
I'll never forget the day I realized I'd been chasing the wrong data dragon. It was a Tuesday afternoon, and I was staring at a dashboard that was supposed to revolutionize our decision-making process. We'd spent months building it, feeding it with every bit of data we could get our hands on. But something wasn't adding up. The numbers were there, alright, but they weren't telling us anything useful. That's when it hit me: we'd been so focused on collecting data that we'd completely overlooked its quality.

This epiphany led me down a rabbit hole that would change the way I thought about data forever. I discovered a strategy so fundamental, yet so often ignored, that it felt like finding a hundred-dollar bill in an old jacket pocket. It wasn't about having more data, or fancier algorithms, or bigger screens to display our charts. It was about something much simpler, and infinitely more powerful: ensuring the quality of our data.
The Hidden Gem: Data Quality Management
At its core, this strategy is deceptively simple: focus on data quality. It's not as flashy as predictive analytics or as exciting as real-time dashboards, but it's the foundation upon which all other data initiatives are built. And yet, it's often treated as an afterthought, a box to be checked rather than a critical business function.
Let me paint a picture for you. Imagine you're the captain of a ship, setting sail for a distant shore. You've got the latest GPS technology, sophisticated weather prediction models, and a crew trained in the most advanced sailing techniques. But what if your compass is off by just a few degrees? What if your maps are outdated? Suddenly, all that cutting-edge technology becomes useless, potentially leading you far off course.
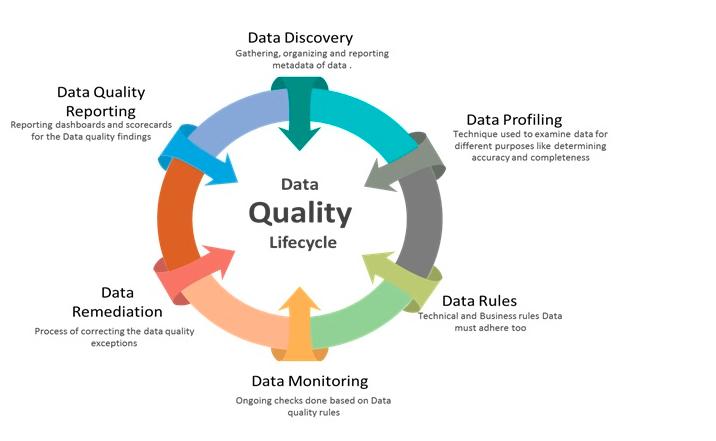
This is precisely the situation many businesses find themselves in when it comes to their data strategy. They invest millions in advanced analytics platforms and hire teams of data scientists, only to feed these systems with data that's inaccurate, incomplete, or inconsistent. The result? Decisions based on flawed insights, missed opportunities, and wasted resources.
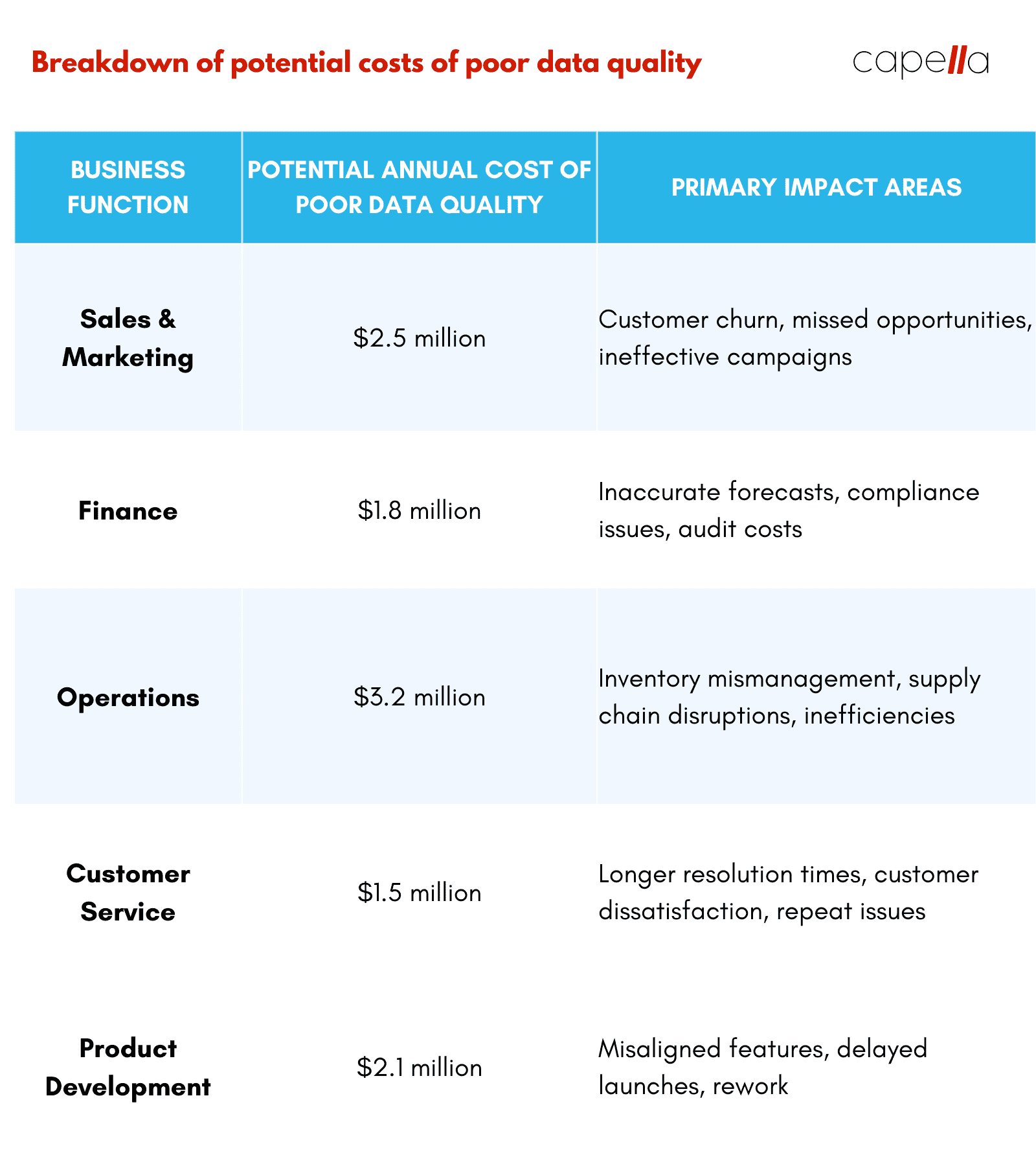
The Real Cost of Poor Data Quality

Before we dive into the specifics of implementing a robust data quality management strategy, let's take a moment to understand the true cost of poor data quality. It's easy to underestimate the impact, but the numbers are staggering.

