

We are in the midst of a new era of healthcare, one where AI and data are enabling a revolution in personalized medicine. No longer will treatments be one-size-fits-all - instead, they will be tailored to each patient's unique biology, lifestyle, and preferences. This shift promises to dramatically improve patient outcomes while lowering costs.

The Promise of Precision Medicine

Precision medicine is an approach that takes into account individual differences in patients' genes, environments, and lifestyles when designing preventative and treatment strategies. It stands in contrast to the "one-size-fits-all" approach that has dominated medicine for decades.
With advanced AI and genomic sequencing, doctors can now identify the specific biological mechanisms underlying a patient's disease and select the treatments most likely to be effective based on the patient's unique profile. Rather than trial-and-error, treatments can be targeted to what will work best for that particular individual.
Research by Deloitte found that "precision medicine can potentially enable savings of up to $100 billion in annual healthcare spending in the United States alone." Other estimates suggest savings could be even higher - up to $600 billion per year.
In addition to lower costs, precision medicine delivers better outcomes for patients. A study published in Nature found that "matching tumor sequencing to drug selection improved progression-free survival times" - from 2.2 months on physician's choice to 5.7 months on matched targeted therapy. Even when the matched drug was not FDA approved for that specific indication, it still outperformed alternatives.
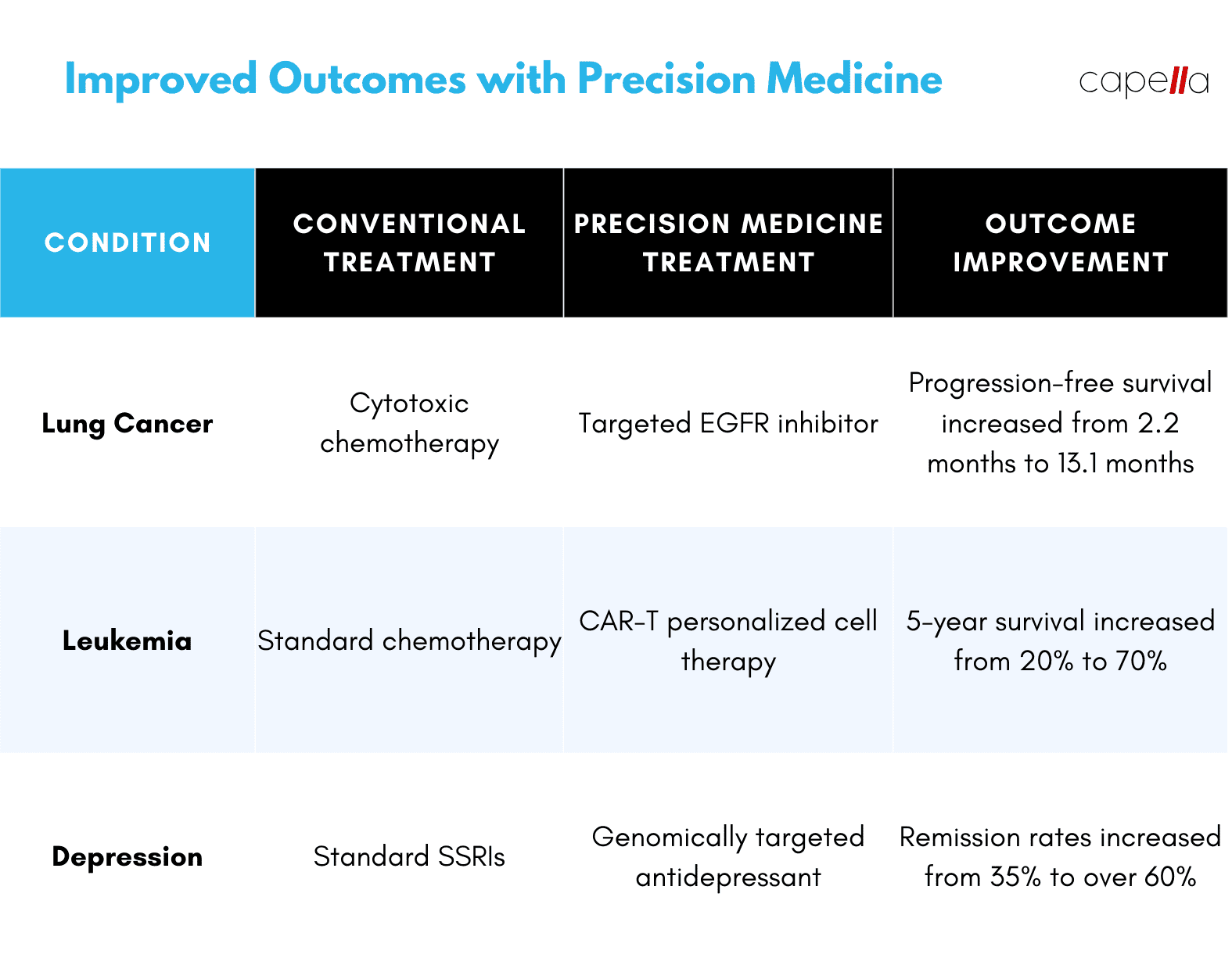
The Role of Artificial Intelligence

A key driver making precision medicine possible is artificial intelligence. AI is revolutionizing healthcare by uncovering patterns in vast amounts of data that humans alone cannot.
