
Remote monitoring has become an increasingly critical capability for enterprises in recent years. The ability to monitor infrastructure, applications, and business processes remotely provides organizations with enhanced visibility, faster response times, and data-driven insights. When combined with artificial intelligence (AI) and machine learning, remote monitoring takes on even greater potential to proactively identify issues, predict outcomes, and drive automation.
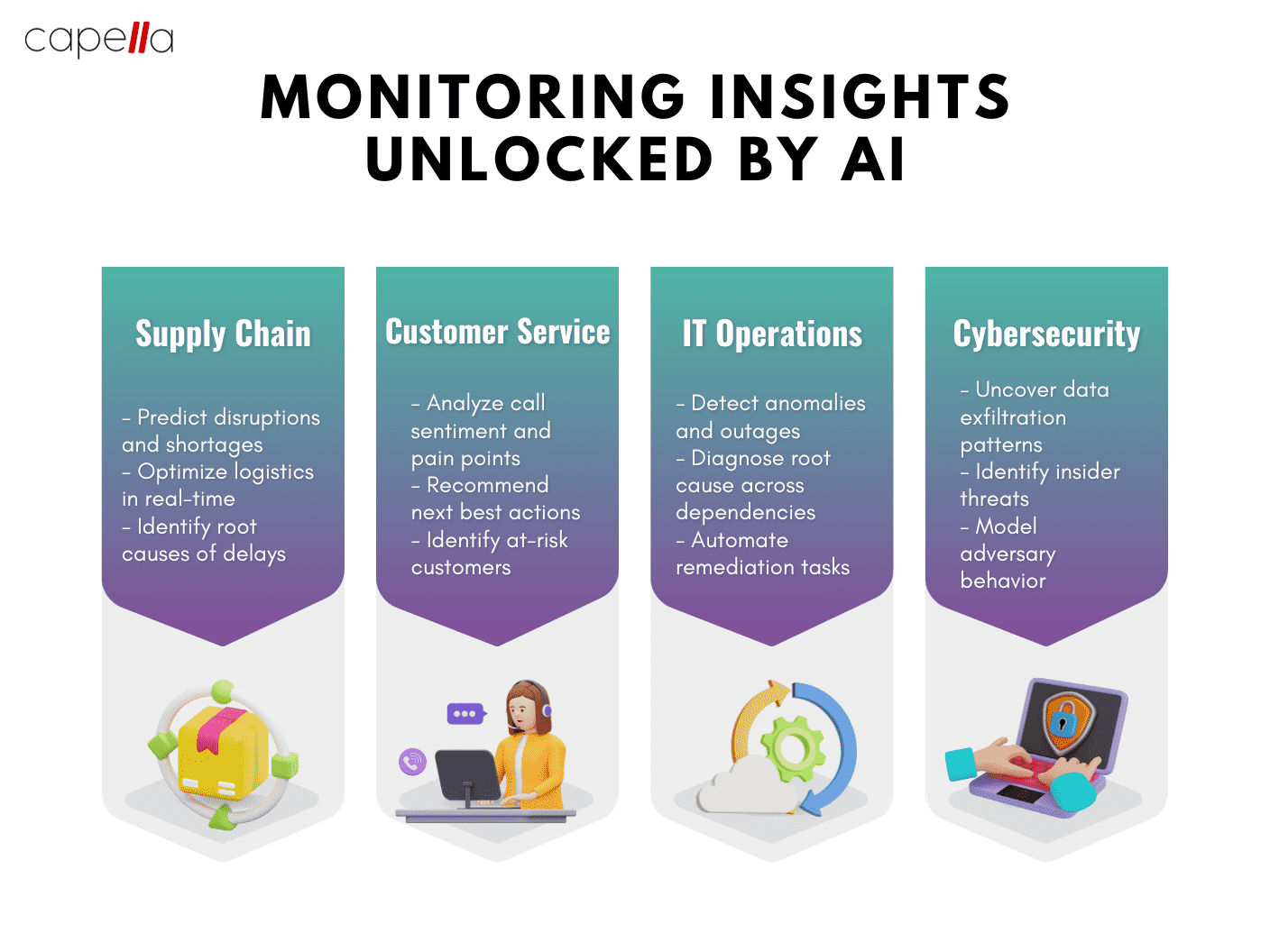
In this blog, we'll explore how enterprises can leverage AI-powered analytics to get the most out of their remote monitoring initiatives. We'll look at real-world use cases, best practices, and key factors to consider when implementing these capabilities. Whether you're just starting your remote monitoring journey or looking to maximize an existing deployment, read on to learn how AI can be a game-changer.
