
The insurance industry relies heavily on audits to ensure accuracy and compliance. However, traditional audit processes can be extremely tedious and time-consuming. There is a tremendous opportunity to streamline audits by taking advantage of new technologies like data analytics and artificial intelligence. In this post, we'll explore practical strategies for transforming audit workflows to be faster, smarter, and more effective.

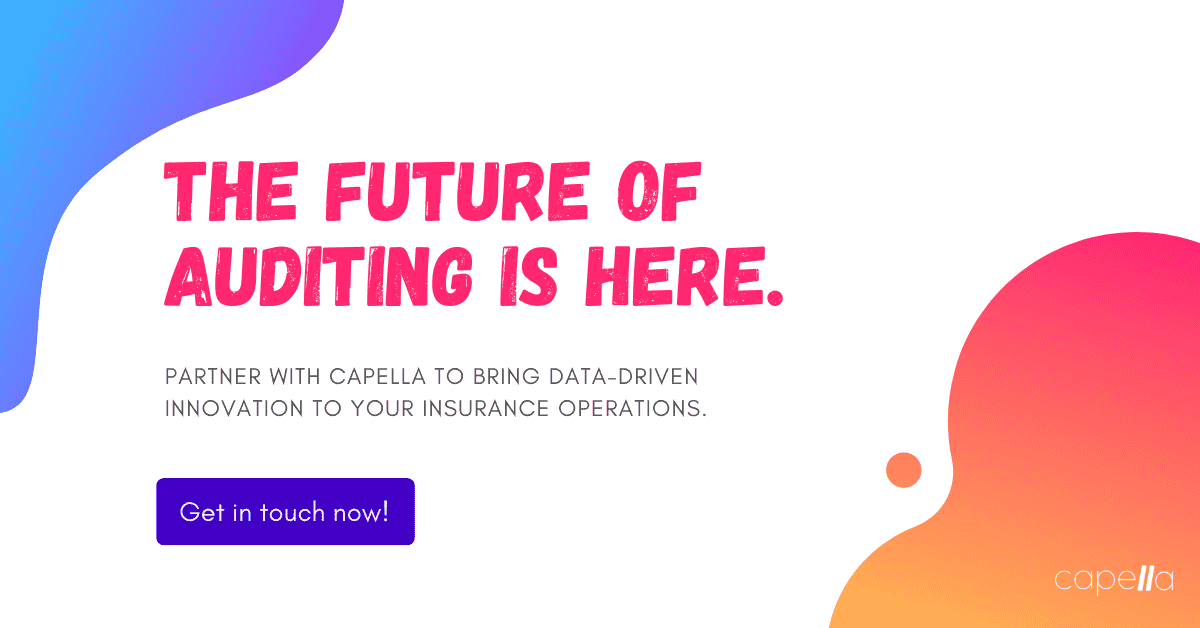
The Challenges of Traditional Auditing
Most insurance companies conduct audits on a regular basis to verify information, monitor quality, and reduce fraud. Agents, underwriters, and claims adjusters need to provide documentation to prove that policies were issued properly, claims were handled accurately, and regulations were followed.
For auditors, reviewing all this documentation is a manual and labor-intensive process. Just pulling the necessary files can take hours or days, especially if data is scattered across multiple legacy systems. Once all the information is compiled, auditors must comb through it line-by-line to identify any discrepancies or issues.
This kind of auditing requires deep expertise and meticulous attention to detail. Yet it still relies heavily on human judgment, which can be prone to bias and inconsistency across auditors. Not to mention, sampling-based auditing techniques may miss some exceptions or problem areas.
As a result, audits end up being extremely slow, expensive, and frustrating for all parties involved. Frequent audits disrupt operations for frontline teams and eat up valuable time for managers. At the same time, auditors are stuck doing repetitive, low-value tasks instead of more strategic analysis.

