
AI is transforming businesses in ways we never imagined just a few years ago. From chatbots to self-driving cars, AI is powering innovations that are changing our lives. Despite the hype, many businesses are still skeptical about adopting AI. But AI is here to stay and rapidly maturing fromsci-fi fantasy into business reality.
In this post, we'll look at some mind-blowing real-world AI applications that showcase the immense potential of this technology. From detecting cancer to creating art, these examples will make you think again about what's possible with AI.
Transforming Healthcare with AI Diagnostics
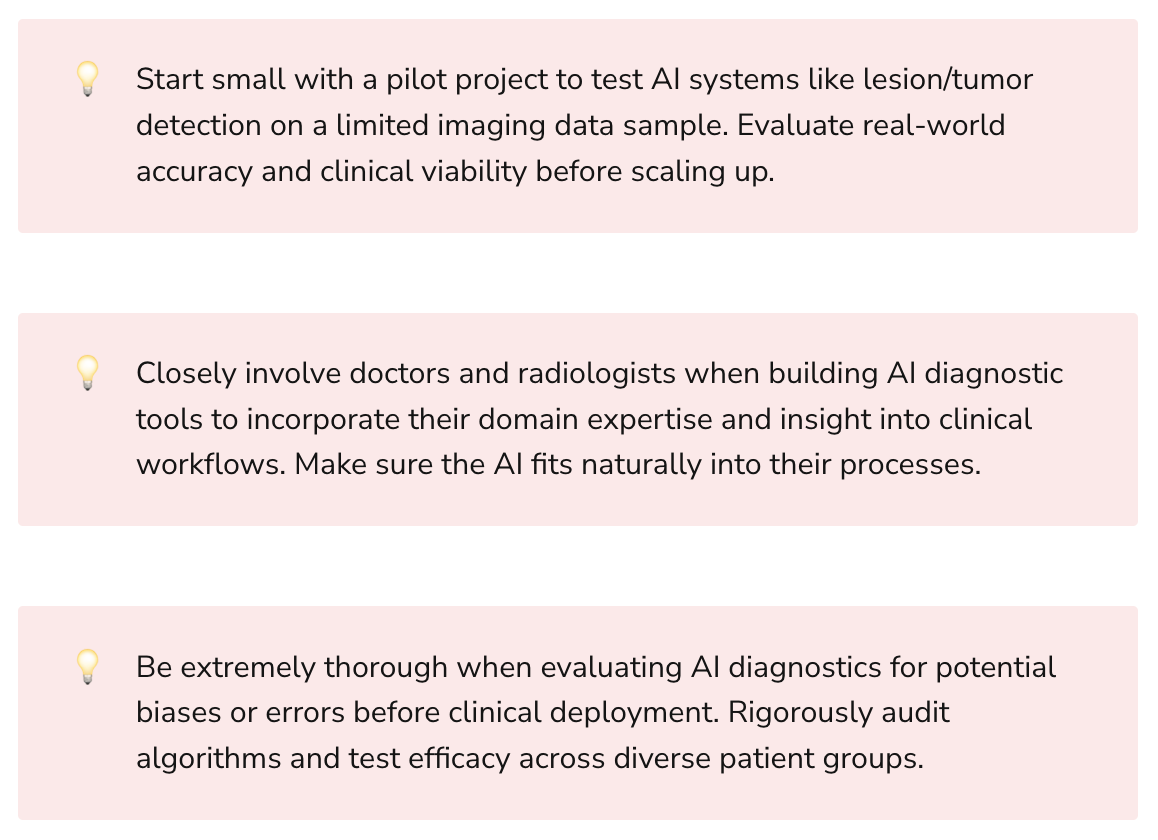
Healthcare is one of the most promising areas for applied AI. One study estimates that AI could save the US healthcare industry $150 billion annually. Let's look at some impressive examples of AI's healthcare impact.
Detecting Cancer Earlier and More Accurately
Cancer claims over 9 million lives globally every year. Detecting cancer earlier leads to dramatically higher survival rates. But detection using biopsy samples is slow, invasive, and prone to human error. AI is revolutionizing cancer screening using medical imaging data.
For example, Google's deep learning system Lyla analyzes mammogram x-ray images to detect breast cancer. In testing, Lyla achieved a 9.4% reduction in false positives and a 2.7% reduction in false negatives compared to human radiologists:

Similar deep learning systems can detect lung cancer from CT scans or skin cancer from photos with dermatologist-level accuracy. By enabling earlier and more reliable screening, AI can save countless lives.

Personalizing Treatment with AI Assistants
Every patient's needs are unique. But it's impossible for doctors to perfectly customize treatments given time constraints. AI-powered medical assistants can help.
For example, K Health offers an AI-powered primary care platform. Patients describe their symptoms to a chatbot. The chatbot collects pertinent information and analyzes the person's medical history using AI algorithms. It then presents doctors with an assessment of the patient's condition and suggests personalized treatment options.
