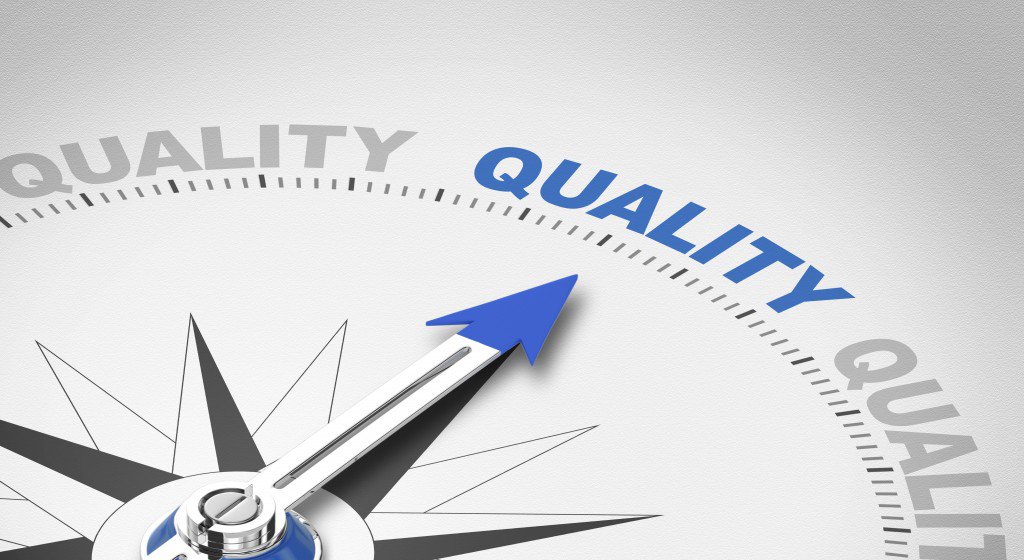
Data quality analysis has become increasingly important as businesses have become more data-driven. The ability to collect and analyze large amounts of data has allowed companies to gain previously unavailable insights. As a result, businesses need to understand data quality to make better decisions.
Statistics show that 83% of organizations view data as crucial to developing a business strategy. Because of this, having quality data is considered the most vital aspect of a successful firm.
This article will look closely at data quality to understand its importance and how you can improve it.
What is Data Quality?
Data quality refers to how accurate data is and its completeness. In other words, it measures how well data meets the requirements of its intended use. It's essential to improve data integrity in any data-driven decision-making process. It's also important to maintain high-quality data to ensure that it's usable and reliable.
The data source, data collection methods, data processing procedures, and data storage can all contribute to the level of your data quality.
Data quality is subjective, so what one considers high-quality data for one purpose may not be regarded as high-quality data for another purpose. This means that different organizations can use different approaches to data quality.
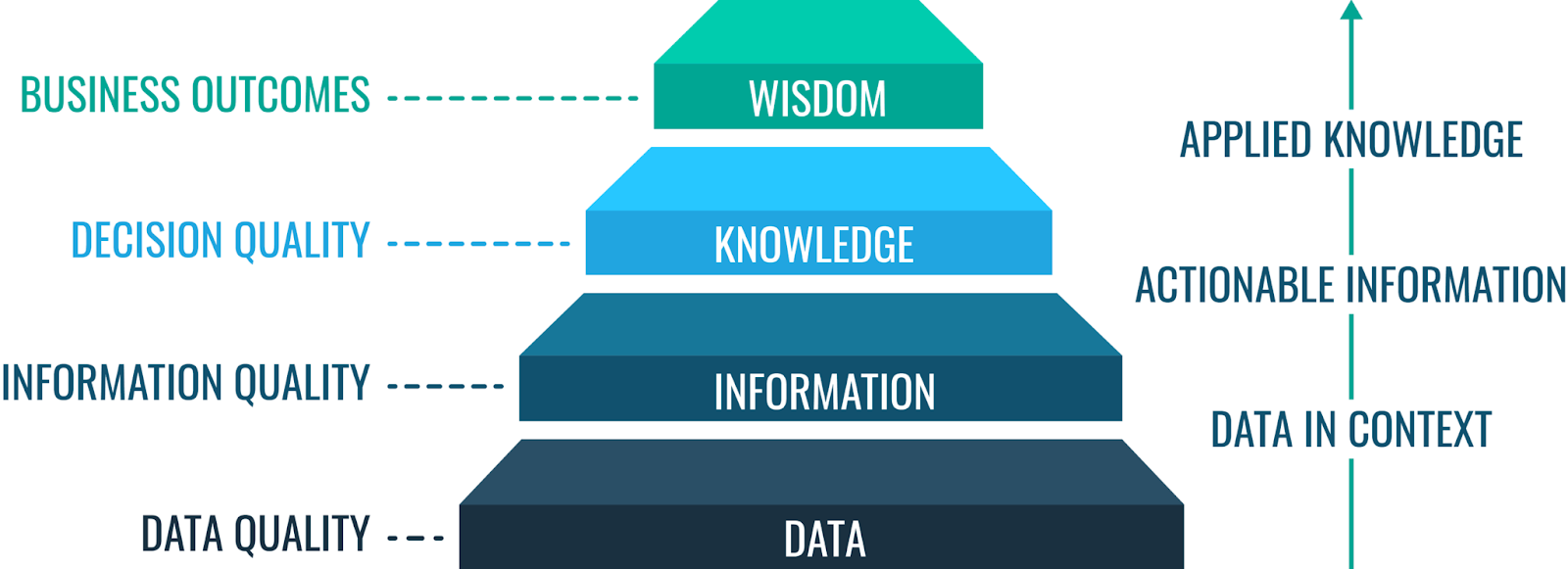
How to Determine Data Quality?
How do you know the quality of data you have? There are six common data quality dimensions, and it's critical to consider them when evaluating data.
Consistency
Data consistency shows the degree to which different data you have in your system matches and has no variations. Ask yourself, does the data contain all the necessary pieces? Is it uniform with other related information?
Timeliness
Timely data is crucial for the functionality of any business. For it to be timely, ensure that it's up-to-date; it should reflect any recent changes or improvements before being distributed.
Validity
Data should accurately reflect what it purports according to business standards or guidelines.