The Promise of Data-Driven Auditing
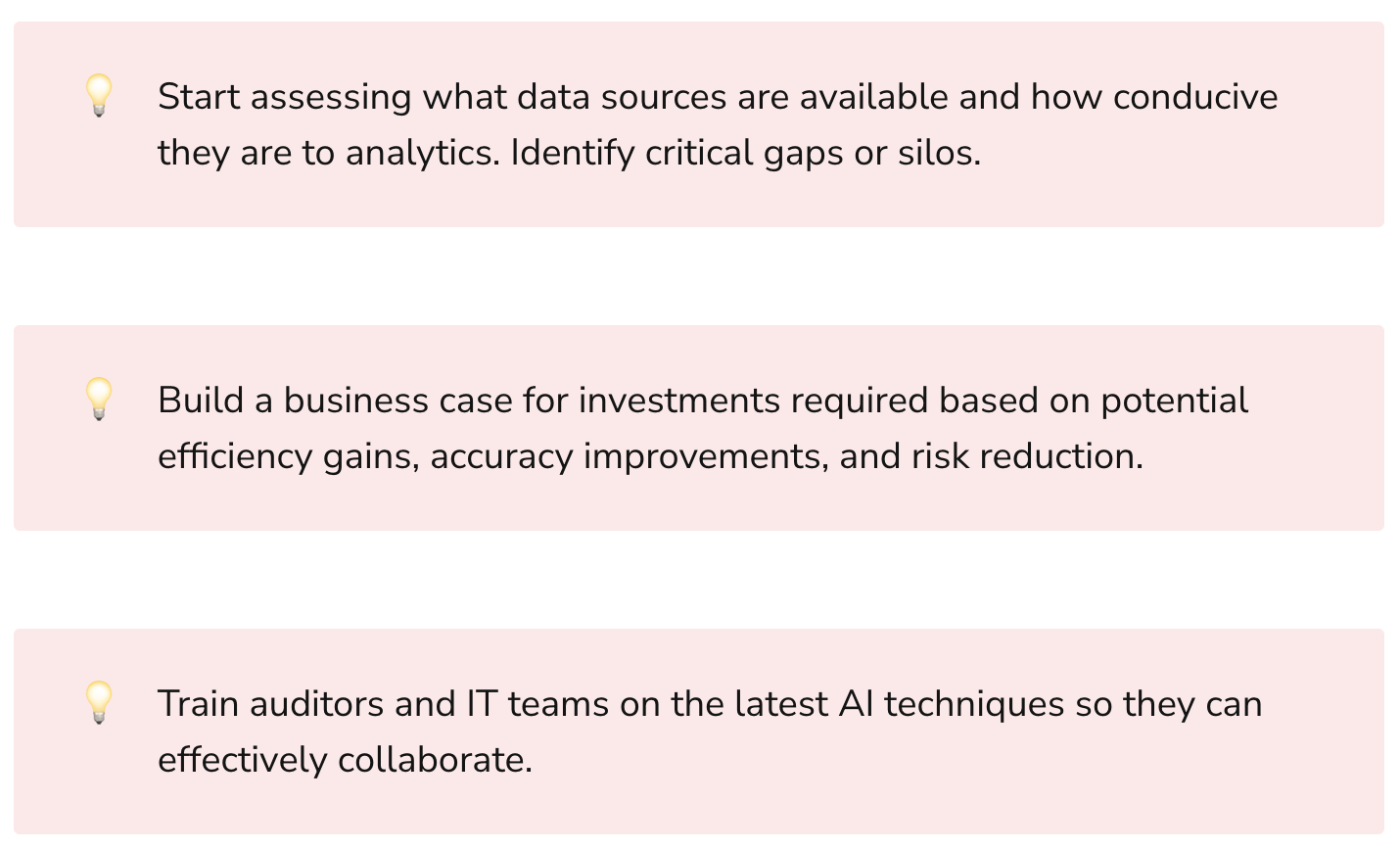
Thankfully, new technologies are emerging that can help streamline audits in the insurance industry. Analytics and AI have the potential to automate many of the manual steps in auditing while also improving accuracy.
Insurance companies already have huge volumes of data from their core policy administration and claims systems. This data contains a wealth of insights that can be leveraged for auditing, if processed correctly.
With modern cloud platforms and advances in AI, it's now possible to ingest and integrate data from across an insurer's disparate systems. This enables creating a unified source of truth and applying advanced analytics at scale.
In terms of techniques, supervised machine learning algorithms can be trained to identify anomalies and predict risks using historical examples of fraud, errors, or non-compliance. This allows focusing audits on the highest risk transactions.
For text mining, natural language processing can extract key entities and semantic relationships from unstructured data like claims adjusters' notes or call center transcripts. These insights help uncover suspicious activity.
Furthermore, as models detect new patterns, those can be incorporated back into the training data through human-in-the-loop workflows. This allows auditing algorithms to continuously improve with more examples over time.