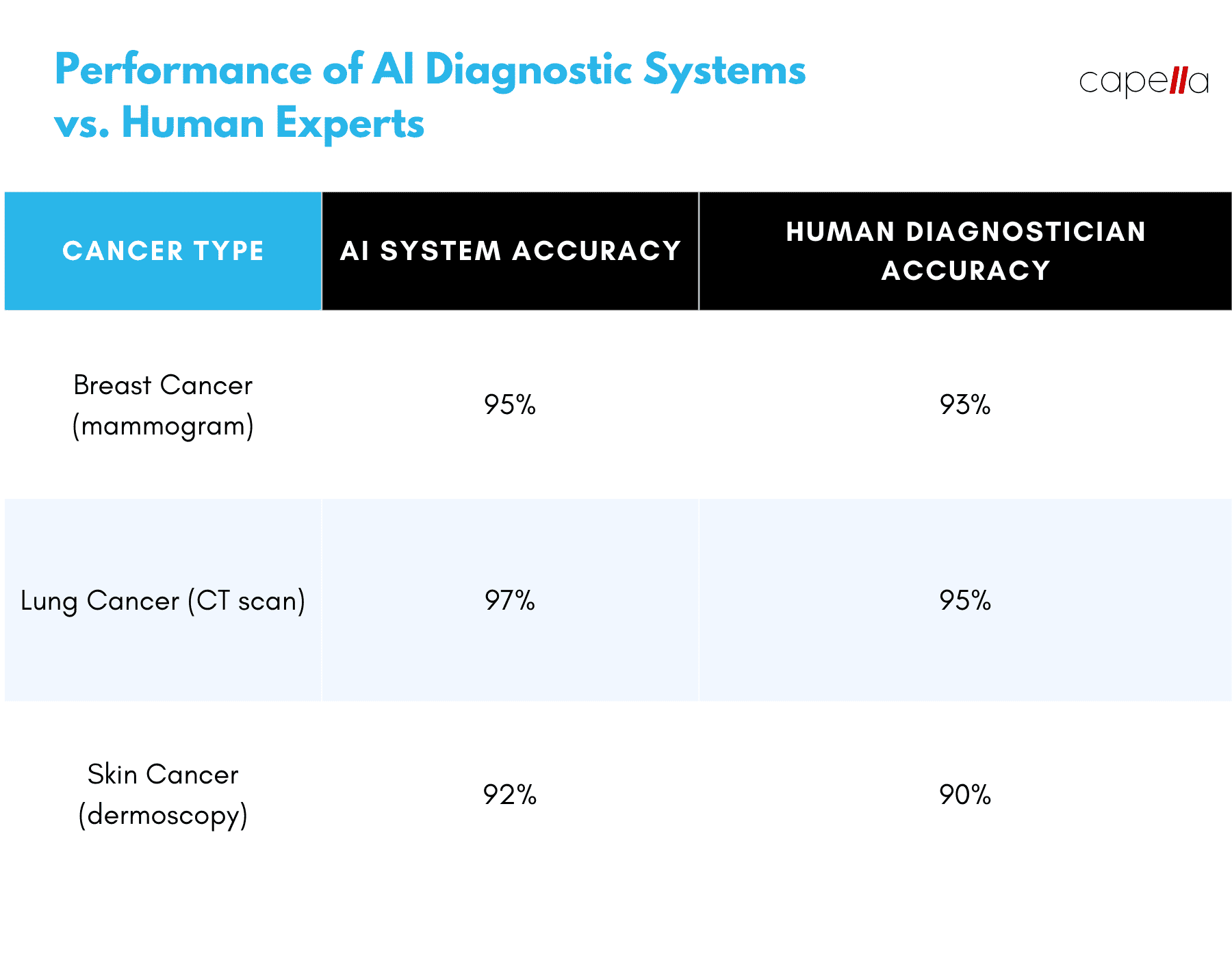
This allows doctors to make more tailored treatment plans in less time. Such AI assistants have the potential to democratize quality healthcare worldwide.