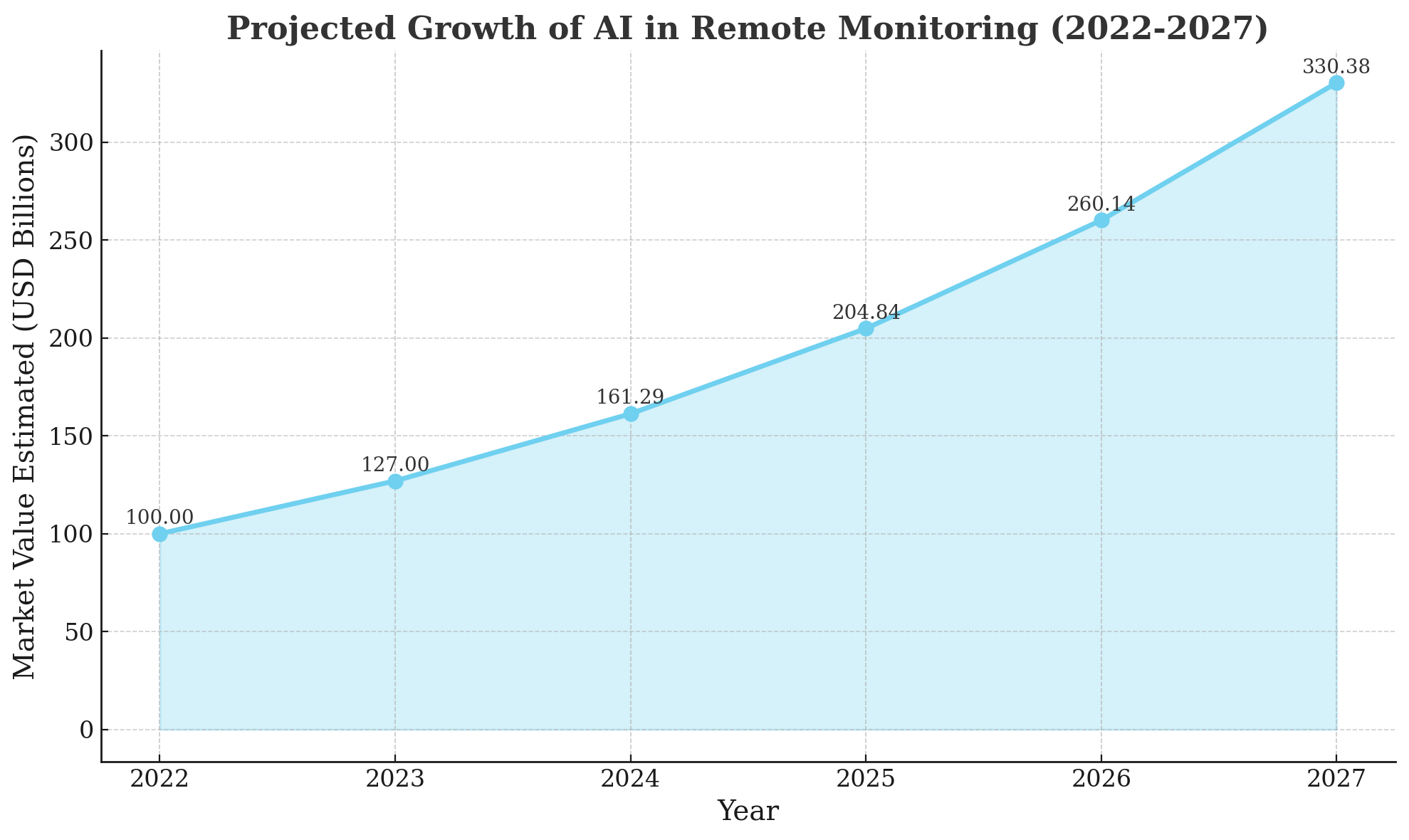

The Promise of AI for Remote Monitoring

Remote monitoring generates massive amounts of data across the enterprise. Sensors, cameras, applications, networks, and more are continuously generating telemetry that provides insight into the health, performance, and behavior of critical infrastructure and processes. However, the scale of this data quickly becomes overwhelming for human analysts.