Uniqueness
Quality data should be free from duplicates or overlaps. Ensure that all values from the data set can be individually identified and accessed. Copies affect data accuracy and usefulness, hence reducing the high uniqueness score.
Completeness
Data completeness measures whether the values you have are comprehensive. Have you included every piece of relevant information in the database? Incomplete data can lead to wrong decisions, creating costly mistakes.
Accuracy
Research shows that 32% of companies believe their data is inaccurate. To remove this doubt, ensure everything is correct, precise, and complete to reflect reliable information. This includes eliminating errors that may occur during transcription or recording processes.
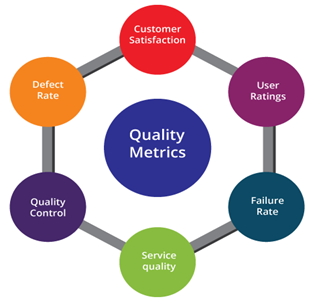
Why Is Data Quality Important?
Data is the lifeblood of a company, so poor data quality hurts your business. Bad data quality costs businesses $12.9 million yearly on average. Here are some benefits of data quality:
Benefits of Data Quality
There are many benefits of having high-quality data, including:
Improved Decision Making
High-quality data enables businesses to make better decisions, both strategically and tactically. When decision-makers trust the data, they are more confident in taking risks for the company to get to the next level of success.
According to Gartner, Senior Director Analyst, the effectiveness of decision-making directly relates to the quality of the data used.
Enhanced Customer Experience
Good data quality helps businesses understand their customers and provides them with suitable products and services.
Furthermore, quality data helps provide your consumers with timely assistance and also helps build personalization. For example, if your customer's data is inaccurate, you may find you delivered items to the wrong address, which can lead to losing that customer's trust. A survey shows that 13% of retailers would change a brand due to late deliveries.
Besides enhancing customers' experience, you'll retain them and attract more.