AI algorithms can analyze a patient's clinical information, family history, genome sequence, microbiome composition, diet, lifestyle factors, and more. It can then compare this data against tens of thousands of other patients to predict which treatments will be most effective for a given individual.
Machine learning techniques like neural networks excel at finding subtle correlations across many variables that could easily elude human analysis. Yet these patterns can provide vital biological insights.
For example, AI research published by Google AI in 2020 demonstrated that algorithms could predict the risk of breast cancer recurrence from mammogram images alone more accurately than human radiologists. The AI was able to identify textural patterns imperceptible to the naked eye that contained prognostic information.
DeepMind has also shown that AI analysis of medical images can spot early signs of eye disease and recommend timely treatment. This can prevent severe vision loss in patients.
In drug discovery, AI methods like deep learning and generative models can explore astronomical combinatorial spaces of potential drug compounds that no human researcher could fully investigate. This accelerates the search for new precision treatments tailored to narrow disease subtypes or genetic profiles.

Real-World Implementation

Precision medicine powered by AI is moving from the lab to real-world clinical practice. Patients around the globe are already benefiting from more targeted therapies.
In oncology, personalized treatment planning is becoming standard practice. Providers like Tempus and SOPHiA GENETICS use AI to analyze patient's genomic profiles along with structured and unstructured clinical data to match their tumor signatures to targeted therapies.
Code example of genomic variant analysis in Python:
import pandas as pdimport variant_annotationvariants = pd.read_csv("patient_variants.csv")annotated_variants = variant_annotation.annotate(variants) actionable_variants = annotated_variants[annotated_variants['clinical_action'].notna()]print(actionable_variants)
This allows oncologists to select from approved treatments as well as experimental drugs in clinical trials most likely to benefit that patient.
AI-enabled apps like ZERO let patients take proactive role in prevention. The ZERO app analyzes user's genetics, blood biomarkers, lifestyle habits and family history to provide personalized recommendations on cancer risks factors to minimize.
In rare disease, AI supports more accurate and faster diagnosis. For patients with undiagnosed conditions, providing the right treatment can be a major challenge. But algorithms can search medical literature, patient databases, and peer-reviewed studies to match unusual symptoms to probable diagnoses.
For example, Isabel Healthcare's AI leverages natural language processing (NLP) to intake clinical notes and lab results and suggest possible rare diseases that physicians should investigate. This gives doctors a starting point when dealing with puzzling medical cases.
Apps like Natural Cycles use sensor data and AI to provide women personalized fertility advice and contraceptive planning tailored to their unique menstrual cycles and physiology.
In psychiatry and mental health, AI chatbots like Woebot use NLP to have empathetic conversations with patients. This provides 24/7 emotional support and therapy customized to an individual's needs and frame of mind.