This is where AI comes into play. Modern AI techniques including machine learning, natural language processing, computer vision, and more can help enterprises unlock greater value from their monitoring data. Key capabilities enabled by AI include:
- Pattern recognition - identify anomalies and detect issues proactively
- Predictive analytics - forecast problems and outcomes before they occur
- Intelligent alerting - separate noise from real incidents requiring human intervention
- Automated remediation - take action to resolve issues automatically based on predefined logic
- Human-like interaction - enable natural language interfaces for easy querying and collaboration

According to recent research by Mordor Intelligence, the use of AI in remote monitoring and management solutions is projected to grow at a CAGR of 27% from 2022-2027. The drivers behind this growth include the need for proactive monitoring, increased adoption of IoT devices, and high data velocity from 5G networks. Enterprises are recognizing the power of AI to enhance their visibility and response while optimizing limited human resources.
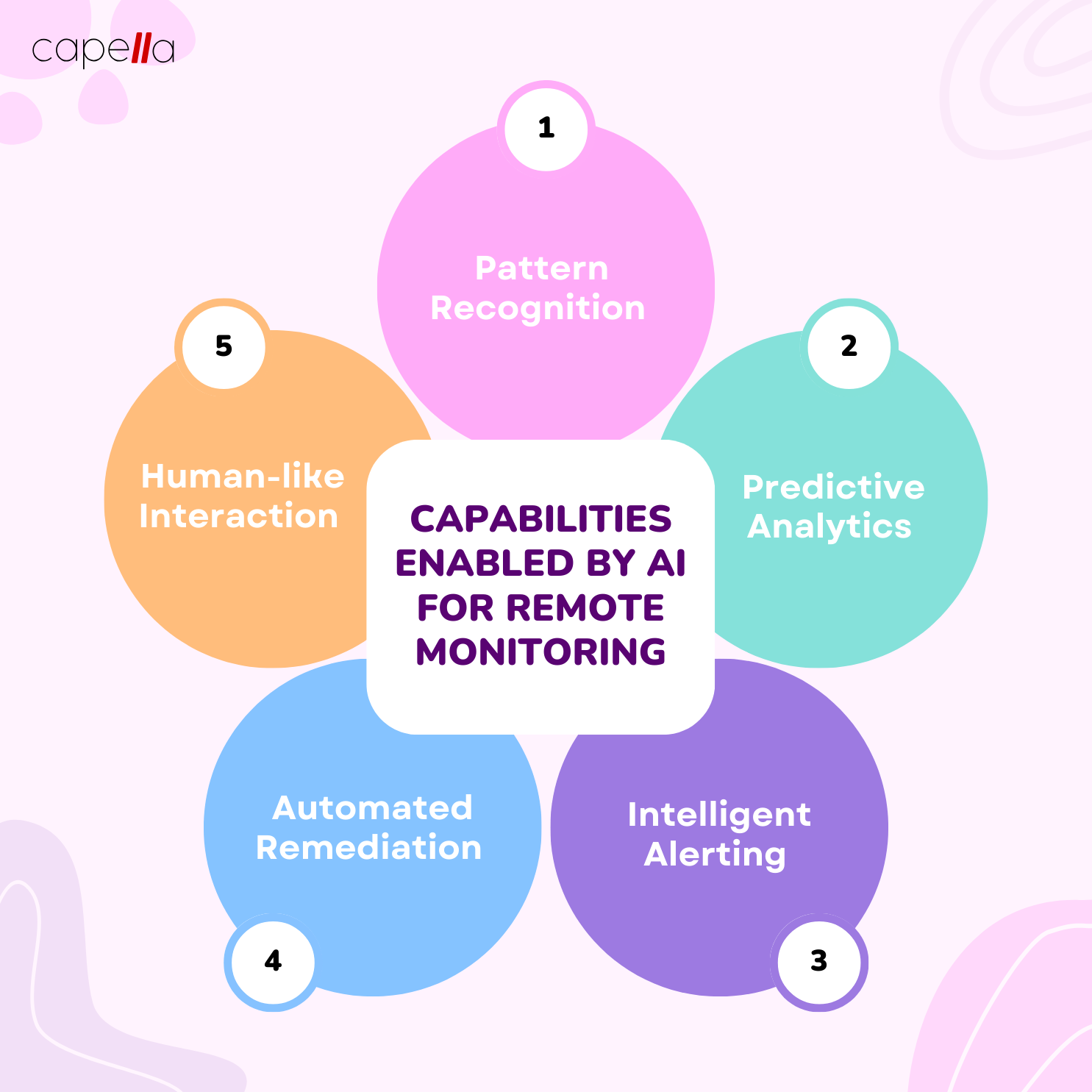
Real-World Use Cases

AI and ML are transforming remote monitoring across a diverse range of industries and applications. Here are just a few examples:
Infrastructure Monitoring
From server racks to factory floors, physical infrastructure must be closely tracked to minimize downtime. AI helps massively scale this monitoring by enabling capabilities like:
- Smart cameras that identify occupancy levels, social distancing, mask wearing, and other critical data
- Anomaly detection on server sensor data to spot failing components
- Predictive maintenance that forecasts equipment failures before they happen
- Automated ticketing and dispatch when human intervention is required
By leveraging computer vision and deep learning algorithms, AI can ingest data from all manner of sensors and cameras to provide holistic monitoring coverage. Humans are freed up to focus on higher judgement decisions.