Increased Productivity
Good data quality can help businesses eliminate errors, increase efficiency and productivity. Also, it allows your teams to give attention to other important tasks rather than working to correct previous mistakes. In addition, with good data quality, there will be new strategies and more incredible turnaround times, since no time will be wasted.
Improved Marketing
Companies with good data quality can identify and improve their marketing strategies' effectiveness. For example, keeping high-quality data helps you understand which marketing strategies are successful and which need a different approach.
It can also help you improve audience targeting. This way, you can reach people who are more likely to engage with your content or who are likely to buy your products.
Poor quality data can lead to wasting money, time, and resources, since you'll focus on a broad audience that may not notice your services or products.
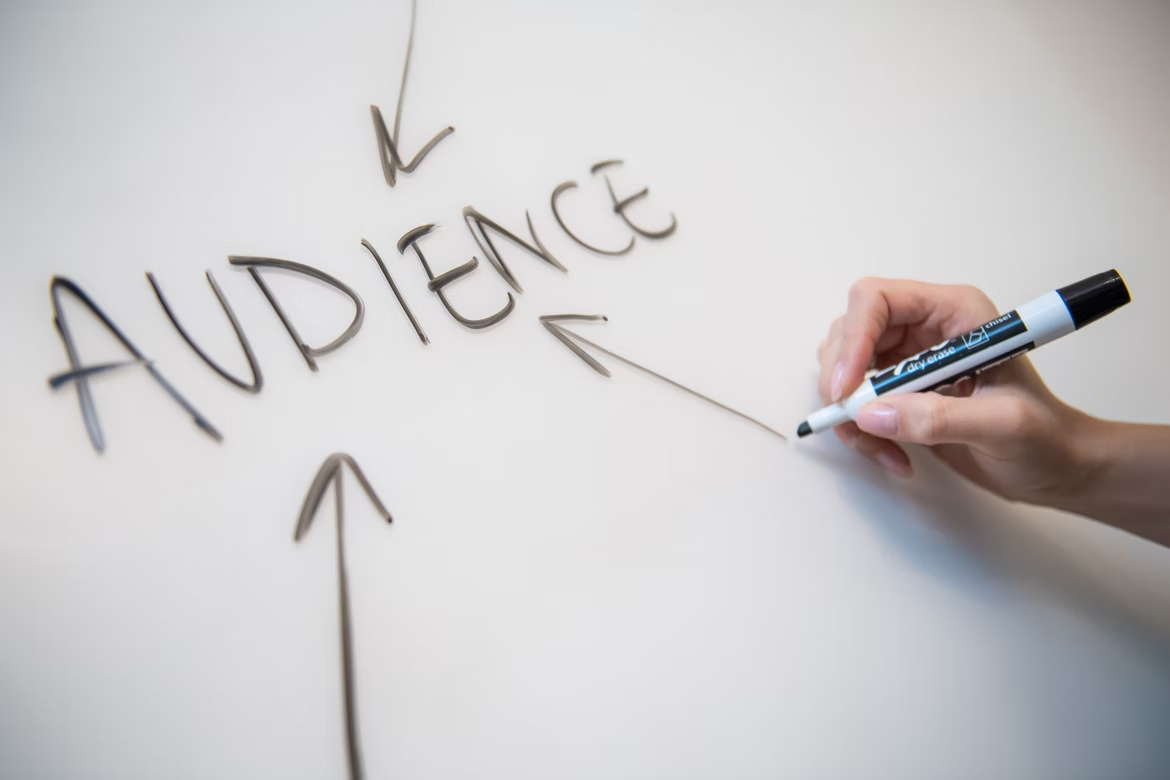
Increase in Business Opportunities
Good quality data provides a company with the leverage to compete with its competitors. Also, with reliable data, you can easily spot new trends and seize the opportunity before your competitors do.
Increased Revenue
According to studies, 91% of businesses believe that bad data quality wastes revenue. This may happen through costs associated with operational inefficiencies, missed sales opportunities, and losing customers, among other factors.