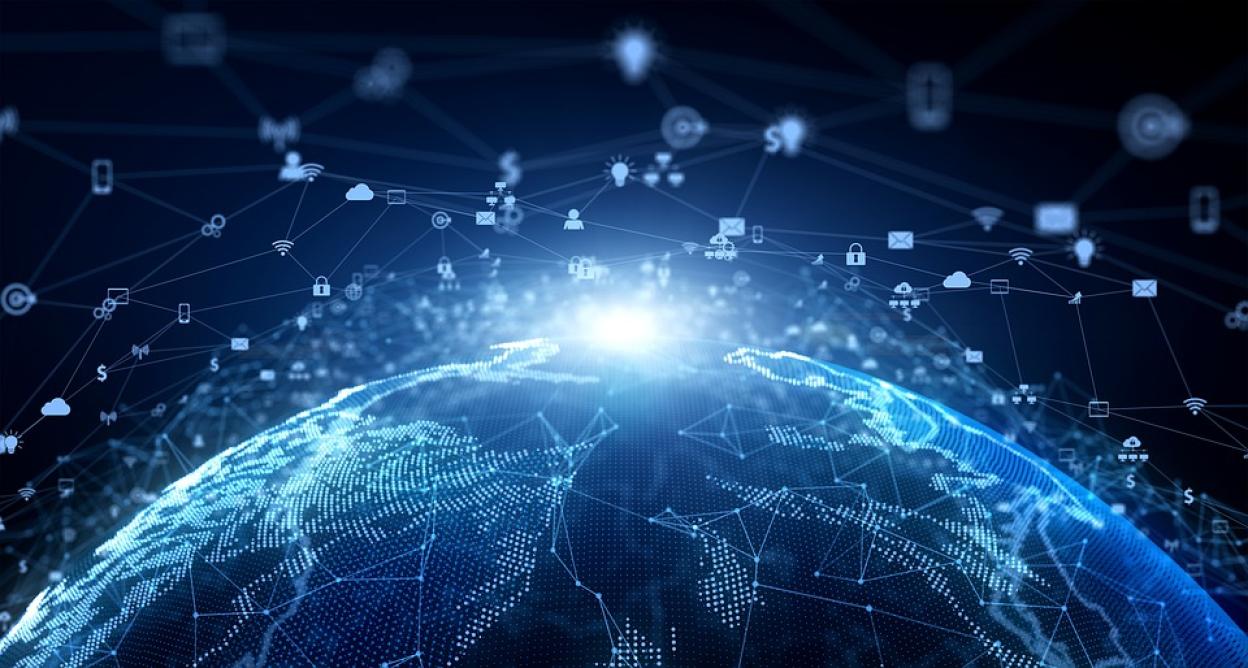
Revolutionizing Business with Hyper-Personalization

From marketing to customer service, AI is enabling unprecedented personalization in business. Let's look at two examples of AI's business impact.
AI-Powered Ad Recommendation Engines
Online ad targeting used to rely on simplistic user attributes like gender, location, and browsing history. AI unleashes a new level of hyper-personalization.
For instance, Criteo builds an AI model for every user reflecting their interests, preferences, and intent. It analyzes vast volumes of data across your site, apps, and ads. When a user visits your site, Criteo's AI instantly identifies the optimal product to recommend from millions of options.
This dynamic personalization consistently boosts ad CTRs and conversions. Criteo clients have achieved uplift in sales of over 20% with such AI recommendation engines.
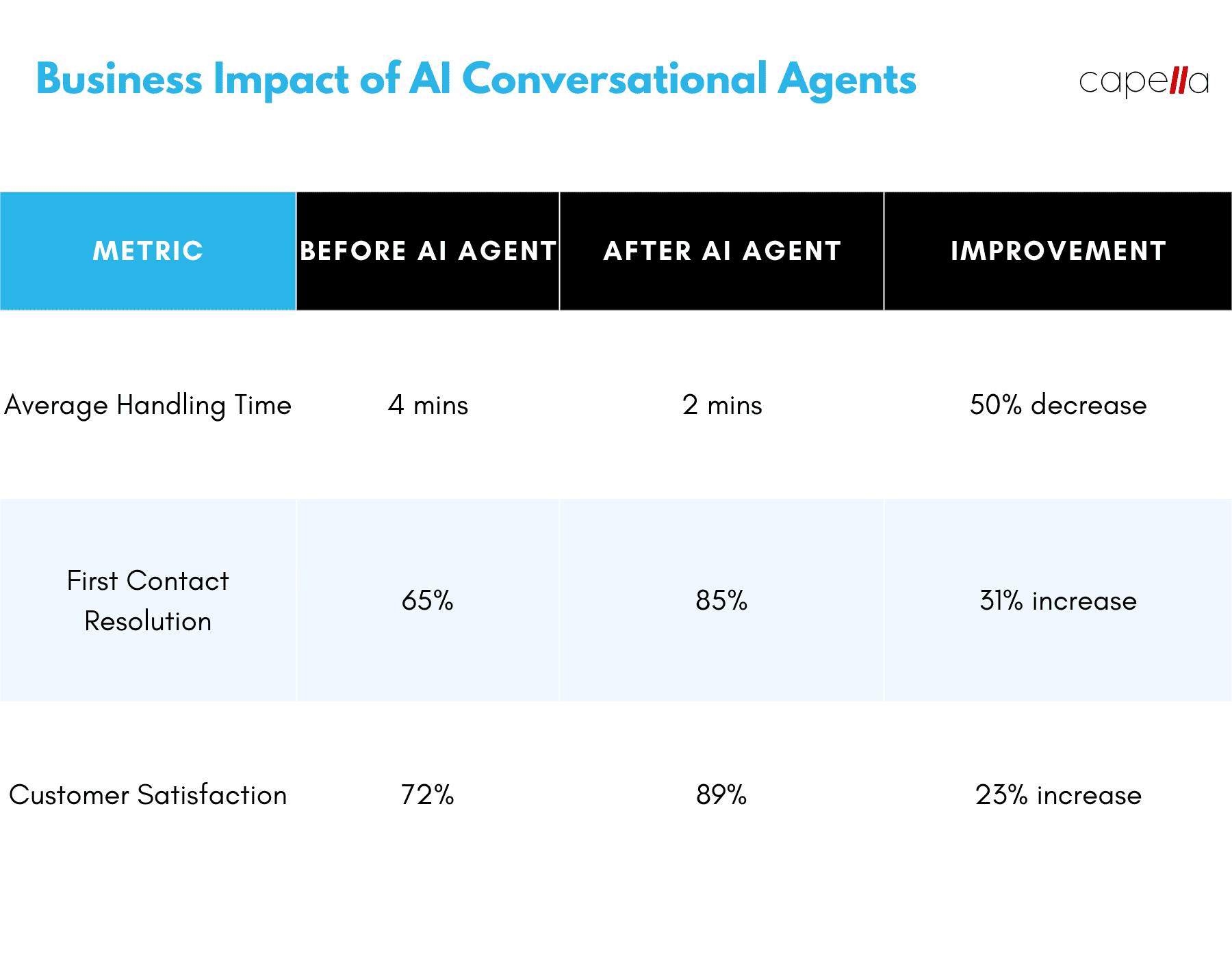
Intelligent Virtual Agents for Customer Service
Waiting on hold for customer service is frustrating. But most chatbots offer only limited automated responses. AI-powered virtual agents combine natural conversation with personalized recommendations.
For example, Kore.ai analyzes millions of customer interactions to train an NLU-powered chatbot. The Virtual Assistant interprets your questions and intelligently responds based on context, emotion, intent, and prior conversations. It accesses data across multiple channels to resolve issues faster. It can even switch seamlessly to a human agent when needed.