Real-World Examples
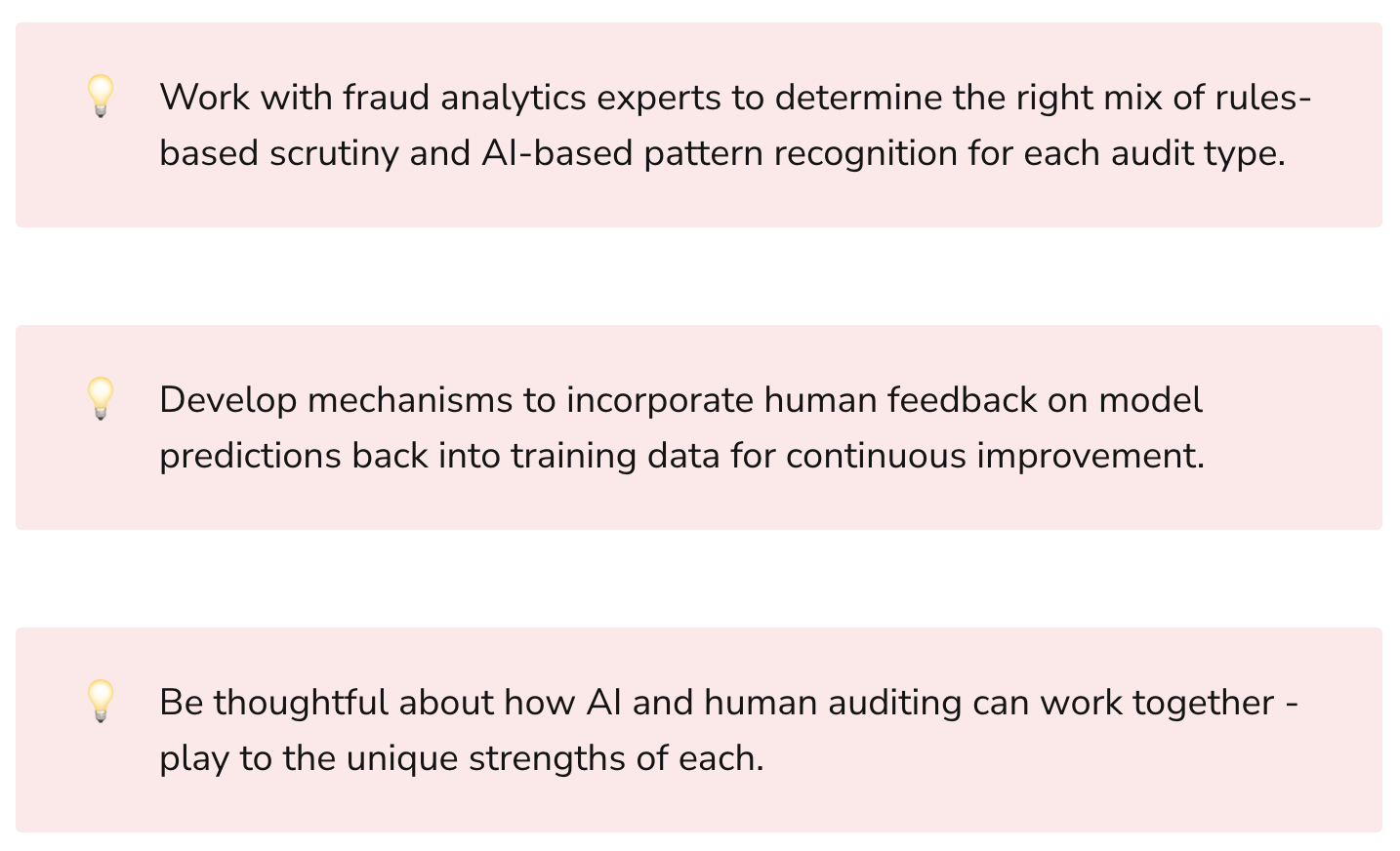
While the technologies powering AI-driven auditing are complex, the practical applications are understandable. Here are a few examples of how analytics can be applied to streamline common auditing scenarios:
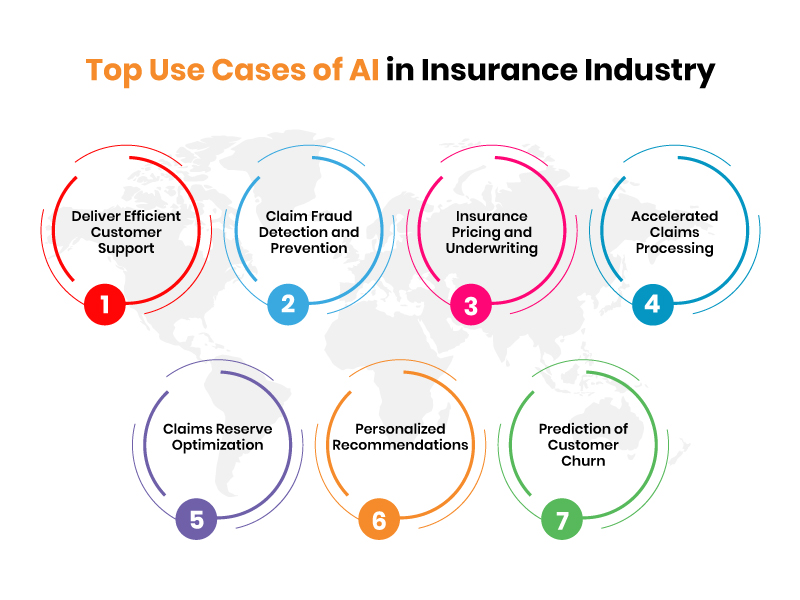
Claims Auditing
- Scan claims data to flag outliers in terms of cost or duration, which may require further review
- Detect associations between claims adjusters and vendors, doctors, or locations that could indicate kickbacks or fraud
- Compare claims against medical records, police reports, and other data to identify discrepancies
- Review claims notes using NLP to catch suspicious or deceptive language
Underwriting Auditing
- Model risk based on historical underwriting data to prioritize audits of high-risk policies
- Verify applicant details like income, credit score, licenses, and other credentials against external databases
- Use geospatial analysis to check if property details match locations