However, complete and consistent data can eliminate waste by taking advantage of business opportunities, perfecting customer experiences, and focusing on the effectiveness of marketing strategies.
Factors Affecting Data Quality
Many factors can affect the quality of data, such as:
Source of the data: The quality of data can be affected by its source. For example, data collected manually is more likely to be inaccurate than data collected automatically.
Method of data collection: The technique used to collect data can affect the data quality. For example, data collected through surveys are more likely to be inaccurate than data collected through observational studies.
Type of data: The quality of data can vary depending on the type of data. For example, qualitative data is more likely to be inaccurate than quantitative data.
How Do You Improve Data Quality?
Everyone within the organization who has a direct or indirect connection to data is involved in the data quality improvement process. After all, if it's of poor quality, any insights gleaned from it will be of limited value, affecting the whole organization. So how do you improve the data quality?
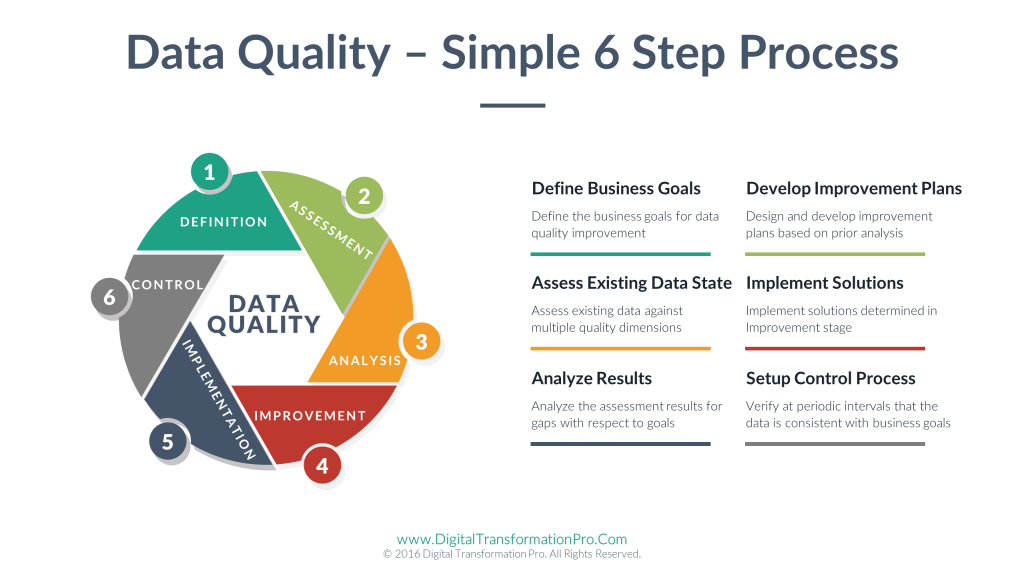
Define What Data Quality Means for Your Business
The first step for improving data quality is understanding what level of quality your organization needs. Data quality is not a one-size-fits-all situation, meaning what works for another company may not be relevant to yours. That's why it's essential to define acceptable data quality for different data types depending on their use.
Implementing Processes and Technologies Data Collection
There is no single silver bullet for collecting high-quality data. Instead, it combines techniques and technologies to ensure data is accurate and complete.
Identify and implement the processes used during the data collection process. This may include a data governance framework, business glossary, and a data quality issues log. Also, have the right technology that captures data consistently and accurately.
Create Standards for Data Entry and Storage
It's crucial to impose some standards to govern data entry and storage. This will ensure that only data that meets the guideline is accepted in a database.
In addition, you can use a defined list of values where possible. Also, you can use normalizing tools to help organize your records.
Regular Audits and Cleansing Data
Regular auditing and cleaning of data will help detect and correct incorrect and inconsistent data in the data set. When this is done early, you can prevent wrong decisions.
Create a Data-Driven Culture
Creating a data-driven culture means ensuring that everyone in the organization understands the importance of data.
Also, employ data governance to standardize data management and ensure the data is consistent and trustworthy. This can be possible if you nominate a data steward to ensure accountability and complete data quality supervision.
When data is given the proper attention, it can be used to improve operations, make better decisions, and drive growth.