Network & Application Performance Monitoring
Modern networks and applications produce immense volumes of performance data. AI algorithms help parse this data to:
- Spot trends predicting usage surges and adapt capacity accordingly
- Detect anomalies indicating potential cyber threats or DDoS attacks
- Identify root causes of issues across integrated IT environments
- Trigger automated remediation actions to resolve problems
Machine learning models can be trained to establish dynamic baselines for normal behavior across network and app performance. This allows the AI to discern important patterns from noisy data and take intelligent action.
Business Process Monitoring
Tracking key business processes end-to-end is vital for performance and compliance. AI enables executives to keep an eye on critical workflows such as:
- New customer onboarding
- Order fulfillment
- Supply chain logistics
- Claims processing
- Payments
Natural language interfaces allow business users to query the status of processes in plain English. Sentiment analysis can detect pain points and bottlenecks before they impact customers. Process mining techniques identify optimization opportunities.
In essence, AI allows organizations to monitor their business processes with the same level of insight as their technical operations. This drives agility, productivity, and risk reduction across the enterprise.
Remote Worker Monitoring
The rise of remote work has increased the need to monitor employee experience and productivity. AI-powered solutions help organizations:
- Gauge collaboration levels, knowledge sharing, and sentiment
- Analyze usage patterns across remote work apps
- Optimize workloads based on individual and team capacity
- Enhance security and data protection for remote endpoints
Advanced natural language processing parses communications, documents, and tasks to uncover crucial insights without compromising privacy. This allows executives to guide culture, improve workflows, and enhance performance across remote teams.