- Scan for suspicious patterns like frequent policy changes or cancellations
Agent Auditing
- Analyze sales patterns to catch churning, twisting, or other questionable practices
- Review agent communications and call transcripts using NLP to identify deceptive language
- Cross-reference transactions across agents to uncover collusion or fraud
- Monitor outlier behaviors like extremely high sales volumes or transaction sizes
Compliance Auditing
- Check policies against regulations like max coverage amounts, required disclosures, etc.
- Scan documents to ensure proper signatures, formatted dates, and other compliance artifacts
- Detect changes in codes, prices, or other data indicating improper modifications
- Review communications across channels for prohibited practices
The key is combining data from across the organization to recreate a complete picture for auditors to review. This expands visibility beyond limited samples while automating manual steps.
Challenges to Consider
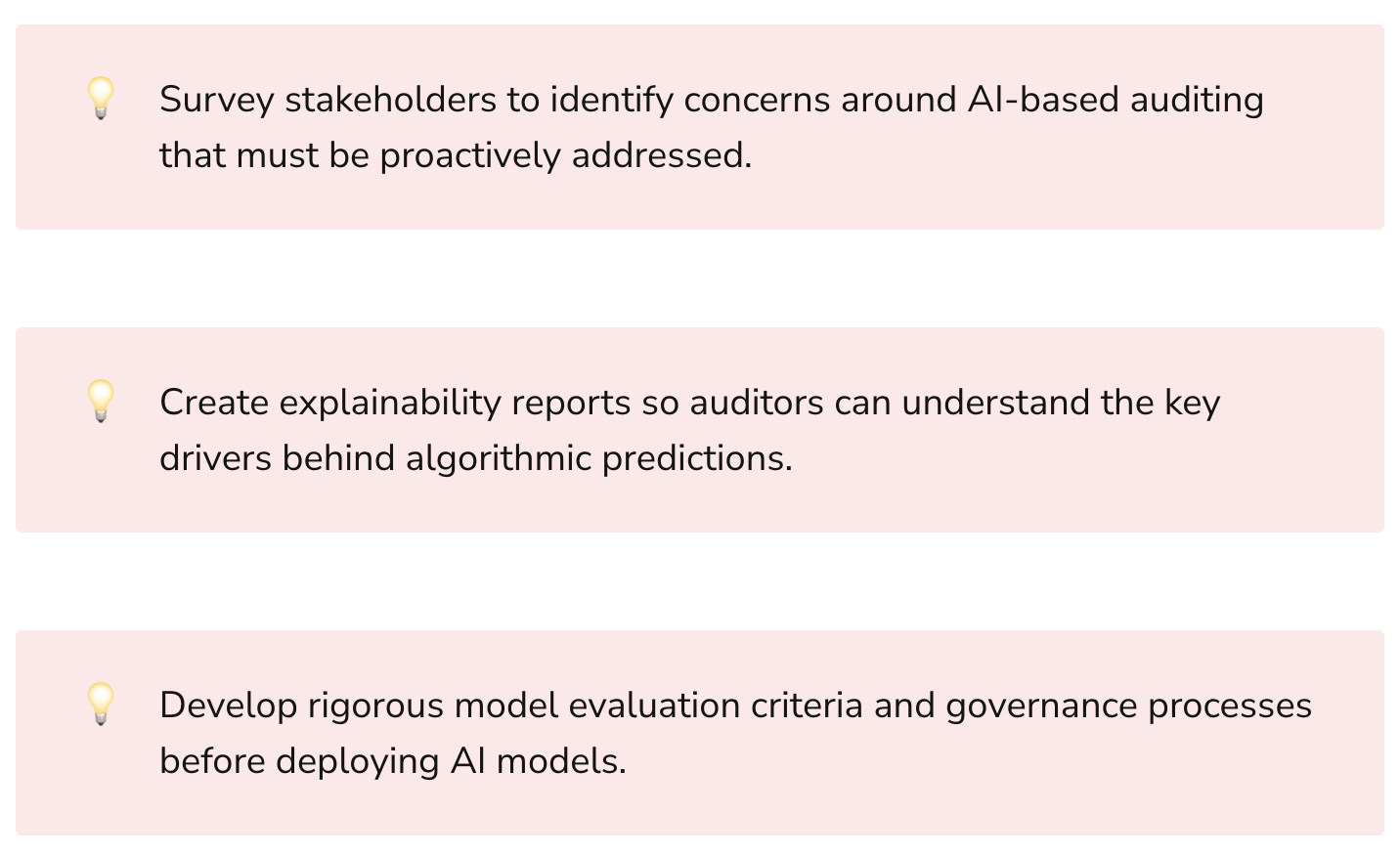