Get a Data Quality Management Solution
Integrating an automated monitoring solution for data management is a much faster and more effective way to improve data quality. This solution can help you track, monitor, and assess the quality of your data.
Automated monitoring will auto-detect relevant insights such as anomalies, trends, and patterns and provide real-time results.
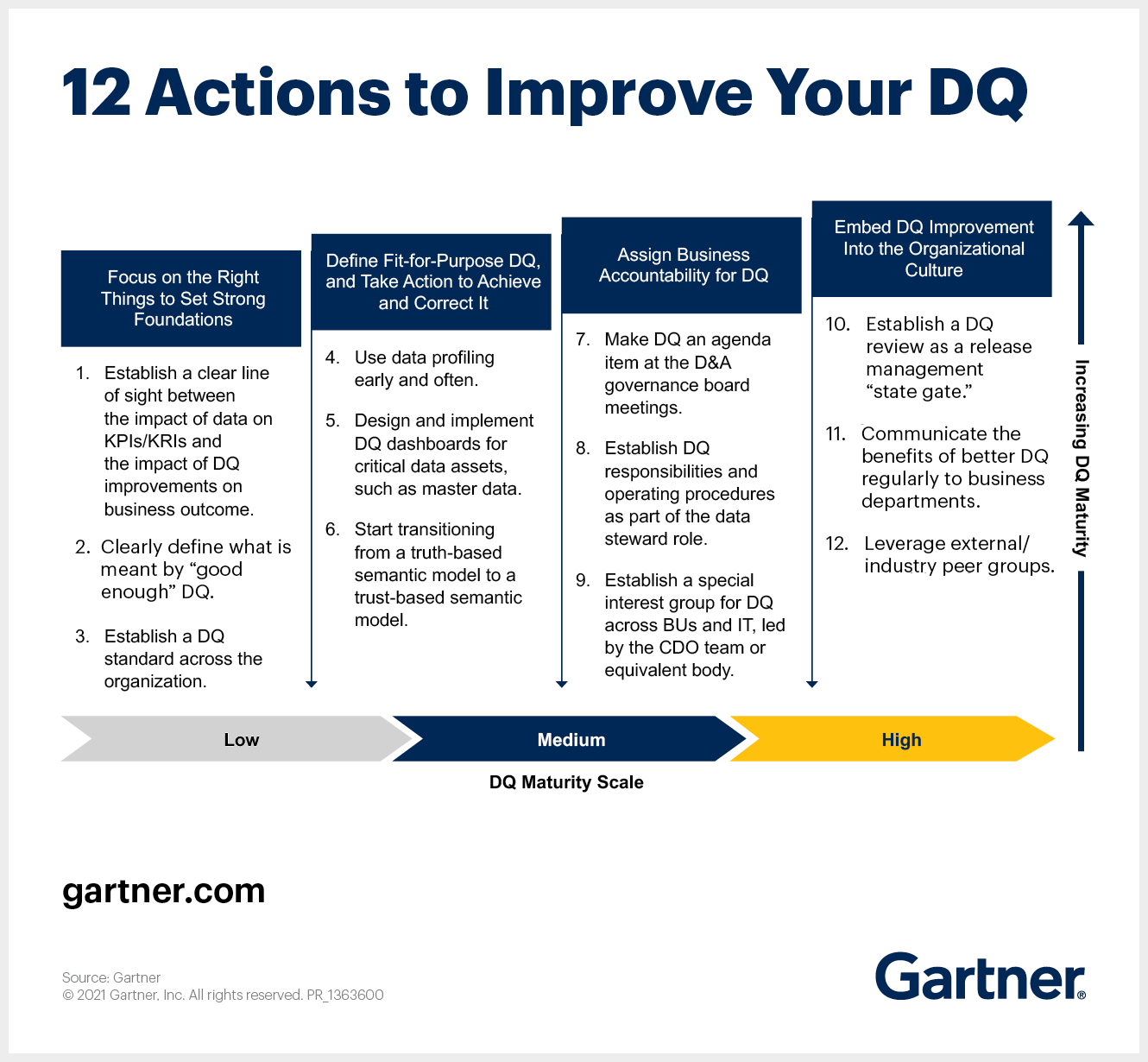
Data Quality Management
Data quality management (DQM) is the systematic process of improving data quality in an organization. For example, identifying and correcting errors and ensuring that data is up-to-date and consistent across all sources. A data manager undertakes DQM from the acquiring process to its distribution.