Best Practices for Implementation

Deploying AI-powered remote monitoring creates immense value but does require careful planning and execution. Here are a few best practices to drive maximum impact:
Start with a targeted use case - Given the breadth of monitoring AI can enable, it's best to start with a well-defined deployment that solves a clear pain point. Let this initial use case prove out capabilities and build confidence.
Ingest quality data - Your algorithms are only as good as the data behind them. Prioritize capturing high-quality data sources with richness and completeness. Clean up historical data for training.
Leverage hybrid AI approaches - Combine multiple techniques like computer vision, NLP, graph algorithms, etc. based on your problem space. Hybrid models outperform single-method approaches.
Focus on explainability - For stakeholders to trust and adopt AI, it must provide explanations for its predictions, decisions, and recommendations. Prioritize transparent algorithms.
Enable human oversight - Even as you scale AI, keep humans in the loop at key points to validate the AI's work. This allows for correction, learning, and continuous improvement.
Measure performance diligently - Rigorously measure AI accuracy, explainability, adoption, and business impact over time. This benchmarks success and helps identify areas for tuning.
Plan for change management - Adoption of AI requires aligning people, processes, and culture. Get buy-in across teams through training and clear change management.
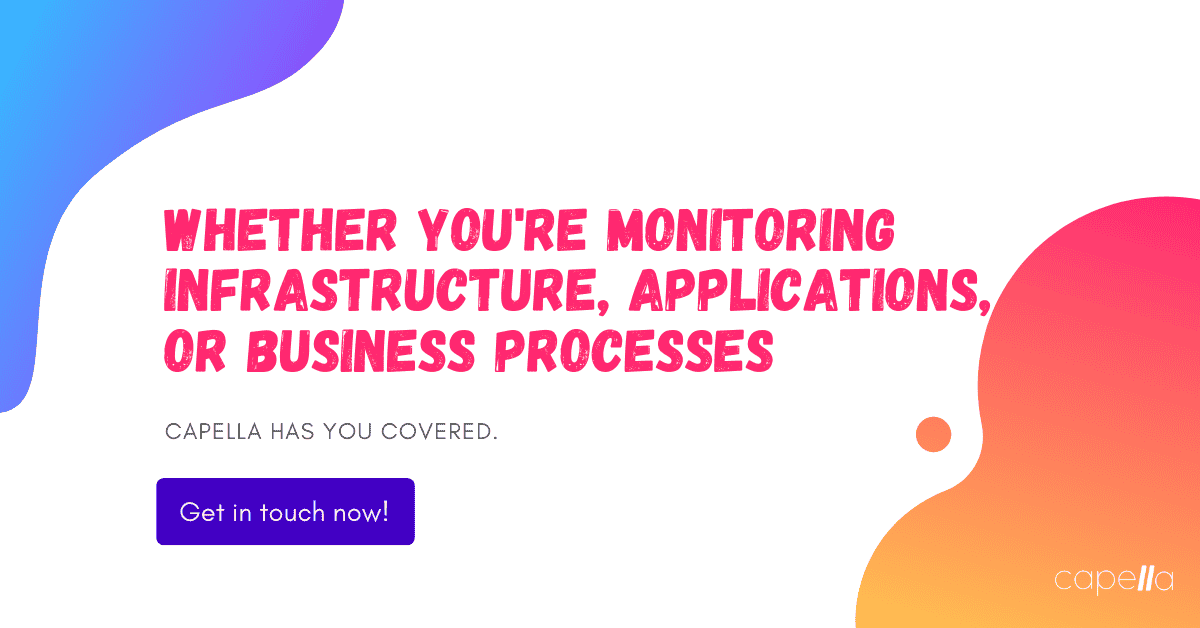
The Future with AI is Bright
AI is truly transforming remote monitoring, enabling proactive visibility at cloud scale. With the right strategy, enterprises can harness AI's potential to optimize costs, reduce risks, and enable predictive operations. Although challenges remain around data quality, algorithm opacity, and adoption, the technology is improving rapidly.