Such virtual agents enable efficient and satisfying customer experiences. MetLife found Kore's chatbot handles over 70% of customer queries with 97% accuracy, greatly reducing wait times.
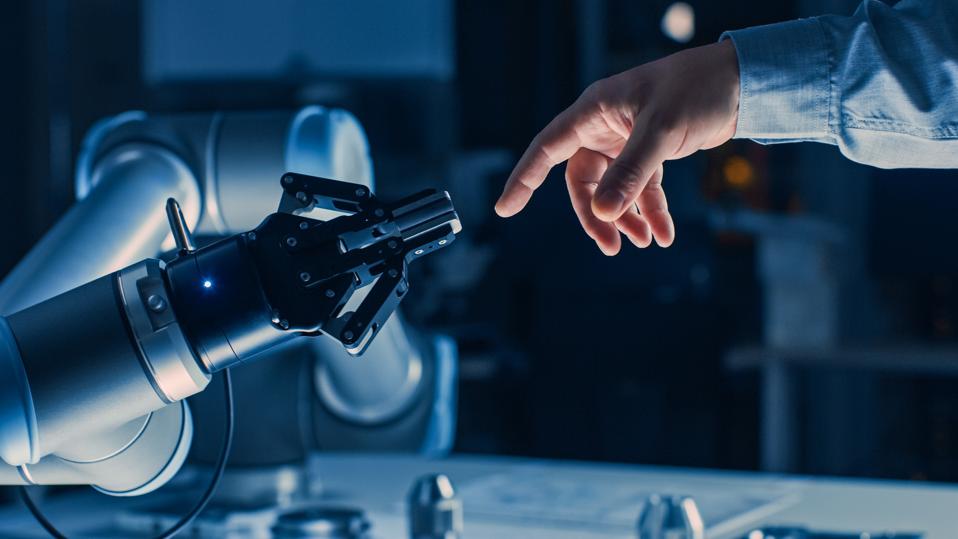
Scaling Business with Hyper-Automation

Automating manual processes with AI-powered software bots is key to scaling efficiently. Let's explore two automation use cases with outsized business impact.
Automating Document Processing
Vital business data is often buried in documents. Manually extracting this data is tedious and expensive. AI document processing automates this workflow.
For example, Rossum offers an AI assistant that replicates human data entry. It analyzes documents and extracts information without templates or rules. Rossum learns to process any document type - from invoices to contracts. It achieves over 90% accuracy, matching skilled professionals.
With Rossum, leading PwC cut document processing time by 80%. Automating such time-consuming tasks drives cost savings and productivity.
Recommending Process Improvements
Most businesses still rely on legacy processes. But few have the expertise to re-engineer these processes for the digital age. AI automation helps fill this gap.
For instance, Celonis offers an AI-powered Process Mining platform. It analyzes your system processes and workflows to build a digital twin. Advanced algorithms compare the optimal process path with real-world execution. Celonis reveals process bottlenecks and recommends data-driven optimizations.
By eliminating process waste, Celonis typically generates over 30% cost savings. Automating process re-engineering with AI unlocks efficiency at scale.