Data quality management helps reduce data quality issues like duplicates, unstructured, and incomplete data.
Data Quality Management Tools
Data quality management uses specific tools to ensure the subjective data quality metrics are top-notch, making the data trustworthy to be used in making informed decisions. Here are the standard data quality tools you can use:
- Data cleansing: Data Cleansing, also data cleaning, is the removing or changing of data that is not required for analysis. This may include duplicate, missing, invalid, or inaccurate records.
- Data profiling: involves analyzing, reviewing, and summarizing the contents of a dataset to determine its characteristics and identify any outliers.
- Data standardization: This involves creating a consistent set of metadata about each dataset so it can be analyzed adequately and used in reports.
- Data Enrichment: Adding new data items to an existing database or system. This helps ensure your databases are as complete as possible.
- Data matching: Comparing two sets of data and matching them to check for duplicates, incompleteness, or errors.
- Data parsing: Transforming data in a dataset from one format to another to achieve a much-needed and organized structure.
-
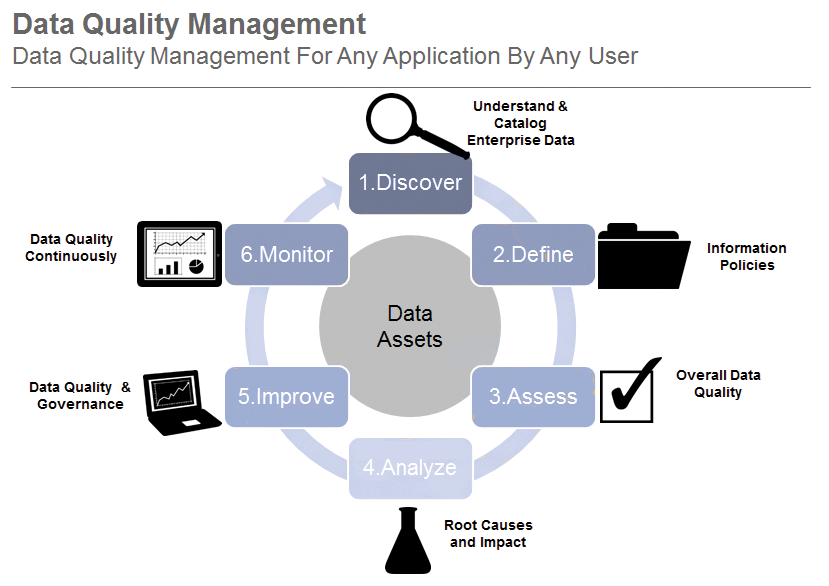
Conclusion
The quality of the data you use can determine the success of your business. That's why it's essential to ensure it meets all the data quality dimensions above to ensure the smooth running of activities. High-quality data can lead to increased business opportunities, enhanced customer experience, productivity, and improved decision-making. To achieve this, you'll need to educate your team, set standards, and have a data quality management system.
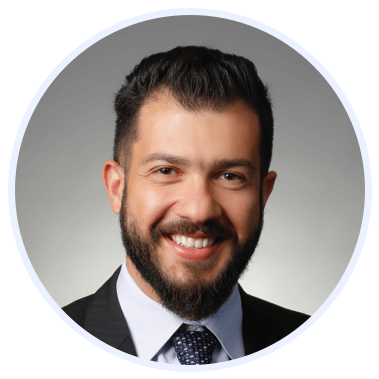
Rasheed Rabata
Is a solution and ROI-driven CTO, consultant, and system integrator with experience in deploying data integrations, Data Hubs, Master Data Management, Data Quality, and Data Warehousing solutions. He has a passion for solving complex data problems. His career experience showcases his drive to deliver software and timely solutions for business needs.