.png)
An analytics platform is a powerful tool that combines data storage, data preparation, data analysis, and other data analytics processes, all in one convenient package. It's like the Swiss Army knife for data exploration - with it, you can slice and dice your data however you like, unlocking insights that can help you make better decisions and improve operations.
And while there are many analytics platforms out there, Capella is making waves in the analytics industry. With Capella, businesses can quickly and easily access, analyze, and visualize data - all in one place. So, if you're looking for a one-stop solution for all your analytics needs, Capella is the perfect choice. Get started with Capella today and see what insights you can uncover!
But what exactly is an analytics platform? Well, this article explains it all! We will cover the following topics to help you better understand analytics platforms:
- How to build a data platform
- What are the different types of data analytics?
- What to consider when looking for an enterprise analytics platform
- Leveraging analytics platforms to make data-driven decisions
By the end of this article, you will have a better understanding of analytics platforms and how they can help your business.
How To Build A Data Platform
Building a data platform is an important step for any business that wants to make data-driven decisions. Data platforms are the foundation of any analytics strategy, and it is essential to have a reliable and secure platform that can scale to meet the needs of the business.
There are several components to consider when building a data platform. The first step is to determine the data that needs to be collected and stored. This includes internal and external sources, such as customer data, sales data, financial data, and more.
Once the data is identified, the next step is to decide on the technology that will be used to store the data. This could include traditional databases, cloud-based storage solutions, or a combination of both.
Once the data is stored, it needs to be made available for analysis. This requires the data to be prepared and organized in a way that makes it easy to access and analyze. This can be done manually or through automated processes, such as ETL (extract, transform, load) processes.
The final step is to decide on the analytics tools and technologies that will be used to analyze the data. This could include traditional analytics tools, such as spreadsheets, or more advanced tools, such as machine learning and artificial intelligence. It is important to choose the right tools to analyze the data accurately and efficiently.
By following these steps, businesses can build a reliable and secure data platform that can be used to make data-driven decisions. With the right platform in place, businesses can unlock the power of their data and make informed decisions that will drive their success.

What Are The Different Types Of Data Analytics?
Data analytics involves collecting, organizing, analyzing, and interpreting data to gain insights and knowledge. It is used to uncover data sets' patterns, trends, and correlations to make informed decisions.
There are several types of data analytics, each with its distinct purpose and approach. Let’s look at some.
- Descriptive Analytics
As the name implies, the goal of descriptive analytics is to simply describe what has occurred; it does not attempt to speculate on possible causes or establish cause-and-effect connections. The only goal is to give a quick, digestible overview.
A good example of descriptive analytics in action is Google Analytics, which gives you a quick overview of what's been happening with your website. For instance, it shows how many people visited your website over a specific period or where they came from. Similarly, tools like HubSpot demonstrate how many recipients of a specific email or campaign engaged with it.
Data aggregation and mining are the two main methods employed in descriptive analytics. The act of gathering data and presenting it in a condensed form is known as data aggregation. Consider an online retailer that gathers a variety of information about its clients and website visitors.
The aggregate data, also known as summarized data, gives a general overview of the larger dataset, such as the typical customer age or the typical number of purchases. Data mining is the analysis part. Analysts investigate data to look for any patterns or trends.
A descriptive analysis produces a visual representation of the data, such as a bar graph or pie chart.
- Diagnostic Analytics
The goal of diagnostic analytics is to delve further into the reasons behind events. The main goal of diagnostic analytics is to locate anomalies in your data and take appropriate action. For instance, you'll want to learn why if your descriptive analysis reveals a 20% decline in sales for the month of March.
A diagnostic analysis should be carried out as the next logical step. The analyst will begin by locating any additional data sources that could provide insight into why the decline in sales occurred to get to the root cause.
If they dig deeper, they might discover that, despite a sizable number of website visitors and "add to cart" actions, very few customers actually checked out and made purchases. Further investigation can reveal that the majority of customers dropped the ball when asked to enter their delivery address.
We're making progress at last! The address form appears to have been problematic; perhaps it wasn't loading properly on mobile devices or was simply too lengthy and annoying. You can get closer to discovering an explanation for your data anomaly by doing a little more research.
However, diagnostic analytics can also be employed to determine what is causing successful outcomes. Maybe the statistics show that website traffic skyrocketed in October - a whopping 60% increase from the previous month!
- Predictive Analytics
The goal of predictive analytics is to foresee likely future events. Data analysts can create predictive models that calculate the likelihood of a future event or outcome based on patterns and trends in the past. Because it enables businesses to plan ahead, this is especially helpful.
For example, you could use the correlation between seasonality and sales figures to forecast when sales are likely to decline. Predictive models use the relationship between a set of variables to make predictions.