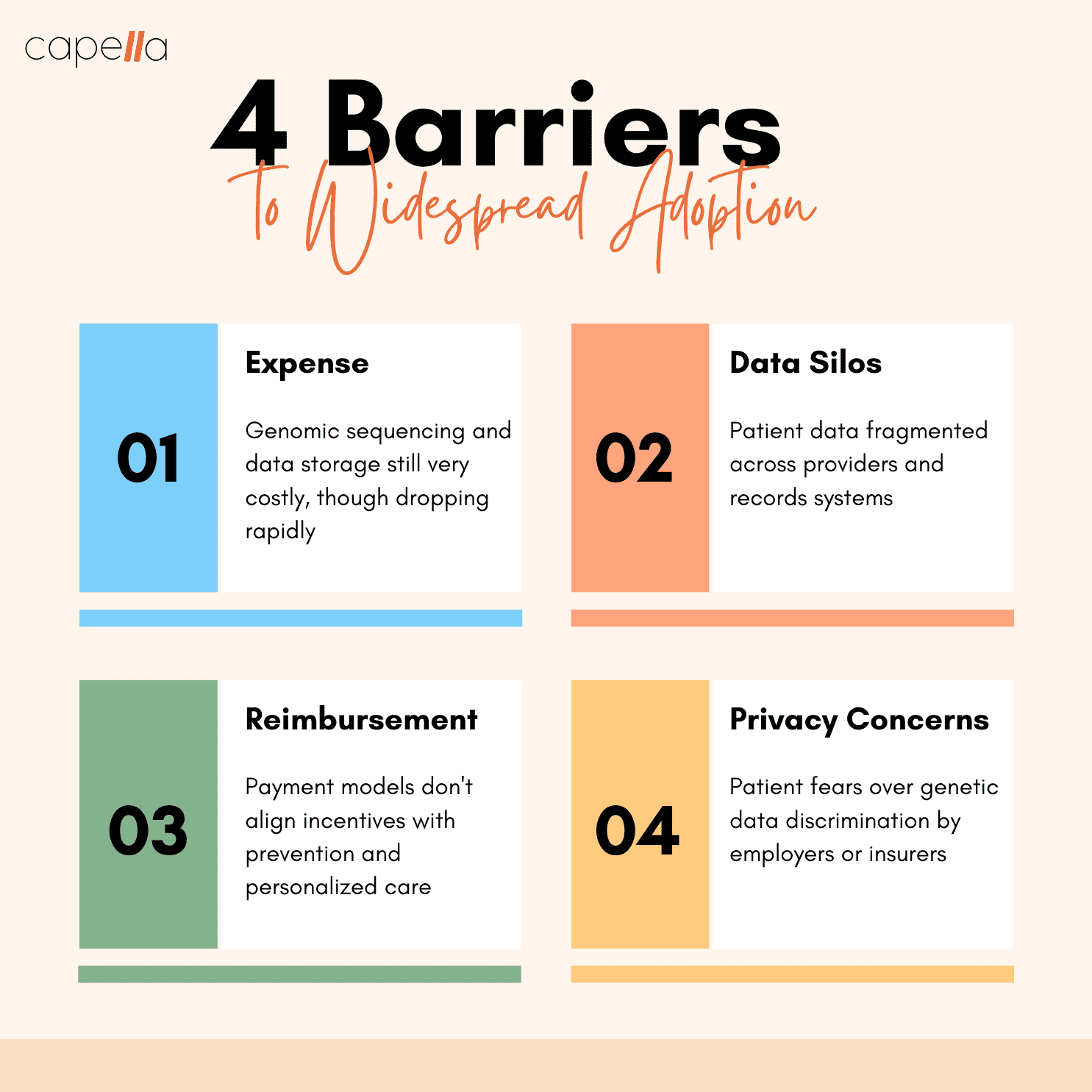
Challenges to Overcome

While great strides are being made, there are still challenges to expand AI-driven precision medicine. Here are some key issues to overcome:
- Data fragmentation - Patient health data is notoriously fragmented across providers. Compiling complete profiles requires better data integration.
- Algorithmic bias - Like humans, algorithms can demonstrate bias. Safeguards must be put in place to ensure AI recommendations are equitable across all patient groups.
- Regulation - Clear regulatory standards are still being defined to evaluate safety and efficacy of AI in healthcare. Explainable AI models will be important for clinical acceptance.