Of course, there are challenges to consider when implementing AI-driven auditing:
- Legacy insurance systems often lack APIs and have data locked in silos. IT teams may need to invest in integration.
- Auditing algorithms require massive training datasets to be accurate. Data collection and annotation is an upfront time investment.
- AI models can detect complex patterns, but explainability is still limited. Auditors need to interpret results.
- Workflows should support seamless hand-offs between algorithms flagging issues and humans investigating further.
- As models improve over time, there need to be mechanisms to track, evaluate, and manage AI-driven decisions across the organization.
While the technology is maturing quickly, overcoming these challenges requires upfront work and continuous governance. The good news is that advanced analytics translate directly into bottom line impact, which provides the business motivation for insurance companies to evolve.

Starting Small and Scaling Up
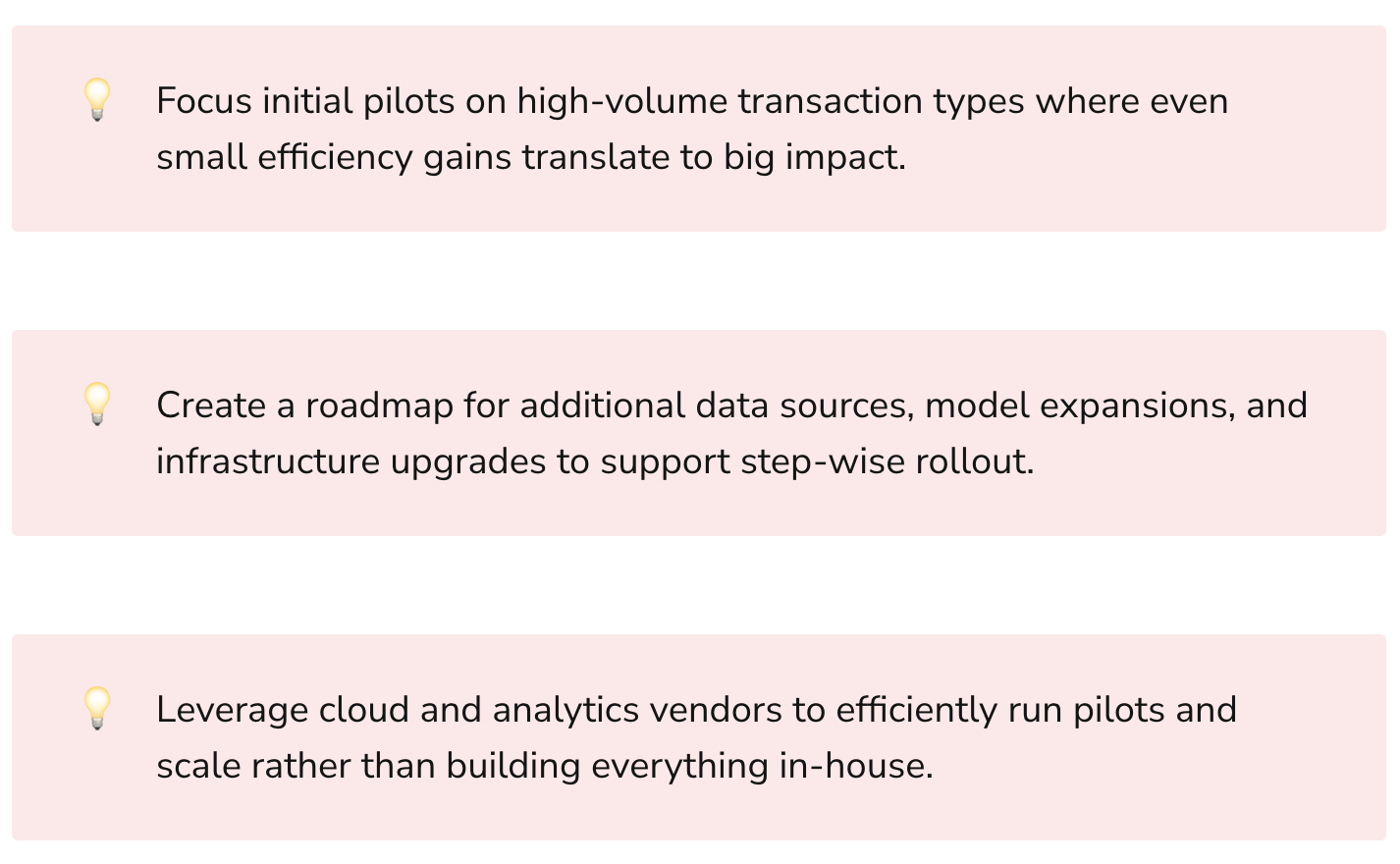
Given the upfront costs, insurance companies should start small with pilots focused on common pain points:
- Automate data extraction and harmonization for a specific process like underwriting audits
- Prototype risk models to prioritize high-risk samples for further auditing
- Implement NLP for reviewing free-text fields like claims notes at scale
- Develop dashboards for auditors with AI-generated alerts and insights
Once proven with a pilot, the capabilities can then be expanded:
- Ingest more data sources like medical records, police reports, etc. to find more connection points
- Train algorithms on larger datasets to improve accuracy and recall
- Apply models across more processes like underwriting, claims, and agent monitoring
- Connect models so outputs from one algorithm feed into others (e.g. use risk scoring to sample claims for NLP)
- Scale via cloud infrastructure to audit every transaction rather than just samples
Adopting this "crawl, walk, run" approach ensures stakeholders see value from AI quickly, while also building for broader impact over time.