According to a study by Gartner, poor data quality costs organizations an average of $12.9 million annually. This isn't just a problem for large enterprises – businesses of all sizes suffer from the consequences of bad data. Here's a breakdown of some of the ways poor data quality can impact your bottom line:
- Lost Productivity: Employees spend countless hours searching for the right data, reconciling inconsistencies, and manually correcting errors.
- Missed Opportunities: Inaccurate or incomplete data can lead to poor decision-making, causing businesses to miss out on potential revenue streams or cost-saving measures.
- Regulatory Compliance Issues: In industries with strict data regulations (like healthcare or finance), poor data quality can lead to hefty fines and legal troubles.
- Customer Dissatisfaction: Incorrect customer data can lead to poor service experiences, damaging your brand reputation and customer loyalty.
- Failed Digital Transformation Initiatives: Many digital transformation efforts fail not because of technology limitations, but due to underlying data quality issues.
Let's look at a real-world example to illustrate this point:
Imagine a large retail chain that's implementing a new customer churn prediction model. They've invested heavily in sophisticated machine learning algorithms and hired top-tier data scientists. However, due to poor data quality, their customer records are riddled with inaccuracies.
Some examples of these inaccuracies include:
- Duplicate customer profiles with conflicting information
- Outdated contact details
- Inconsistent formatting of addresses and phone numbers
- Missing or incorrect purchase history data
As a result, their churn prediction model is working with flawed input data. This leads to inaccurate predictions, causing the company to misallocate resources in their customer retention efforts. They might be targeting the wrong customers for retention campaigns or missing out on high-value customers who are at risk of churning.
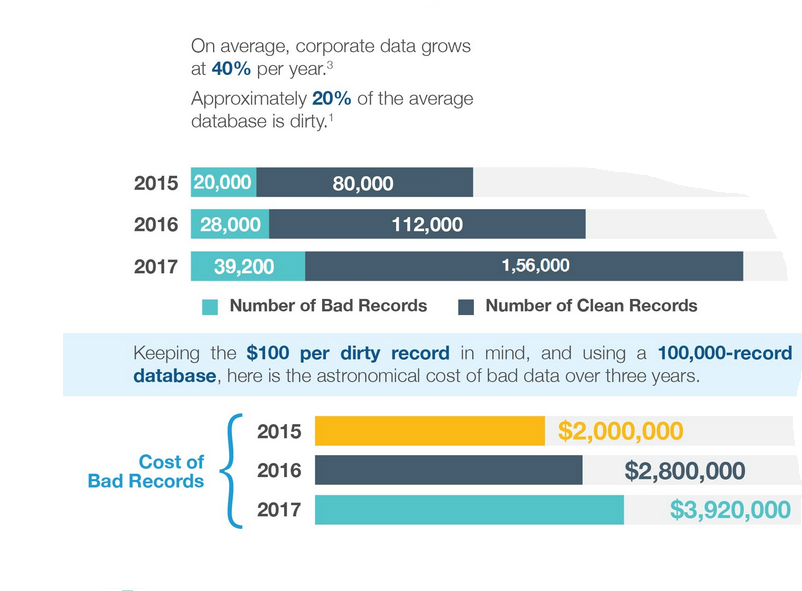
The consequences? Wasted marketing spend, lost revenue from churned customers who weren't identified, and a general lack of trust in data-driven decision making across the organization.
The Million-Dollar Strategy: Implementing Data Quality Management
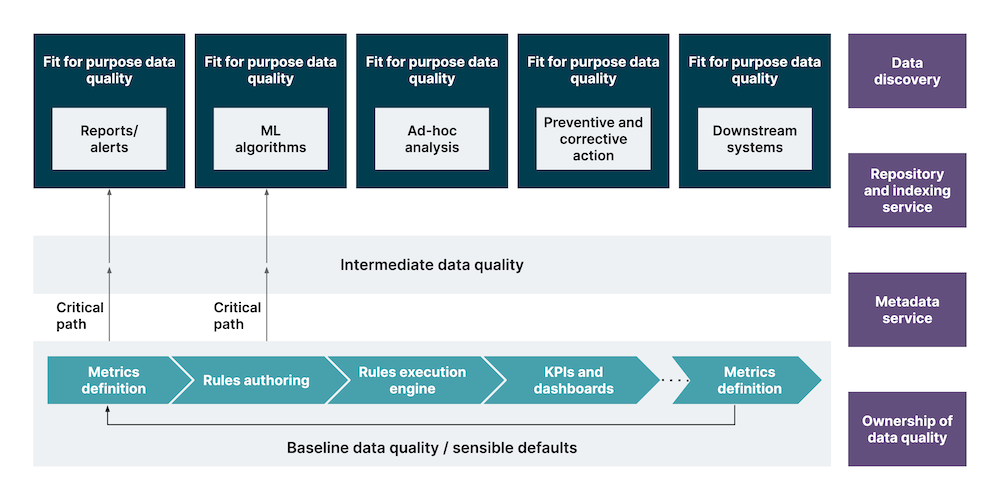
Now that we understand the cost of poor data quality, let's dive into the strategy that can potentially save (or make) your organization millions: implementing a comprehensive data quality management program.