Organizations that embrace AI now will gain a considerable competitive advantage as this technology becomes a baseline capability across industries. By leveraging the latest AI capabilities and best practices, enterprises can turn their remote monitoring data into a strategic asset that drives smarter decisions, greater efficiency, and reduced downtime. The future with AI is bright - the time to put these powerful capabilities to work is now.
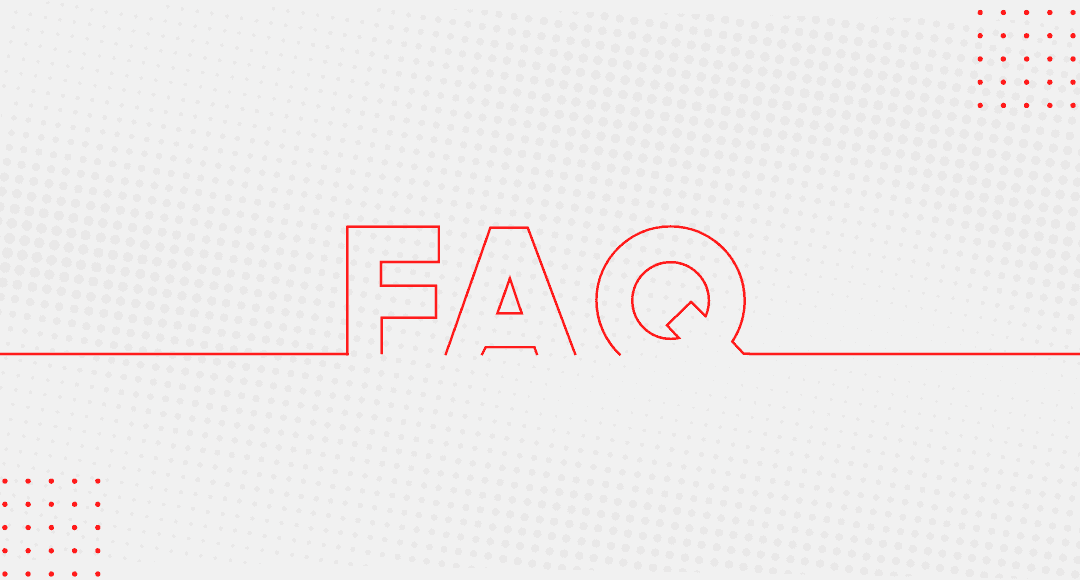
1. What are the main benefits of using AI analytics for remote monitoring data?
Applying AI and machine learning algorithms to remote monitoring sensor data enables more accurate anomaly detection, failure prediction, pattern recognition, forecasting, and optimization. This allows operators to detect issues proactively before they cause downtime. AI analytics also helps identify root causes from the mass amounts of timeseries data. The continuous learning capabilities of AI improve detection accuracy over time. Overall, AI analytics increases uptime, reduces maintenance costs, and prevents operational disruptions caused by equipment failures or process upsets.
2. What types of sensors and data work best for AI analytics?
Rich timeseries sensor data with high contextuality and many relevant parameters tends to yield the most accurate AI models. Sensors that measure vibration, temperature, pressure, flow rates, voltage, current, and other operational metrics provide crucial insights. High frequency data (eg. kilohertz sampling) allows detecting emerging faults earlier. Images, video, and audio data can also train computer vision and speech analytics models. Collecting data during known failure events provides the labeled examples needed for supervised learning. Metadata like environmental conditions, operating context, work orders, and maintenance logs helps AI models interpret the signals.