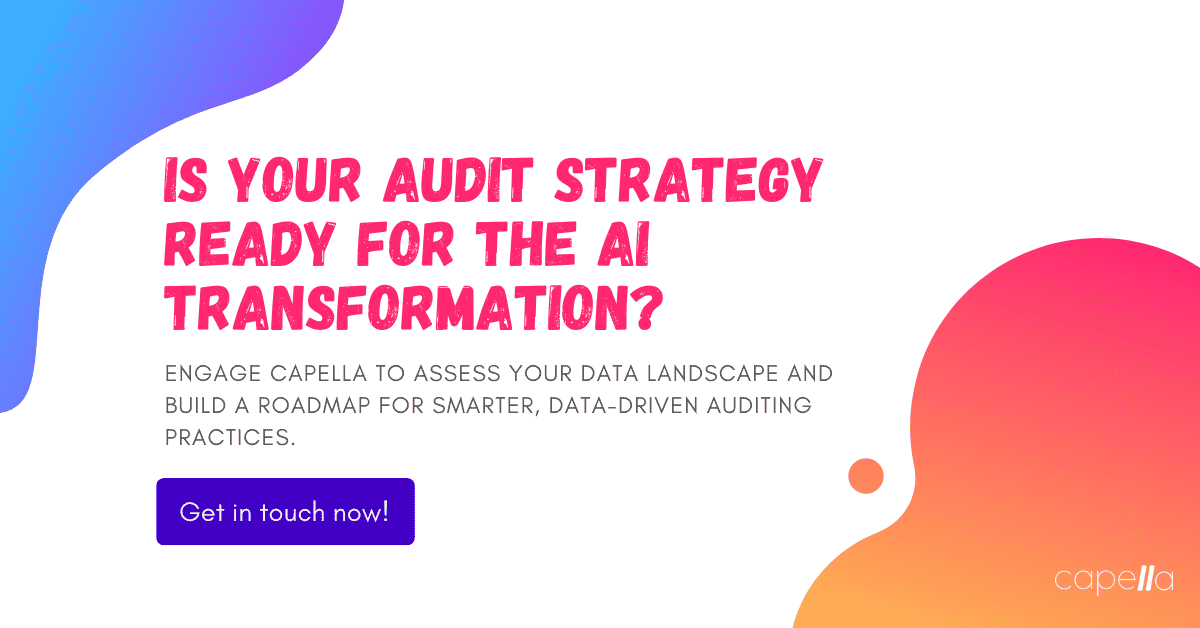
The Intelligent Audit of the Future
By investing in data and AI, insurance audits can transform from slow and tedious sample-based testing to fast and comprehensive continuous auditing. This leads to:
- Improved efficiency by reducing tedious manual steps
- Increased accuracy by expanding scope beyond limited samples
- Faster timelines with issues flagged in real-time vs. long reviews
- Reduced costs by needed less intensive human auditing
- Enhanced compliance with problems caught early before downstream impacts
- Lower fraud via sophisticated detection of suspicious patterns
Just as importantly, streamlining audits through automation empowers auditors, agents, and managers to focus on higher-value analysis and strategic initiatives rather than repetitive tasks.
The data and technology for enabling this transformation already exist at most insurance companies. With the right strategy and governance, insurance leaders can tap into modern AI to reinvent auditing for the better. This will create significant competitive advantage while also improving compliance and reducing risk.

1. What are some common challenges with traditional insurance auditing?
Traditional insurance auditing is very manual, relying on sampling, paperwork, and human judgment. This leads to several challenges:
- Audits are extremely time consuming - compiling documentation can take weeks before reviews even begin. Teams get bogged down gathering and processing files.
- Accuracy suffers from small sample sizes - only reviewing a subset of transactions means errors or fraud can be missed.
- There is potential for bias and inconsistency since audits depend heavily on individual auditor's discretion.
- The focus is on backward looking reviews versus continuous, real-time monitoring for issues. Problems get identified long after the fact.
- With sampling approaches, the same items may get reviewed repeatedly while other items are rarely checked. There are gaps in coverage.
- The overall process is expensive due to the labor intensity and need for specialized expertise. Costs continue rising as transaction volumes grow.
2. Where is AI already being applied for insurance auditing today?
Some common use cases of AI in auditing include:
- Using natural language processing to review claims notes, call center logs, and other unstructured text data at scale to identify suspicious activity.
- Building predictive models using past examples of fraud to automatically flag high risk transactions for further review.
- Analyzing networks and relationships in claims data to detect collusion between vendors, doctors, and claimants.
- Scanning documents to verify signatures, dates, required disclosures, or other compliance artifacts.
- Geospatial analysis on property data to identify discrepancies in locations or details.
- Anomaly detection on financial transactions, claims patterns, or sales activity to catch outliers.
3. What types of data are required to implement AI-driven auditing?
Some important data sources for AI auditing include:
- Historical audit reports categorizing past issues to train algorithms.
- Transactional data from policy admin and claims systems to model patterns.
- External datasets like medical records, weather data, demographics etc. to provide greater context.
- Unstructured data like claims notes, emails, call logs to process with NLP.
- Relationship graphs linking policyholders, agents, adjusters, providers etc.
- Past examples of fraud, compliance breaches, billing errors to build predictive models.