1. Establish a Data Governance Framework

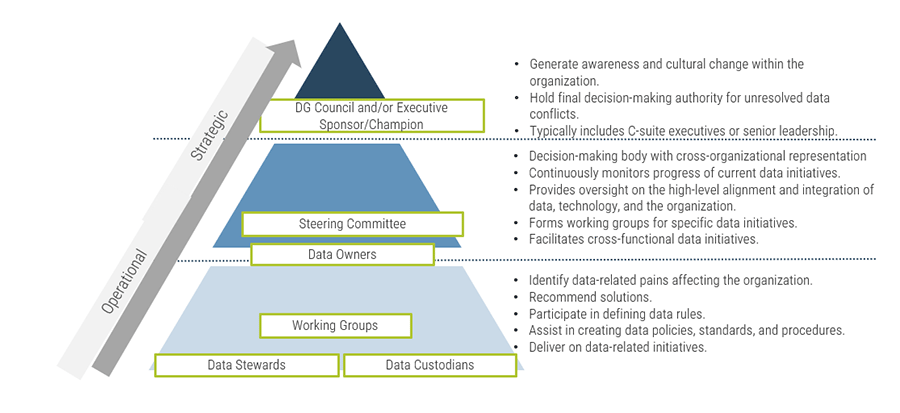
The first step in any data quality initiative is to establish a clear governance structure. This involves:
- Defining Data Ownership: Assign responsibility for different data domains to specific individuals or teams.
- Creating Data Quality Metrics: Develop clear, measurable criteria for assessing data quality.
- Implementing Data Quality Policies: Establish guidelines for data entry, maintenance, and usage across the organization.