- Reimbursement - Payment models need to be realigned from fee-for-service to value-based care to incentivize personalized treatments.
The Future with Patients at the Center
The full promise of precision medicine is empowering patients to take charge of their own health. AI will enable curated, personalized recommendations for each individual to optimize wellness and longevity.
Sequencing, sensors, and AI will provide real-time biological insights. Instead of sporadic checkups, health data will be continuously monitored to detect any deviations from one's normal baseline. AI assistants will provide 24/7 support to interpret results and offer tailored, timely advice.
Medicine will shift from reactive to preventative, driven by patients not providers. Healthcare will finally put the individual first, not one-size-fits-all population averages. This patient-centered model aligned with our unique biology holds incredible potential to transform human health and longevity.
Conclusion
Precision medicine powered by AI represents an immense opportunity to improve patient outcomes while lowering healthcare costs. By applying advanced analytics to rich biological, clinical, and lifestyle data, AI can unlock personalized treatments tailored to each individual. Significant progress has already been made applying these principles in oncology, rare disease, mental health, and other areas. Overcoming key challenges like data integration and algorithmic bias will accelerate adoption. Ultimately, AI stands to make medicine preventative, predictive, and patient-focused - finally aligning treatments to our unique genetic makeups.
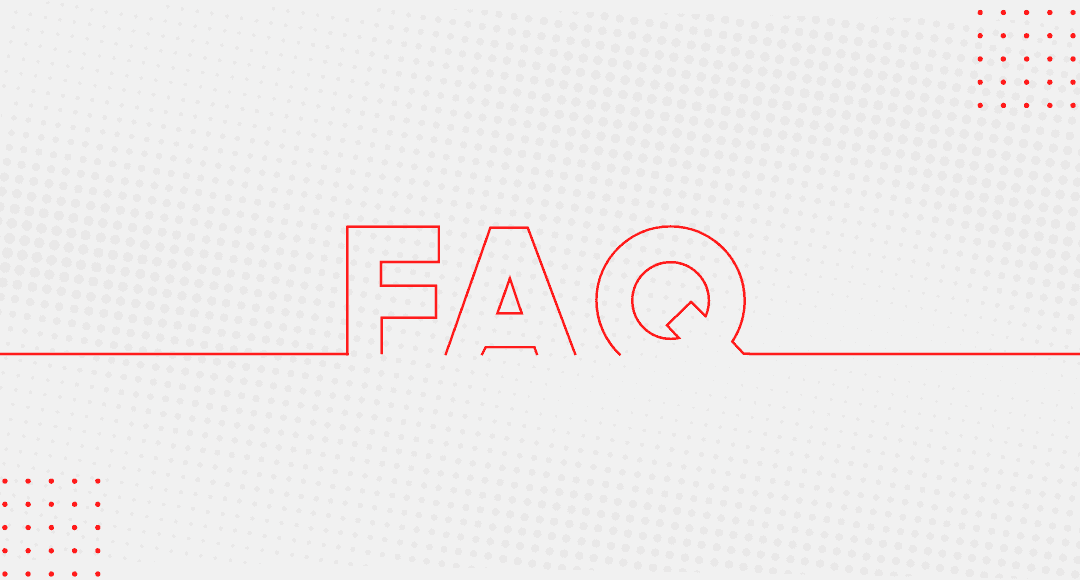
1. What is personalized or precision medicine?
Personalized medicine is a medical model that customizes healthcare to each patient's unique biological makeup, lifestyle, and environmental factors. Instead of a one-size-fits-all approach, preventative strategies and treatments are tailored to an individual's specific profile. This can incorporate information on one's genes, proteins, microbiome, diet, exercise patterns, and more. The goal is to target interventions to maximize effectiveness based on a deep understanding of the patient's unique health status and risks.
2. How is personalized medicine made possible today?
Advances in artificial intelligence and expanded access to detailed biological data are enabling personalized medicine approaches. AI can analyze extensive health data from electronic records, genomics, medical imaging, wearables, and more to determine the most effective predictive, preventative, and therapeutic strategies for each person. Expanding capabilities also now exist to collect and interpret detailed molecular profiles through genetic sequencing, biometric tracking, and microbiome sampling.