4. What types of AI approaches are best suited for insurance auditing?
Some proven AI techniques for insurance auditing include:
- Supervised learning using past audits to train predictive models to identify risks.
- Unsupervised learning like clustering and anomaly detection to find outliers.
- Text mining using NLP to extract insights from unstructured data.
- Graph algorithms to detect networks and relationships indicative of collusion.
- Regression algorithms to predict expected values and flag outliers.
The choice depends on the specific process being audited and the data available. A combination of different techniques is usually needed for comprehensive auditing.
5. How can insurance companies start small but build towards enterprise-wide AI auditing over time?
A few key tips:
- Start with high volume transaction types where small improvements translate to major savings.
- Focus initial models on accuracy rather than completeness - prioritize recall over precision.
- Build APIs and data integration upfront with expansion in mind.
- Plan for second stage models to take first stage model outputs as inputs.
- Allow models to continuously retrain as human auditors provide more feedback.
- Allocate a portion of cost savings from early wins to fund expansion.
6. What are important considerations around explainability and transparency for AI models used in auditing?
Explainability is critical for auditing algorithms, given the sensitive nature of the findings. Tactics include:
- Selecting model types such as linear regression and decision trees that are more interpretable.
- Using SHAP or LIME to generate feature importance and contribution scores.
- Retaining human auditors “in the loop” to validate model outputs before formal actions.
- Providing model training and testing set metrics to quantify accuracy, recall, and precision.
- Generating alerts for both the transactions flagged and rules/patterns driving those predictions.
- Comparing AI-driven audits to sampling audits to demonstrate enhanced detection.
7. How can insurers maximize business benefits from AI-driven auditing?
Some tips to maximize benefits include:
- Quantify efficiency gains, cycle time improvements, and cost reductions.
- Highlight risk reduction and avoided losses from enhanced detection.
- Link audit automation to strategic goals like customer satisfaction and retention.
- Enable high value activities by freeing auditors from repetitive tasks.
- Continuously curate training data and learn from algorithm outputs.
- Foster collaboration between fraud teams, IT, and analytics groups.
- Develop consistent metrics and governance across audit types.
8. What are some key challenges to address with stakeholders when implementing AI for auditing?
Some considerations for change management include:
- Get executive buy-in by demonstrating tangible ROI.
- Involve stakeholders like auditors and IT in designing processes.
- Set expectations that AI is a complement rather than a complete replacement for human auditors.
- Address job security concerns through training and by shifting to higher value tasks.
- Create general understanding of model limitations and guardrails.
- Develop designated audit teams to oversee AI models and response protocols.
9. How can insurers ensure AI models comply with regulations and standards for auditing?
Strategies to address compliance include:
- Conducting bias testing and ensuring factors like race and gender are excluded.
- Establishing model risk governance procedures and model validation standards.
- Enabling ability to explain what drives AI-generatedAudit flags for regulators.
- Monitoring outcomes to identify any unacceptable algorithm behavior.
- Maintaining responsibility chains so humans remain accountable for AI.
- Adhering to "Ethical AI" principles around transparency and fairness.
10. What does the future look like for AI-driven auditing in insurance?
The future is very bright. Over time, AI allows:
- Transitioning from periodic to continuous, real time auditing.
- Scaling to audit all transactions versus just samples cost-effectively.
- Further automating repetitive compliance checks through robotic process automation.
- Providing frontline teams "self-service" auditing capabilities on-demand.
- Enabling proactive loss prevention versus just detecting issues reactively.
- Making audits seamless rather than disruptive activities.
The ultimate vision is embedded intelligence that consistently ensures every transaction is compliant, accurate, and risk-free.
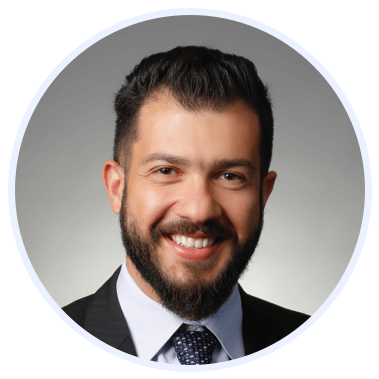
Rasheed Rabata
Is a solution and ROI-driven CTO, consultant, and system integrator with experience in deploying data integrations, Data Hubs, Master Data Management, Data Quality, and Data Warehousing solutions. He has a passion for solving complex data problems. His career experience showcases his drive to deliver software and timely solutions for business needs.