Here's an example of how you might structure your data governance team:
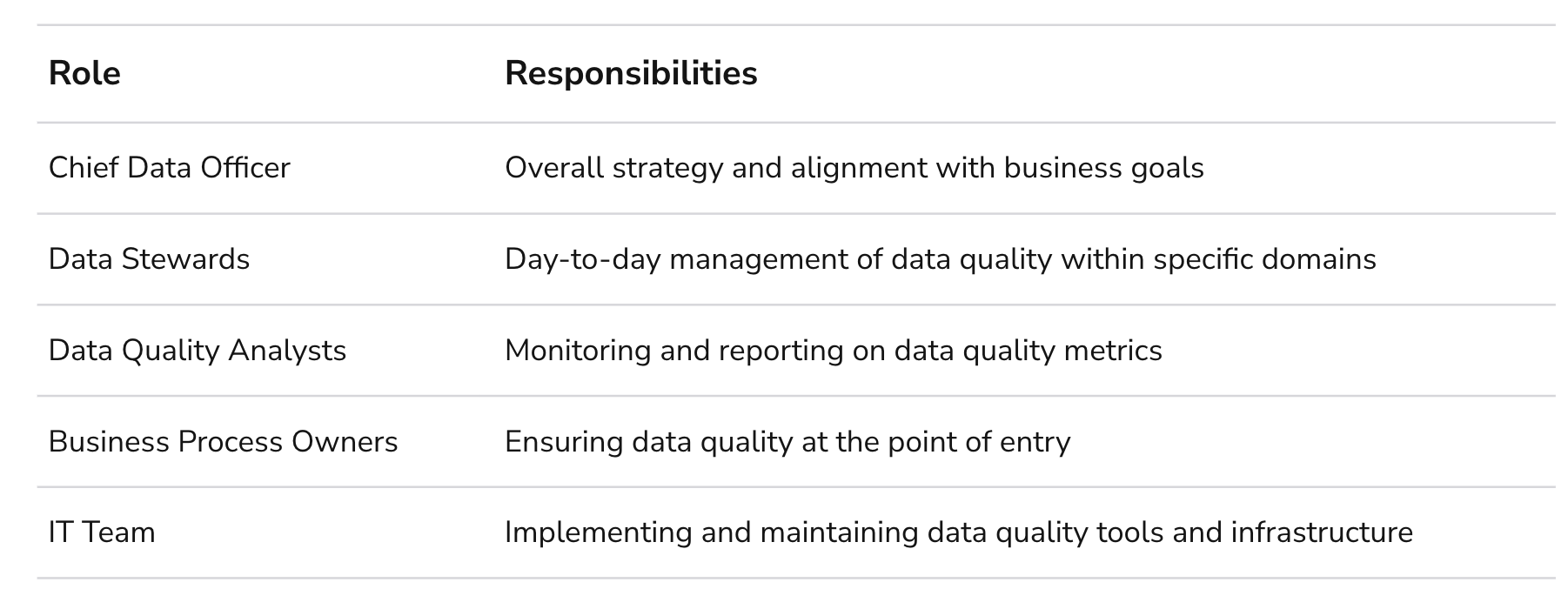
2. Implement Automated Data Profiling and Monitoring
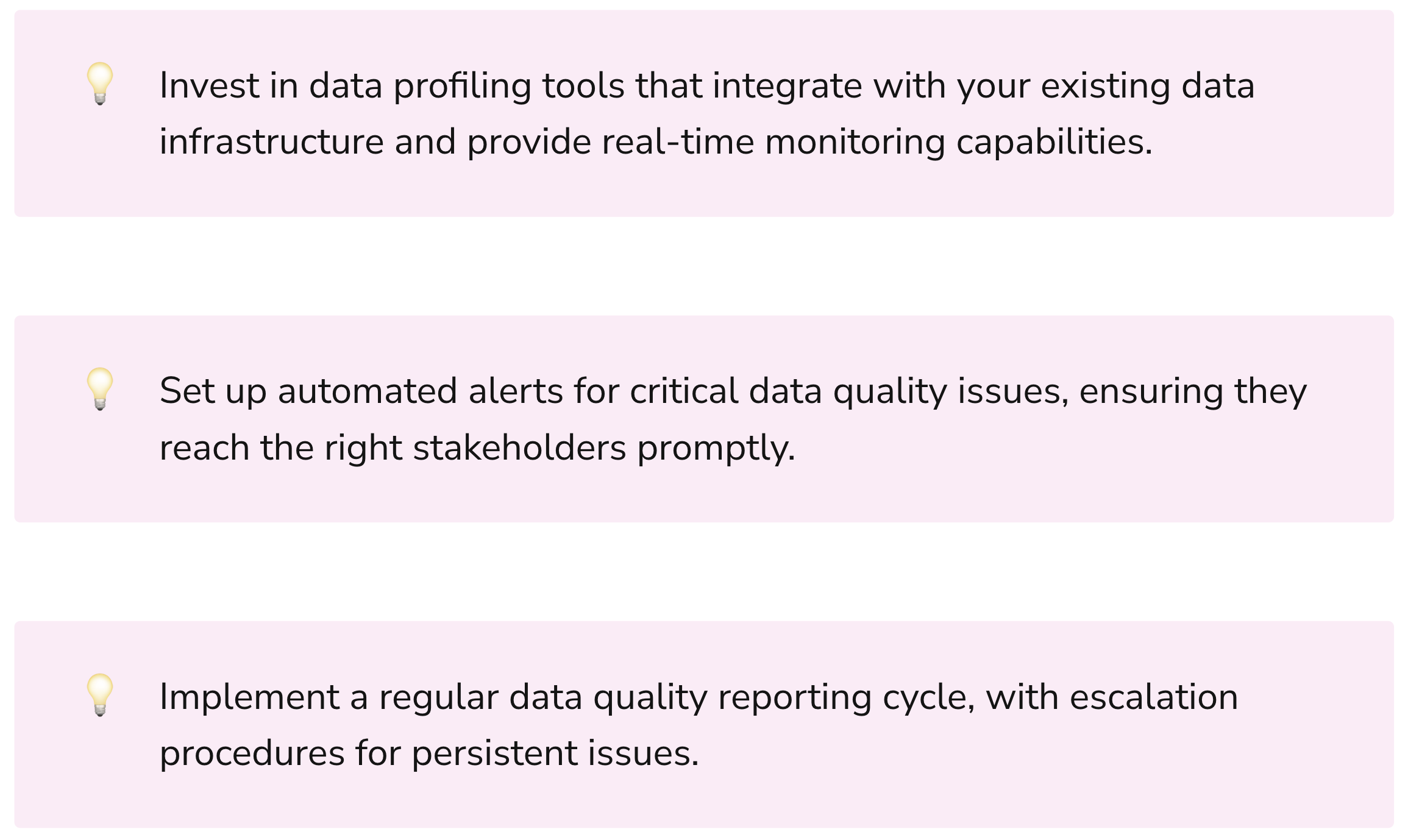
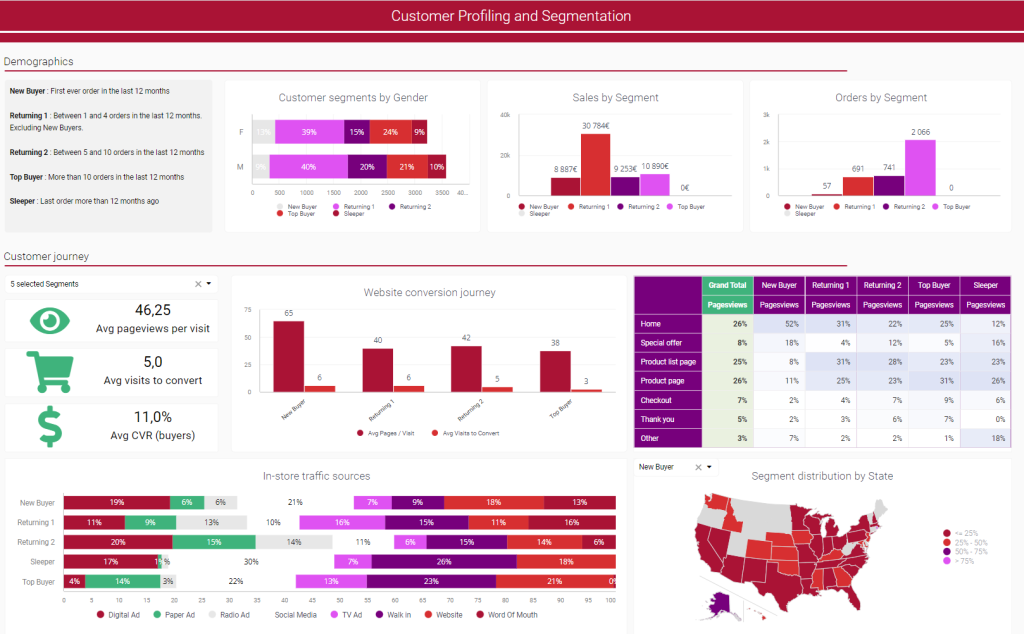
Manual data quality checks are time-consuming and prone to human error. Implementing automated data profiling and monitoring tools can help you catch issues early and maintain consistent data quality over time.
