Tactically, HR professionals can participate in early solution scoping calls to detail pain points, objectives and success indicators from an end-user lens. They can enrich limited datasets that data science teams utilize. Testing phases allow them to provide contextual qualitative feedback on model performance while engineers focus on mathematical measures. Beyond implementation, HR plays an indispensable role in Training and Change Management to drive workforce adoption.
What are some pitfalls HR should avoid with expanding AI usage?
- Prioritizing technology over problem definition - Don’t chase AI for its own sake without tying usage to business goals
- Wholesale automation - Retain human oversight for impactful people decisions rather than end-to-end self-service
- Data errors propagating risk - Question model inputs and assumptions thoroughly to avoid “garbage in, garbage out”
- Mishandling private information - Implement stringent security protocols around employee data access and usage
- Overtrusting algorithms as absolute truth vs suggestions - Account for data gaps skewing insights, continuously validate outputs
- Isolating models as set-and-forget instances - Monitor systems continuously as data environments rapidly evolve
- Obsoleting existing workforce expertise too fast via automation - Plan adequately for transitional reskilling and structural changes
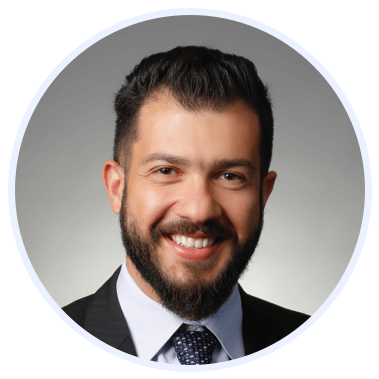
Rasheed Rabata
Is a solution and ROI-driven CTO, consultant, and system integrator with experience in deploying data integrations, Data Hubs, Master Data Management, Data Quality, and Data Warehousing solutions. He has a passion for solving complex data problems. His career experience showcases his drive to deliver software and timely solutions for business needs.