Suppose your predictive model indicates that summertime sales are likely to decline. In that case, you might use this information to develop a promotional campaign focused on the summer or cut spending elsewhere to compensate for the seasonal decline.
- Prescriptive Analytics
Prescriptive analytics is an advanced form of analytics that goes beyond the descriptive and predictive analytics that are more commonly used. It uses data and advanced algorithms to not only provide insights into what happened in the past and what is likely to happen in the future but also to provide insights into what should be done in the present.
It works by combining data and advanced algorithms to provide insights into what should be done in the present. It looks at the data from the past and present, then applies predictive algorithms to identify patterns and trends. It then uses these patterns and trends to provide insights into what actions should be taken in the present to achieve the desired outcome.
For example, if a company wants to increase sales, prescriptive analytics can be used to identify the best combination of marketing and sales tactics that will lead to the desired result.
Also, companies leverage prescriptive analytics to identify the best course of action in complex situations. For example, a company may use prescriptive analytics to identify the best way to respond to a customer complaint or to identify the best way to optimize a supply chain.
- Cognitive Analytics
Cognitive analytics is an intelligent technology that covers multiple analytical techniques to analyze large data sets and give structure to unstructured data. Simply put, a cognitive analytics system searches through the data stored in its knowledge base to identify answers that make sense for the problems being addressed.
Cognitive analytics can be thought of as analytics with human-like intelligence. This can involve comprehending a sentence's context and meaning or, given a lot of information, identifying specific objects in an image. A cognitive application can get better over time because cognitive analytics frequently uses machine learning and artificial intelligence algorithms. Simple analytics cannot reveal certain connections and patterns that cognitive analytics can.
A company may use cognitive analytics to keep track of customer behavior trends and new developments. This way, the organization can forecast future results and adjust its goals to perform better.
Some sections of cognitive analytics also fall under predictive analytics, where data in business intelligence is used to make predictions.
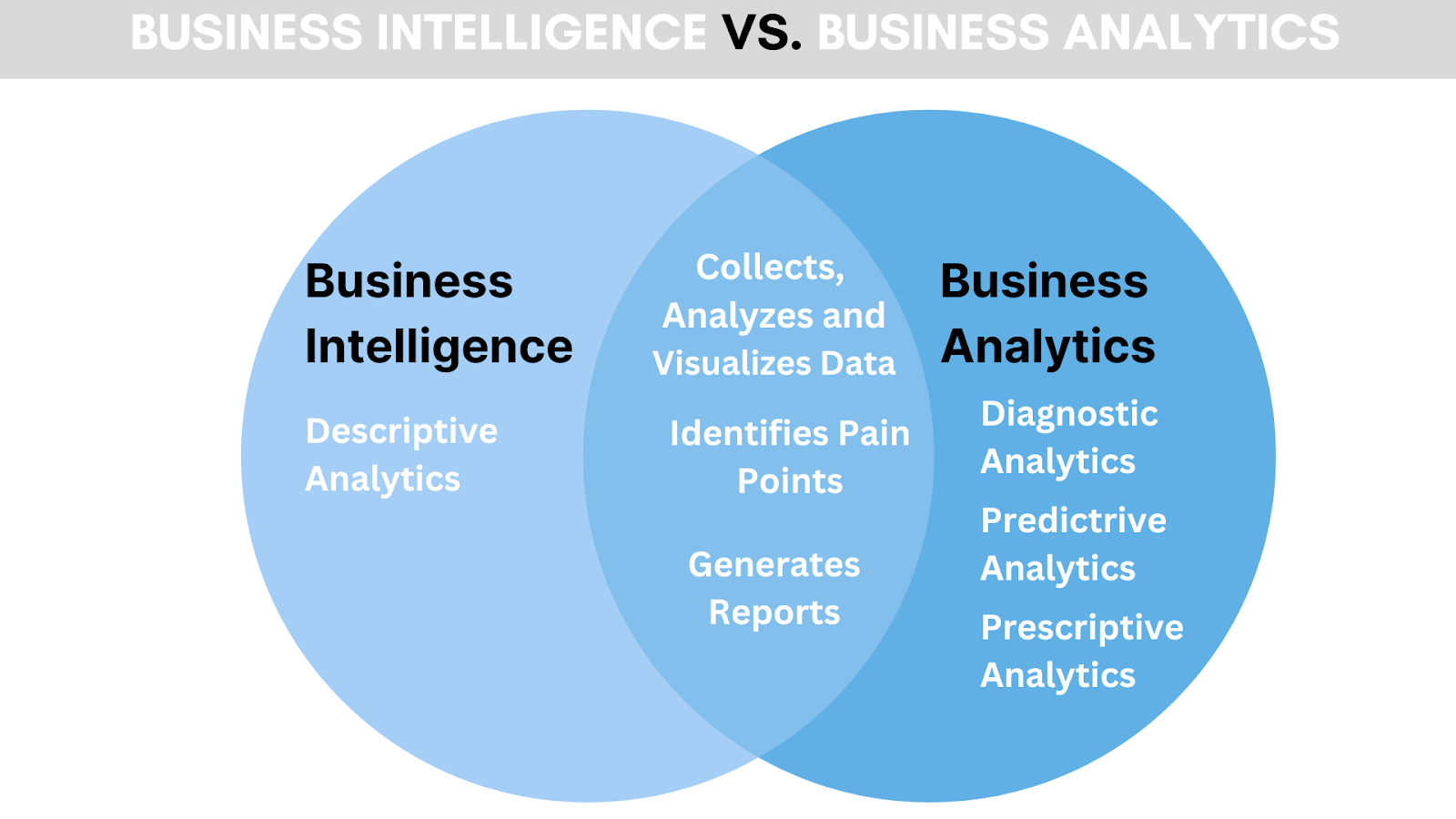
What To Consider When Looking For An Enterprise Analytics Platform
When looking for an enterprise analytics platform, there are a few key considerations to keep in mind.