For instance, you might implement a system that automatically scans your customer database nightly, flagging potential issues such as:
- Duplicate records
- Missing critical fields (e.g., email addresses for digital marketing campaigns)
- Outliers in numerical fields (e.g., unrealistic order values)
- Inconsistent formatting (e.g., phone numbers in various formats)
These automated checks can alert relevant team members to issues before they cascade into larger problems, allowing for proactive data management rather than reactive firefighting.
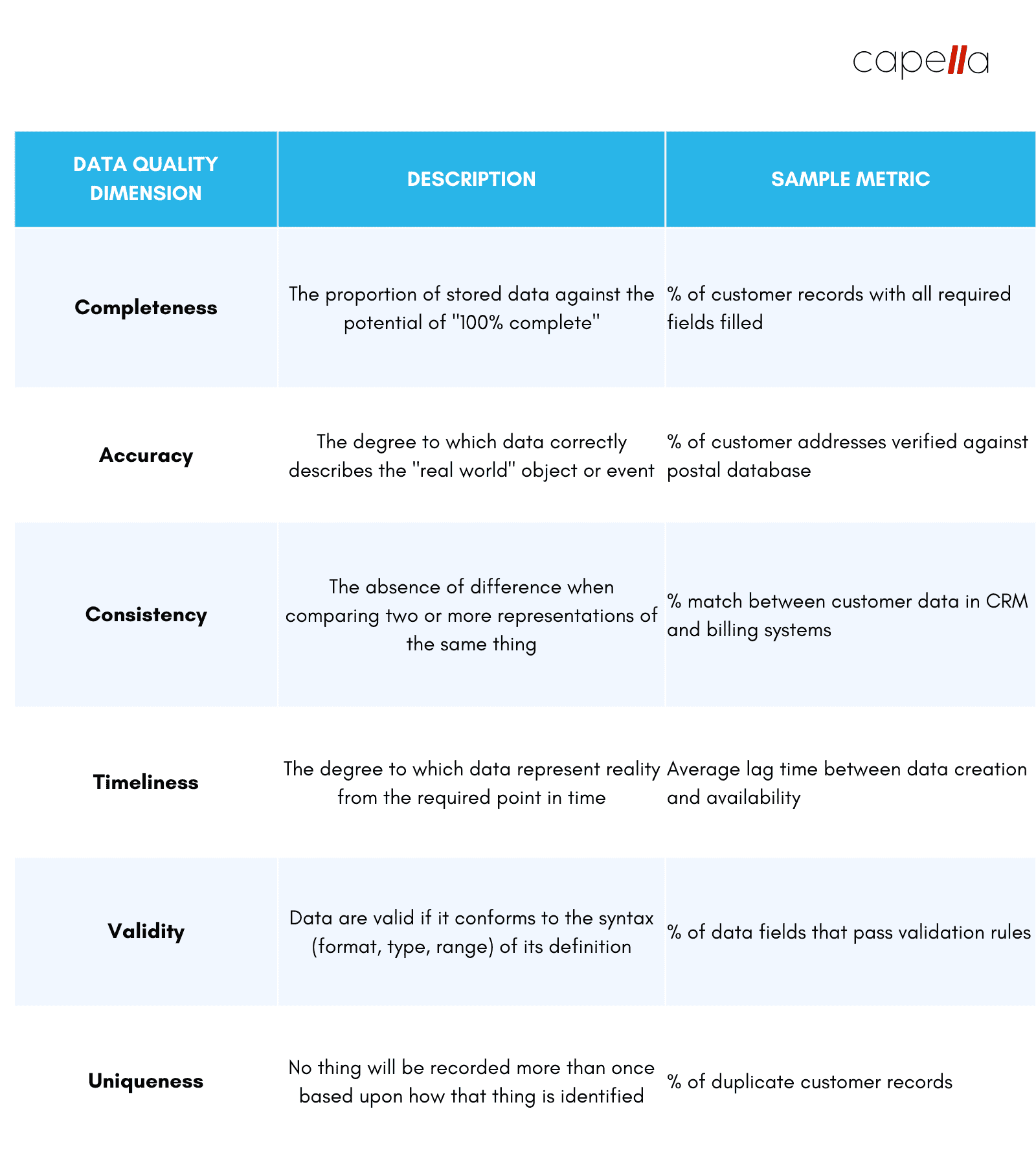
3. Develop a Data Quality Scorecard


Create a scorecard that provides a quick, visual representation of your data quality across different dimensions. This can help you track progress over time and identify areas that need immediate attention.
Here's an example of what a data quality scorecard might look like:

This scorecard could be updated monthly and shared with key stakeholders, providing a clear, at-a-glance view of your organization's data health.
4. Implement Data Cleansing and Enrichment Processes


Once you've identified data quality issues, you need processes in place to clean and enrich your data. This might involve:
- Deduplication of records
- Standardization of data formats
- Validation against external sources
- Filling in missing values
For example, in a B2B context, you might implement a process where company names are validated and enriched using a trusted third-party business database. This could help standardize company names (e.g., "IBM" vs "International Business Machines"), fill in missing industry classifications, and add additional valuable data points like company size or revenue.