3. How do you ensure good data quality for remote monitoring AI?
Carefully designed sensor networks with redundancy, overlapping coverage, and failover provides robust data collection. Validating sensors calibrations and performance establishes trust. Cleanly timestamped data with reliable connectivity enables proper temporal analysis. Checking for missing values, gaps, outliers, and noisy data prevents training models on bad data. Tracking sensor and connection health flags unreliable data for exclusion. Enriching raw data with contextual metadata enables deeper insights.
4. What expertise is required to implement AI analytics?
Getting started with pre-built AI applications for common monitoring use cases minimizes in-house expertise needed. Vendor solutions encapsulate complex data science into easy-to-use products. However, some knowledge in areas like statistics, analytics, reliability engineering, and machine learning helps evaluate vendor claims critically. Understanding monitoring program objectives and domain knowledge of the target assets is crucial for contextualizing and acting upon AI model outputs. Start with tight feedback loops between data scientists, reliability engineers, and operators.
5. How long does it take to see benefits from AI analytics?
Some basic reporting dashboards and alerts can be configured in just a few weeks by leveraging canned AI models. But, training custom AI models specific to your equipment can take several months to collect labeled data encompassing different operating regimes and failure modes. Plan on continuous model retraining and improvement. The highest value areas to pilot AI analytics are high-cost failures with clear predictive signals available in existing monitoring data.
6. How do you integrate AI analytics into existing systems?
Leveraging OPC UA, MQTT, and open APIs allows integrating AI analytics with data sources like distributed control systems, SCADA, and historians. Cloud platforms simplify aggregating dispersed data. For example, combining IoT data streams with computerized maintenance management system (CMMS) work orders can link AI model outputs to recommended maintenance actions. Presenting alerts and insights via mobile notifications allows technicians to act quickly.
7. How can AI analytics augment human operators?
Humans interpret nuanced context that AI cannot. Domain experts should validate model results. However, AI can surface insights from enormous data streams that humans cannot process alone. The goal is seamless collaboration - AI handles tedious data crunching and pattern recognition, while humans provide contextualization and nuanced decision making. With proper human oversight, AI can augment operators to optimize performance.
8. How do you build trust in AI model recommendations?
Trust comes from proving an AI model works consistently in real operational conditions over time and across a range of abnormal situations. Transparency into model factors and internal logic builds understanding. Showing examples of successful and erroneous predictions provides confidence. Tracing runtime performance metrics like precision, accuracy, and recall demonstrates reliability. Providing operators the ability to correct bad predictions further improves model robustness and trust.
9. How is edge analytics impacting monitoring and AI?
Performing analytics at the edge reduces reliance on cloud connectivity. Edge compute resources allow analyzing data and running AI models locally to enable real-time condition monitoring and closed loop control. This is crucial for use cases with milliseconds latency constraints. Distributed edge analytics also provides inherent data redundancy. The downsides are higher hardware costs and lack of centralized data.
10. What is the future roadmap for analytics and monitoring?
The continued exponential growth in sensor data volume and velocity will require ever more sophisticated AI and machine learning. Democratizing these technologies through autoML and intuitive tools will empower non-experts. Hybrid physics-based and data-driven models could deliver deeper physical insights along with predictive power. And further progress in areas like tiny sensors, battery technology, and low-power wireless networking will enable ubiquitous condition monitoring.
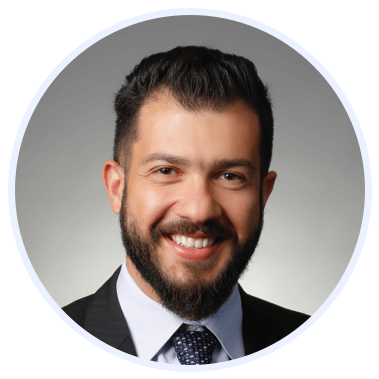
Rasheed Rabata
Is a solution and ROI-driven CTO, consultant, and system integrator with experience in deploying data integrations, Data Hubs, Master Data Management, Data Quality, and Data Warehousing solutions. He has a passion for solving complex data problems. His career experience showcases his drive to deliver software and timely solutions for business needs.