Consider The Scope Of The Platform
Does it provide the necessary data storage and management capabilities, as well as the necessary data preparation and other data analytics processes?
Consider The Scalability Of The Platform
Does it offer the ability to scale up or down as your data needs change?
Consider The Security Of The Platform
Does it provide the necessary security measures to protect your data?
Consider The Cost Of The Platform
Is it within your budget?
By considering these factors, you can ensure that you select the right enterprise analytics platform for your needs.
An enterprise analytics platform should provide the necessary data storage and management capabilities, as well as the necessary data preparation and other data analytics processes. Additionally, it should be scalable, secure, and cost-effective.
With an enterprise analytics platform, you can leverage the power of data to make informed decisions and drive your business forward.
Why Companies Turn To Enterprise Analytics Platforms
In today’s digital world, companies are looking for ways to leverage data to gain insights that can help them make better decisions. This is why many companies are turning to enterprise analytics platforms.
An analytics platform provides an integrated suite of tools and services that enables organizations to quickly and easily access, analyze, and visualize data. By harnessing the power of analytics, companies can gain a deeper understanding of their customers, operations, and markets.
Additionally, analytics platforms provide companies with a wide range of capabilities to help them make better decisions. For example, analytics platforms can help organizations identify patterns in customer behavior, identify trends in market conditions, and uncover insights into their operations. Plus, they can be leveraged to develop predictive models that can help organizations anticipate future outcomes and make better decisions.
Analytics platforms also provide organizations with the ability to quickly and easily access and analyze data from a variety of sources. This makes it easier for organizations to quickly and easily access data from multiple sources and gain insights into their operations. With these platforms, companies can quickly and easily create custom data models - which can be used to gain insights into their operations.
Leveraging Analytics Platforms To Make Data-Driven Decisions
Data-driven decisions are becoming increasingly important for businesses of all sizes. With the help of an analytics platform, companies can gain insights into their data, allowing them to make decisions that are informed by evidence.
One modern analytics platform that offers a comprehensive solution for all businesses is Capella. From its AI technology and consumer-grade search capabilities to an intuitive and user-friendly platform, Capella delivers true self-service analytics that everyone in any organization can use. With Capella, organizations can quickly and easily access and analyze their data to gain the insights they need to make better decisions.
So, if you’re looking to leverage analytics to drive your business forward, Capella is the ultimate analytics platform. Get started with Capella today and unlock the power of data!
Final Thoughts
Analytics platforms provide businesses with the tools and insights they need to make data-driven decisions. By unifying data storage, management, and analytics processes, these powerful platforms can help businesses gain insights into their operations, customers, and markets. And with a revolutionary platform like Capella, businesses of all sizes can quickly and easily unlock the power of data to drive their business forward.
So, contact Capella today and let us help you make the most of your data!
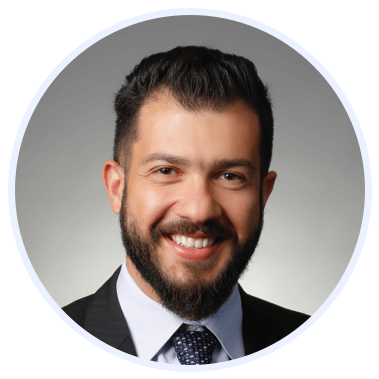
Rasheed Rabata
Is a solution and ROI-driven CTO, consultant, and system integrator with experience in deploying data integrations, Data Hubs, Master Data Management, Data Quality, and Data Warehousing solutions. He has a passion for solving complex data problems. His career experience showcases his drive to deliver software and timely solutions for business needs.