5. Foster a Data-Quality-Centric Culture

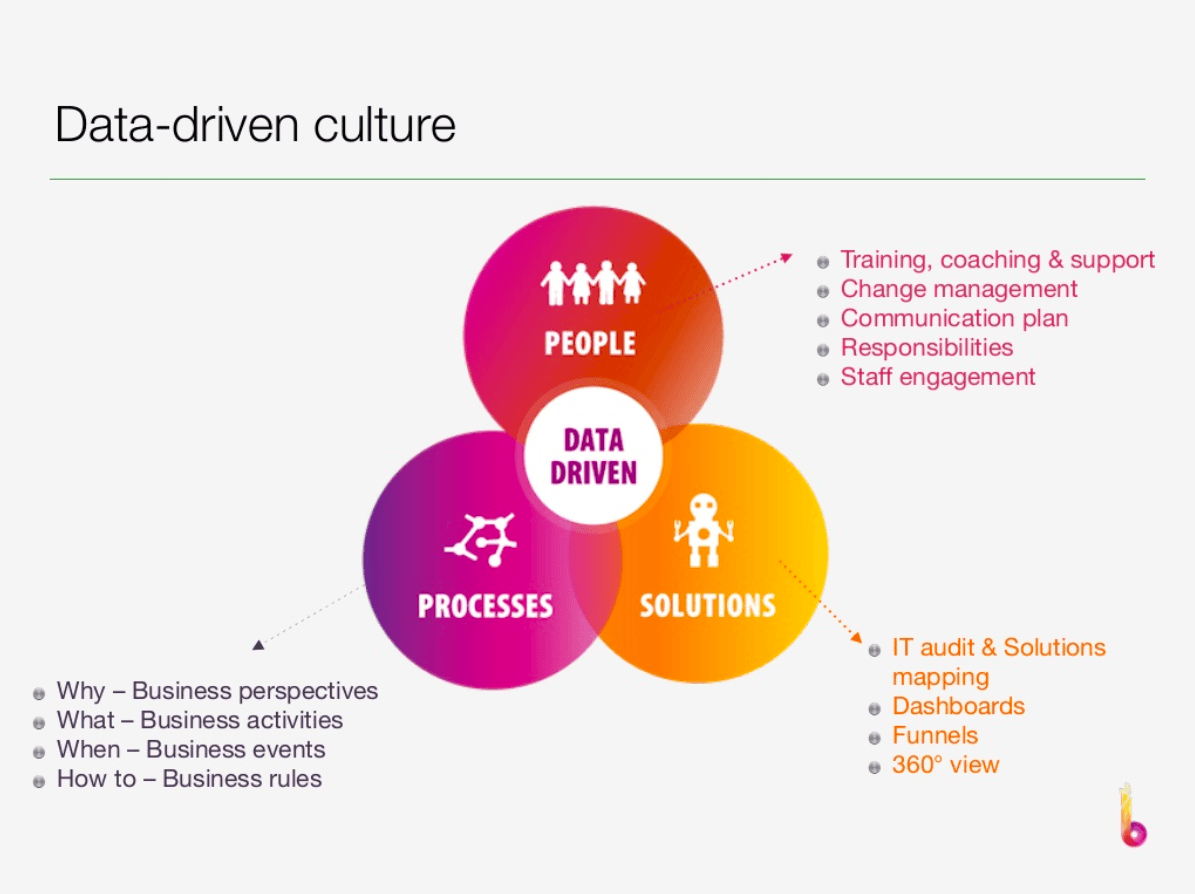
Perhaps the most challenging – and most crucial – aspect of this strategy is fostering a culture where everyone in the organization understands the importance of data quality. This involves:
- Training Programs: Educate employees at all levels about data quality principles and best practices.
- Incentive Structures: Tie data quality metrics to performance evaluations and bonuses.
- Regular Communication: Share data quality success stories and challenges across the organization.
Consider implementing a "Data Quality Champion" program, where employees from various departments are trained in data quality best practices and tasked with promoting these practices within their teams. This can help embed data quality consciousness throughout the organization.
The Million-Dollar Impact: Real-World Success Stories
To truly appreciate the power of this strategy, let's look at some real-world examples of organizations that have reaped significant benefits from focusing on data quality:
- Global Pharmaceutical Company: By implementing a comprehensive data quality management program, this company was able to reduce clinical trial errors by 25%, leading to faster drug approvals and an estimated $40 million in annual savings.
- Major Retailer: After cleaning and consolidating their customer data, this retailer saw a 15% increase in the effectiveness of their targeted marketing campaigns, resulting in an additional $25 million in revenue over the course of a year.
- Financial Services Firm: By improving the quality of their risk assessment data, this firm was able to more accurately price their products, leading to a 10% increase in profitability for their loan portfolio.

Overcoming Common Challenges
While the benefits of a robust data quality management strategy are clear, implementing such a program is not without its challenges. Here are some common obstacles you might face, and strategies to overcome them:
- Lack of Executive Buy-In:
- Solution: Build a business case using concrete examples of how poor data quality is impacting the bottom line. Use the data quality scorecard to demonstrate progress and ROI over time.
- Siloed Data and Processes:
- Solution: Implement a centralized data management platform and establish cross-functional data quality teams to break down silos.
- Legacy Systems:
- Solution: Start with small pilot projects to demonstrate the value of data quality initiatives. Use these successes to build momentum for larger system overhauls.
- Resource Constraints:
- Solution: Prioritize data quality efforts based on business impact. Focus on the most critical data elements first and expand over time.
- Resistance to Change:
- Solution: Invest in change management and training programs. Celebrate early wins and share success stories to build enthusiasm.