Amplifying Human Potential with AI Augmentation

Beyond automating tasks, AI also elevates human capabilities. Let's look at two emerging use cases of human-AI collaboration.
AI Writing Assistance
Writing well requires creativity and nuance. Can AI really help humans write better? Surprisingly, yes.
Tools like QuillBot and Grammarly use NLP to refine text. But AI can go beyond spellcheck and grammar fixes. For instance, Writesonic offers an AI writing assistant. Just provide a headline, keywords, tone of voice, and output length. Writesonic's algorithms generate an outline, content structure, and written draft. Humans then edit the draft while benefiting from the AI's ideation and framework.
Such tools boost writing productivity over 300%. AI turns unstructured writing into a collaborative process between human creativity and machine intelligence.
Making Agents Smarter at Complex Sales Dialog
Sales conversations require subtle cues like empathy, listening, and nudging. Can AI really handle such nuance? Surprisingly, AI dialogue systems are mastering this art.
For example, Observe.AI provides an AI assistant for sales reps. Its deep learning system listens to hundreds of thousands of sales calls to learn persuasive human conversations. During a live sales call, the AI whispers real-time guidance into the rep's headset on the optimal talking points, questions, and strategies based on cues from the prospect.
This natural coaching helps reps have more effective conversations. In piloting Observe.AI, Cisco increased deal sizes by over 15%. AI dialogue augmentation takes human collaboration to the next level.

Enabling New Possibilities with Generative AI
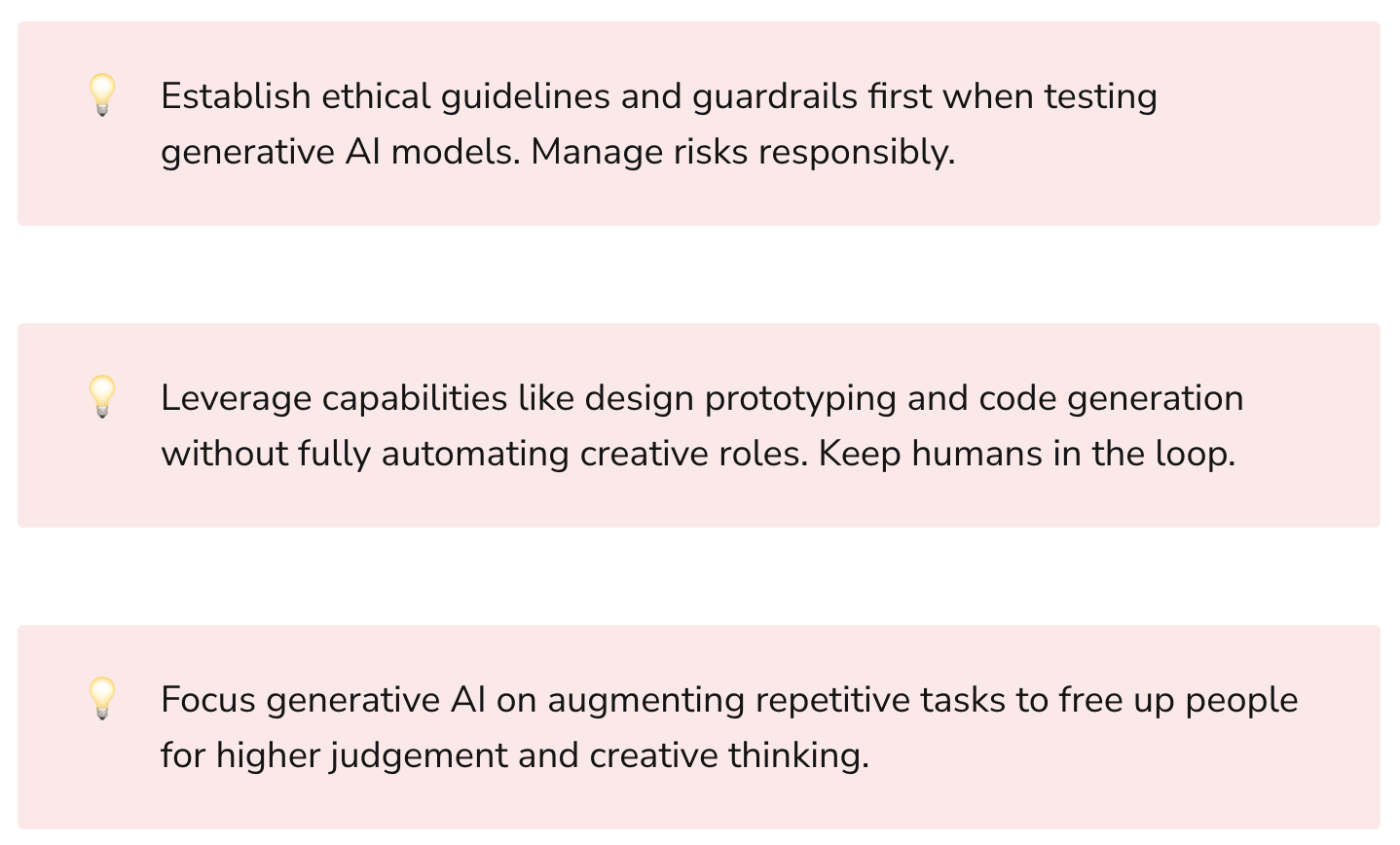
Generative AI models like DALL-E 2, GPT-3, and AlphaCode unlock novel ways to generate content, code, and more. Let's explore two generative use cases.