3. What are the benefits of personalized medicine?
Personalized care can improve patient outcomes by assessing risk factors and detecting conditions earlier when they are most treatable. It can also improve treatment efficacy by identifying therapies most likely to be effective and safe based on an individual's profile. This also reduces exposure to unnecessary, ineffective therapies that could cause side effects. On a larger scale, personalized medicine may lower overall healthcare costs by reducing medical errors, avoidable hospitalizations, and system inefficiencies.
4. What types of data are used in personalized medicine?
Many types of health data can provide insights into an individual's risks and optimal treatments. These include genomics (DNA sequencing), proteomics (protein profiles), metabolomics (chemical makeup), microbiomics (gut flora), medical history, diet, lifestyle habits, cellular biomarkers, physiological metrics, and more. AI help makes sense of this vast heterogeneity across individuals to drive personalized care.
5. How is AI enabling personalized medicine?
AI techniques excel at rapidly analyzing massive, diverse datasets to uncover patterns that can provide biological insights. Advanced algorithms can combine details on genes, lab tests, environment, lifestyle factors and health history to predict disease risk, identify optimal therapies, and more accurately diagnose conditions for each patient. AI also aids researchers in rapidly screening potential new treatment options customized to narrow disease subtypes and genetic profiles.
6. How is personalized medicine used in cancer treatment?
In oncology, physicians now routinely sequence the specific mutations driving tumor growth to select targeted therapies that specifically inhibit those pathways. Immuno-oncology leverages biomarkers to identify treatments most likely to activate a patient's immune system against cancer cells. Patients also receive support matching to clinical trials testing novel therapies for their specific cancer variant. AI aids diagnosis, treatment selection, clinical trial matching, and therapy response monitoring.
7. What role does personalized medicine play in rare disease diagnosis?
Patients with rare, undiagnosed conditions often undergo lengthy diagnostic odysseys. But AI tools can help accelerate diagnosis by surfacing possible rare diseases that align with a patient's profile from across warehouses of published case reports and scientific literature that physicians could never survey alone. This provides doctors a starting point when dealing with puzzling medical cases caused by uncommon genetic mutations.
8. How is pharmacogenomics related to personalized medicine?
Pharmacogenomics studies how genetic variation impacts drug metabolism and response. It enables selecting and dosing therapies based on a patient's genomic profile in order to maximize efficacy and minimize adverse reactions. AI can help predict response and optimize treatment regimens accounting for an individual's pharmacogenomic makeup.
9. What are key barriers to broader adoption of personalized medicine?
Hurdles including high upfront costs, fragmented data, lack of reimbursement frameworks, regulatory uncertainty around emerging technologies like AI, privacy concerns, and more need addressing to support wider implementation. Educating clinicians and aligning financial incentives will also aid adoption. But the trend is clearly toward a more personalized model of healthcare.
10. What does the future look like for personalized medicine?
In the future, preventative care may be driven by continuous patient monitoring through wearables, sensors, and frequent sequencing to enable truly personalized real-time interventions. Privacy-preserving AI assistants could offer 24/7 support interpreting results and providing tailored health advice. Treatments could leverage synthesized-on-demand therapies like cell and gene editing. The line between consumer wellness and healthcare may blur, with patients fully empowered partners in their lifelong health.
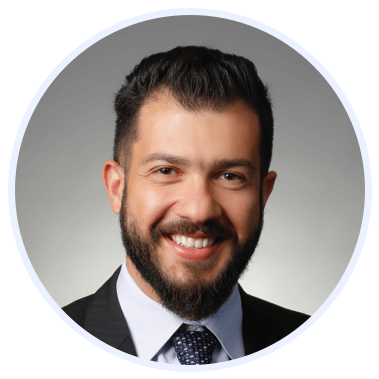
Rasheed Rabata
Is a solution and ROI-driven CTO, consultant, and system integrator with experience in deploying data integrations, Data Hubs, Master Data Management, Data Quality, and Data Warehousing solutions. He has a passion for solving complex data problems. His career experience showcases his drive to deliver software and timely solutions for business needs.