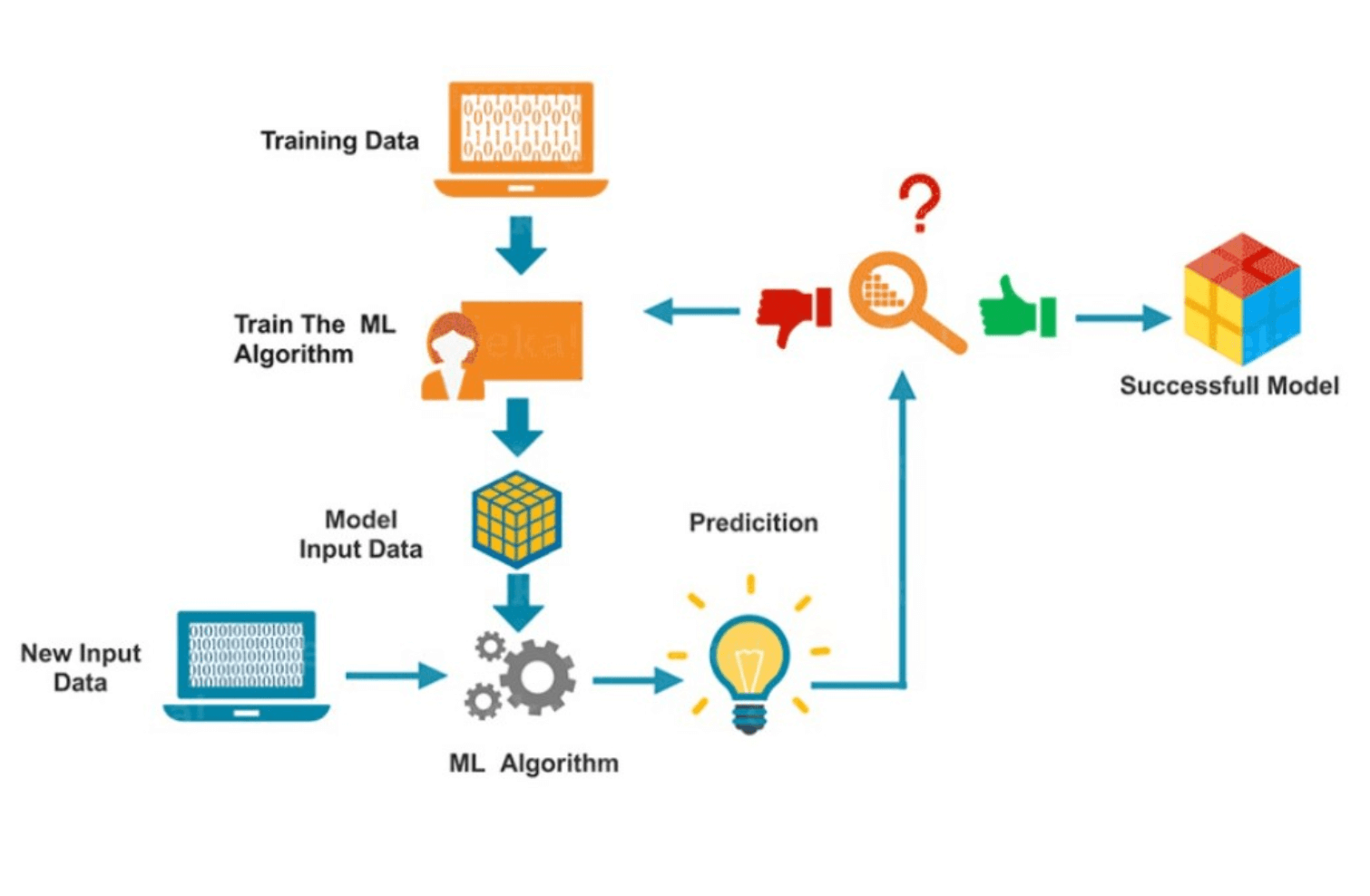
Looking to the Future: Data Quality in the Age of AI and Machine Learning
As we look to the future, the importance of data quality will only increase. With the rise of artificial intelligence and machine learning, the adage "garbage in, garbage out" has never been more relevant. High-quality data is the fuel that powers these advanced technologies.
Consider a scenario where an AI system is being used to optimize supply chain operations. The system relies on historical data about supplier performance, demand patterns, and logistics costs to make predictions and recommendations. If this underlying data is inaccurate or inconsistent, the AI's recommendations could lead to costly mistakes – overstocking perishable goods, underestimating demand during peak seasons, or choosing unreliable suppliers.
On the other hand, with high-quality data, such an AI system could revolutionize supply chain management, predicting demand with uncanny accuracy, optimizing inventory levels, and identifying cost-saving opportunities that human analysts might miss.
This is just one example of how data quality acts as a force multiplier for advanced technologies. As AI and machine learning become more prevalent across industries, organizations with a strong foundation in data quality will be poised to reap outsized benefits from these technologies.
Conclusion: The Million-Dollar Opportunity
In the rush to adopt the latest data technologies, it's easy to overlook the foundational importance of data quality. But as we've seen, a focus on data quality can yield returns that far exceed the investment required.
By implementing a comprehensive data quality management strategy, you're not just avoiding costly mistakes – you're positioning your organization to fully leverage the power of data in driving business success. From more accurate predictive models to more effective marketing campaigns, the benefits touch every aspect of your business.
Remember, in the world of data, quality trumps quantity every time. It's not about having the most data, but about having the right data – accurate, consistent, and trustworthy. This is the true million-dollar strategy that too many organizations are ignoring.
As you embark on your data quality journey, keep in mind that this is not a one-time project, but an ongoing commitment. The data landscape is constantly evolving, and your data quality strategies must evolve with it. But with the right approach, the rewards – both financial and operational – can be truly transformative.
In the end, the question isn't whether you can afford to invest in data quality. The real question is: can you afford not to?