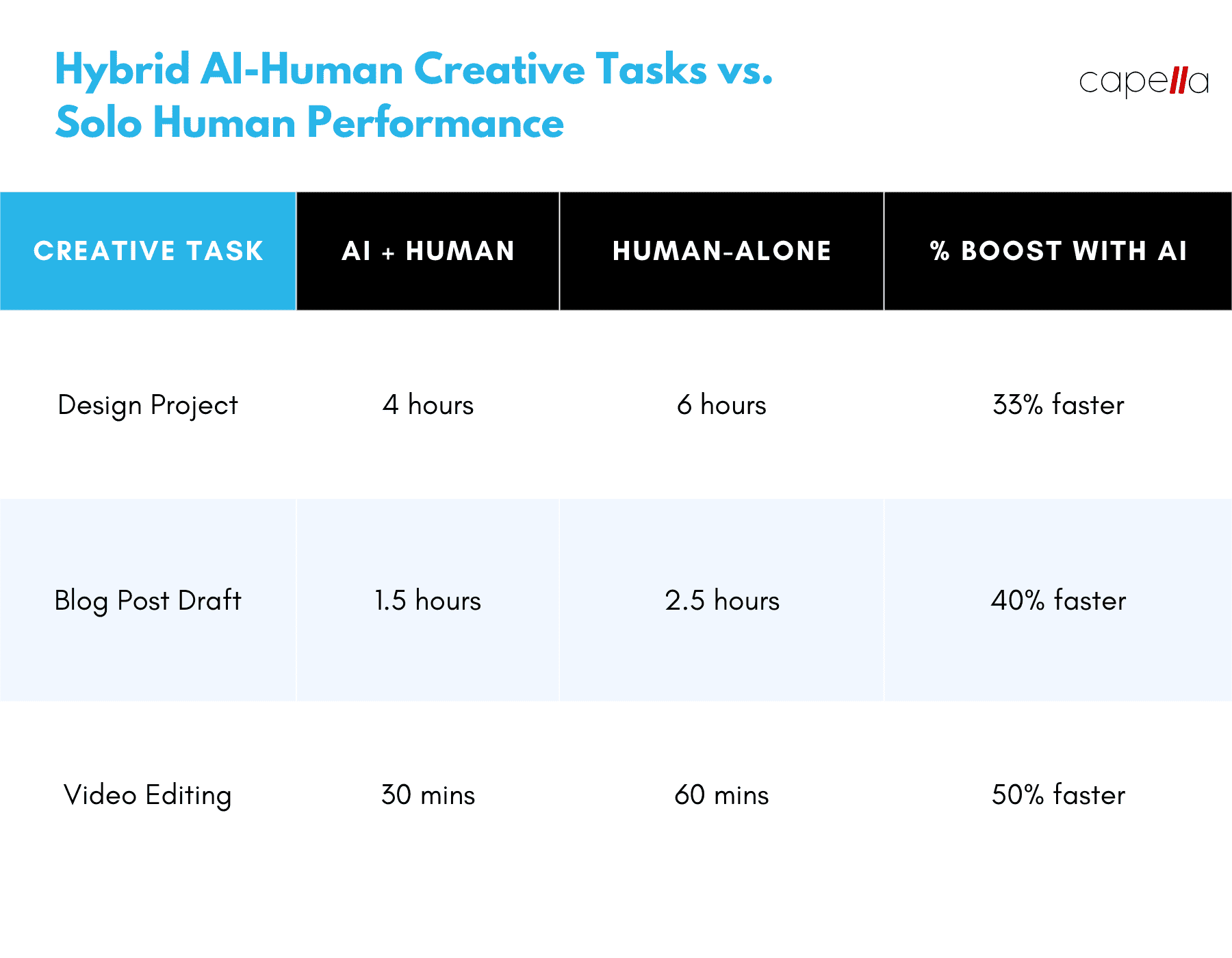
Automating Design Work
Design is an inherently creative human endeavor. Can AI really generate quality designs? Surprisingly yes, and it's transforming design workflows.
For instance, tools like Canva and Tailor Brands offer AI-powered graphic design platforms. Just input your preferences and content. The AI generates customized designs for presentations, social media, ads, and more. Humans can provide creative direction to refine the designs.
Such tools expand design capabilities to non-designers. Even experienced designers can ideate faster by starting with AI-generated designs. The future of design is human-AI collaboration.
Code Completion and Generation
Coding requires deep human insight. Can AI meaningfully help? With advances like GPT-3, the answer is increasingly yes.
For example, GitHub Copilot is an AI pair programmer. As you type code, it suggests entire function definitions, reference implementations, and optimal approaches tailored to the context - all in real time. Copilot can even generate whole boilerplate code snippets from comments. This helps programmers become significantly more productive.
Next-gen code generation platforms like Anthropic's Claude take it further. Claude converts natural language prompts into clean, functional code. For instance, you can simply ask it to "create a python script that scrapes data from this website" and Claude will generate complete runnable code. AI code generation augments human developers in amazing ways.
The Future with AI is Now
AI is no longer a futuristic technology. As the examples above highlight, AI is already broadly transforming business and society today. Forward-thinking leaders recognize that AI's benefits go far beyond hype and cost reduction. Adopting AI now will be essential to compete in the future.
At the same time, businesses need an ethical approach to AI. Powerful generative AI risks abuse if deployed carelessly. Leaders must proactively build responsible and inclusive AI practices.
The possibilities with AI truly seem endless. What creative ideas do you have to leverage AI's potential? The future with AI belongs to those who start today. Let us know your thoughts on these mind-blowing use cases and what's next for AI!
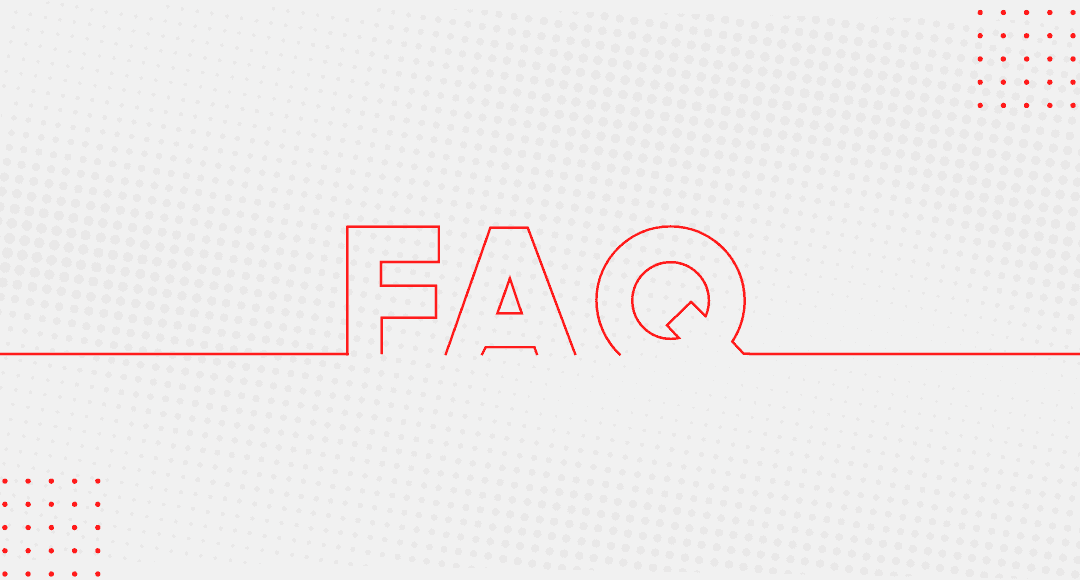
What are some challenges to be aware of when implementing AI in healthcare?
Implementing AI in healthcare comes with substantial challenges that must be navigated thoughtfully. Some key considerations include:
- Patient privacy and data governance is critical when applying AI to sensitive health data. You need robust systems to enforce data privacy, transparency, and ethics reviews.
- AI diagnostics require exhaustive validation to evaluate and address potential algorithm biases. Testing efficacy across diverse patient populations is essential before clinical use.
- Doctors may resist adoption if AI is positioned as replacing human expertise rather than augmenting it. Change management matters.
- Regulation of medical AI will continue evolving. Stay adaptable to comply with new laws, especially regarding transparency and accountability.
- As health data grows exponentially, scalable and robust data pipelines are crucial to train accurate AI algorithms.
How can businesses maximize the value of AI personalization and recommendations?
There are a few best practices businesses can follow to drive value from AI personalization:
- Combine AI insights with ongoing human oversight and A/B testing instead of fully automating decisions. AI is a tool, not the only driver.
- Ensure your training data is unbiased and representative or personalization algorithms can exclude demographics. Monitor and correct bias.
- Build in continuous feedback loops to track when AI suggestions are accepted or rejected. Refine the models accordingly.
- Validate that each personalized recommendation or prediction actually improves desired business outcomes like conversions, LTV, etc.
- Support transparency so customers can see why they received particular suggestions and provide direct input.
What skills does an organization need to adopt AI business automation successfully?
Adopting AI for business automation requires some key skill sets:
- Data engineering and data science skills to build scalable pipelines and machine learning models.
- Change management and employee training abilities as automation transforms workflows.
- Process excellence capabilities to identify optimization opportunities and redesign processes suited for automation.