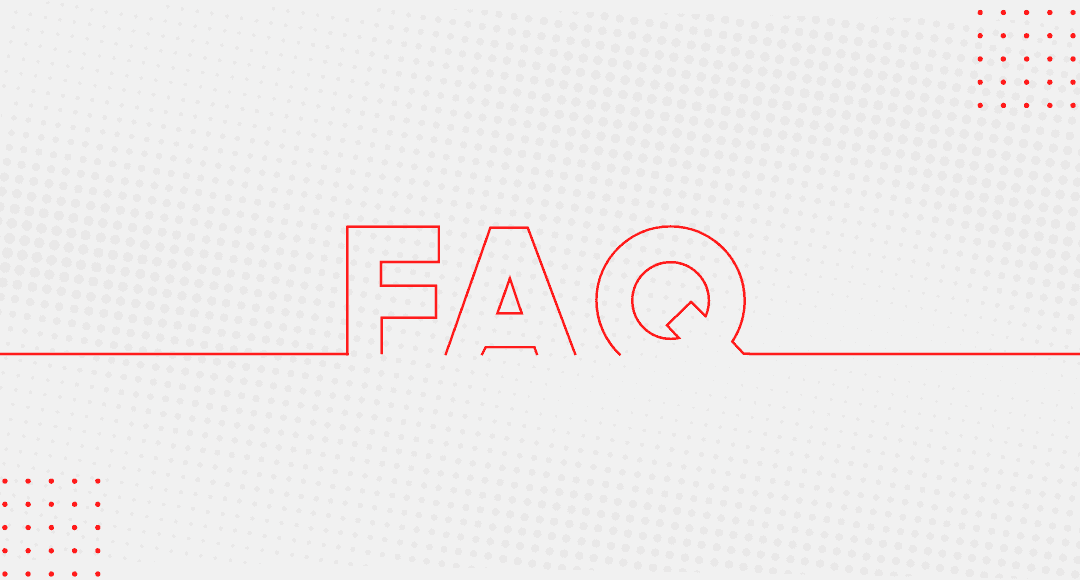
Q1: What exactly is data quality management?
A: Data quality management is the ongoing process of maintaining the accuracy, completeness, consistency, and reliability of data throughout its lifecycle. It involves strategies and practices to ensure that data remains fit for its intended uses in operations, decision-making, and planning.
Q2: How does poor data quality impact my bottom line?
A: Poor data quality can significantly impact your bottom line through increased operational costs, missed opportunities, poor decision-making, customer dissatisfaction, and regulatory compliance issues. Studies suggest that poor data quality costs organizations an average of $12.9 million annually.
Q3: We already have a data team. Isn't that enough?
A: While having a data team is crucial, effective data quality management requires a company-wide approach. It involves establishing governance frameworks, fostering a data-quality culture, and integrating quality checks into all data-related processes across the organization.
Q4: How do I measure the ROI of data quality initiatives?
A: Measure ROI by tracking improvements in key performance indicators such as reduced error rates, increased operational efficiency, enhanced customer satisfaction, and improved decision-making accuracy. Quantify the time and resources saved, as well as any increase in revenue or cost savings directly attributable to better data quality.
Q5: What's the first step in implementing a data quality management strategy?
A: The first step is to conduct a comprehensive data quality assessment. This involves profiling your data, identifying critical data elements, and establishing baseline quality metrics. This assessment will help you understand your current data landscape and prioritize improvement efforts.
Q6: How often should we perform data quality checks?
A: Data quality checks should be an ongoing process. Implement real-time monitoring for critical data elements, conduct daily or weekly automated checks for key datasets, and perform comprehensive audits quarterly or annually. The frequency may vary based on the nature of your data and business needs.
Q7: What role does automation play in data quality management?
A: Automation is crucial in maintaining data quality at scale. It helps in continuously monitoring data, detecting anomalies, enforcing data standards, and streamlining cleansing processes. Automated tools can significantly reduce manual errors and ensure consistent application of data quality rules across the organization.
Q8: How do we balance data quality with data security and privacy?
A: Data quality and security are complementary concerns. Implement role-based access controls, data masking, and encryption to protect sensitive information. Ensure that data quality processes adhere to privacy regulations. Remember, high-quality data is inherently more secure, as it's easier to track, manage, and protect.
Q9: What skills does my team need to effectively manage data quality?
A: A mix of technical and soft skills is ideal. Technical skills include data analysis, statistics, database management, and familiarity with data quality tools. Soft skills like communication, problem-solving, and change management are equally important. Consider training programs to upskill your existing team.
Q10: How does data quality management relate to AI and machine learning initiatives?
A: Data quality is fundamental to the success of AI and ML initiatives. High-quality, clean data is essential for training accurate and unbiased models. Poor data quality can lead to flawed insights and unreliable predictions. As you advance into AI and ML, your data quality management practices will need to evolve to meet the more stringent requirements of these technologies.
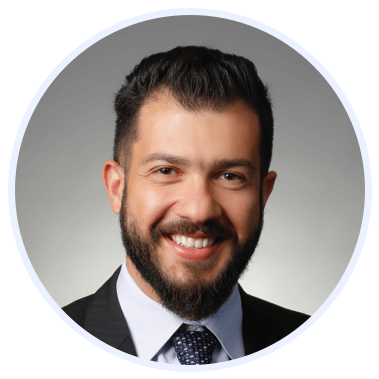
Rasheed Rabata
Is a solution and ROI-driven CTO, consultant, and system integrator with experience in deploying data integrations, Data Hubs, Master Data Management, Data Quality, and Data Warehousing solutions. He has a passion for solving complex data problems. His career experience showcases his drive to deliver software and timely solutions for business needs.