- Compliance expertise to navigate regulatory issues like data transparency as AI makes decisions.
- Hybrid technical/business acumen to drive returns on automation investments and manage AI-human collaboration.
How can companies ethically implement AI writing and content generation?
Careful oversight is required to ethically apply writing and content generation AI:
- Humans should drive top-level messaging, with AI assisting by generating draft copy. Don't rely wholly on machine-generated content.
- Establish authorship and attribution protocols for AI-generated content with appropriate disclosures.
- Audit content for biases, misinformation, plagiarism, and toxicity. Actively filter harmful content.
- Foster responsible use by limiting auto-generated content to internal teams and discouraging external publication without substantial editing.
- Consider limiting highly advanced models like GPT-3 to reduce risks of propagating harmful, biased, or misleading content.
What are some best practices for leveraging generative AI responsibly?
Here are some best practices for leveraging generative AI responsibly:
- Establish clear human oversight protocols for reviewing and approving any outputs before sharing or publishing.
- Implement stringent content moderation to filter out harmful, biased, or low-integrity outputs. Avoid releasing unvetted content.
- Maintain full transparency on use cases and publish regular impact assessments and ethics reviews.
- Govern access tightly to prevent abuse or over-generation of unnecessary content that wastes resources.
- Develop technical solutions like watermarking and attribution techniques to distinguish AI-generated content.
How can companies get started with AI today in a scalable way?
Some tips to get started with scalable AI adoption:
- Focus initial projects on augmenting employees versus full automation. Tools like Copilot improve productivity without disruptive change.
- Start with off-the-shelf AI through cloud platforms like AWS and Azure instead of building from scratch.
- Concentrate AI capabilities in small internal teams first, testing solutions before broad rollout.
- Choose narrowly-defined automation use cases like invoice processing to demonstrate quick ROI. Then scale.
- Invest in change management and employee education to build support and amplify AI's benefits.
What are the limitations of current AI capabilities companies should recognize?
Current AI has important limitations including:
- Algorithms require massive training data. Data scarcity can impede progress in some business domains.
- Explainability is still limited. Interpreting AI model logic remains difficult.
- AI struggles with contextual reasoning and common sense humans intuitively have.
- There are unsolved challenges in transferring learning between tasks and across different data distributions.
- Predictive modeling failures can be hard to anticipate. Gradual deployment with fallback plans is prudent.
- Securing model integrity and preventing data poisoning attacks is an emerging concern.
How can companies build responsible AI practices internally?
Some ways companies can promote responsible AI:
- Create ethics review boards with diverse experts to oversee projects and address emerging risks proactively.
- Institute bias bounty programs to incentivize discovering model issues so they can be resolved quickly.
- Make AI principles like transparency, fairness, and accountability core pillars of your model development process.
- Build tools to detect and mitigate unintended harm from model predictions early. Actively listen to concerns.
- Support external research and open standards that advance the safety and benefits of AI for all of society.
What is the best way to start implementing AI in my company?
The optimal way to start adopting AI depends on your business context, but general tips include:
- Focus on augmenting employees before pursuing wide automation to build trust and capabilities.
- Identify narrowly-defined automation opportunities with low risk and quick ROI, like form processing.
- Leverage off-the-shelf AI cloud services instead of building complex models from scratch initially.
- Start with a pilot project with a small user segment to evaluate real-world performance before scaling.
- Involve cross-functional teams in planning, especially change management and employee education.
- Assign dedicated AI leads and devote adequate budget. Progress will take strategic commitment over years.
How do I convince skeptics in my industry that AI can make a real impact?
Some ways to convince AI skeptics include:
- Find the early adopters who see the potential and work with them to demonstrate value. Then highlight their results.
- Start with less disruptive AI assistance tools like AI writing tools that remove roadblocks vs replace jobs.
- Relate AI benefits to their key pain points around efficiency, personalization, decision making, etc. Make the use case tangible.
- Be balanced on AI's limits and emphasize it as an augmentation tool. Over-promising undermines credibility.
- Encourage small pilots to build familiarity with evidence-based results. AI pilots convert many skeptics.
- Note competitors adopting AI successfully. Peer usage often persuades reluctant adopters more than hypotheticals.
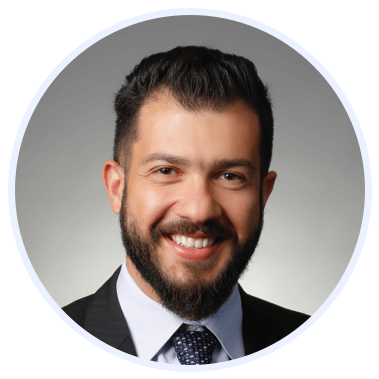
Rasheed Rabata
Is a solution and ROI-driven CTO, consultant, and system integrator with experience in deploying data integrations, Data Hubs, Master Data Management, Data Quality, and Data Warehousing solutions. He has a passion for solving complex data problems. His career experience showcases his drive to deliver software and timely solutions for business needs.