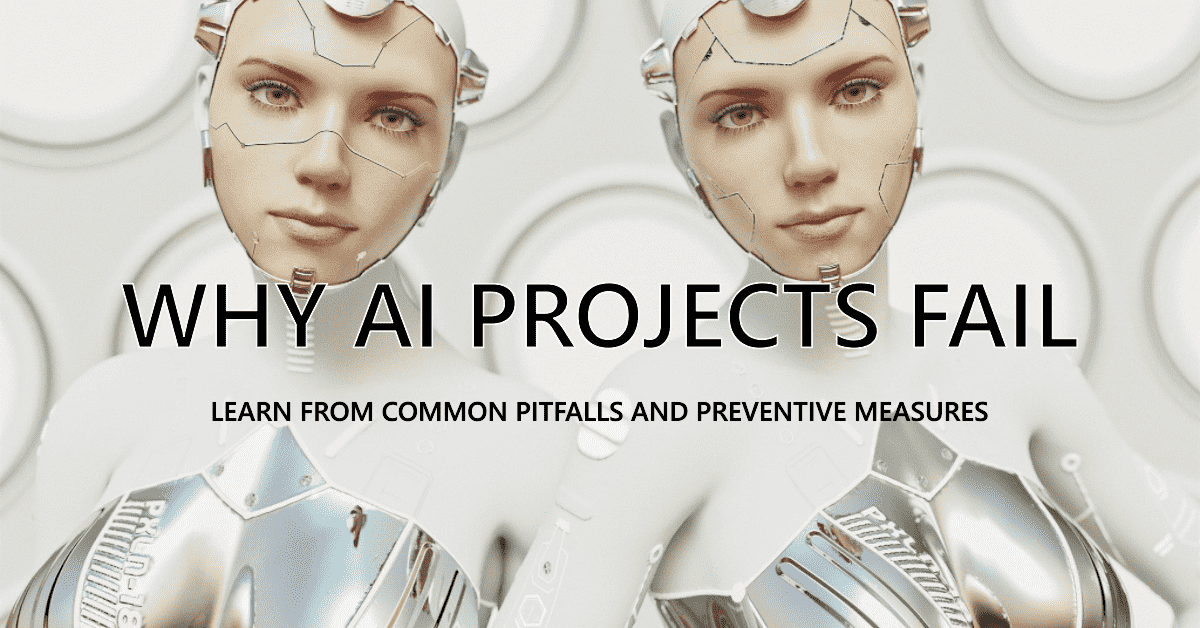
There's a particular kind of magic in the air these days. It's the kind of magic that promises to transform businesses, revolutionize industries, and reshape the world as we know it. Yes, you guessed it right - it's the magic of Artificial Intelligence (AI).
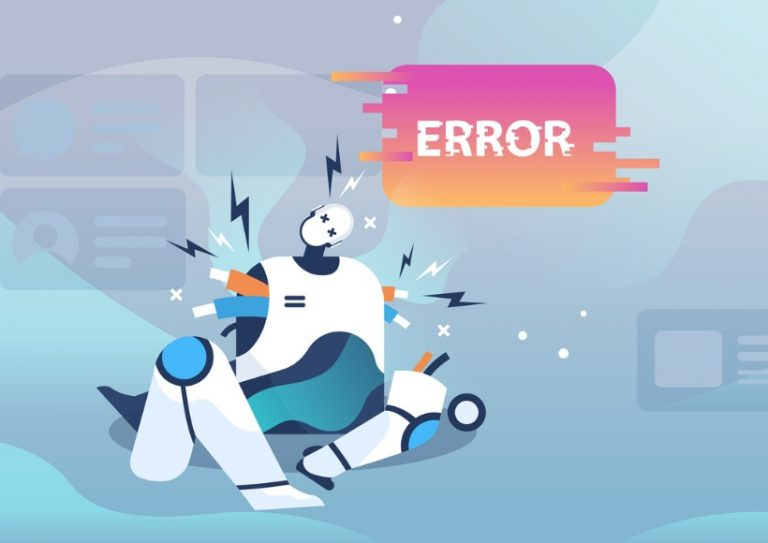
However, not all that glitter is gold. A Gartner survey reveals that 85% of AI projects ultimately fail to bring their organizations the desired returns. So, why do so many AI projects fall short of their promise?
Let's dive deep into this issue, explore the most common pitfalls, and discuss the preventive measures that you, as a decision-maker, can take to avoid these traps.
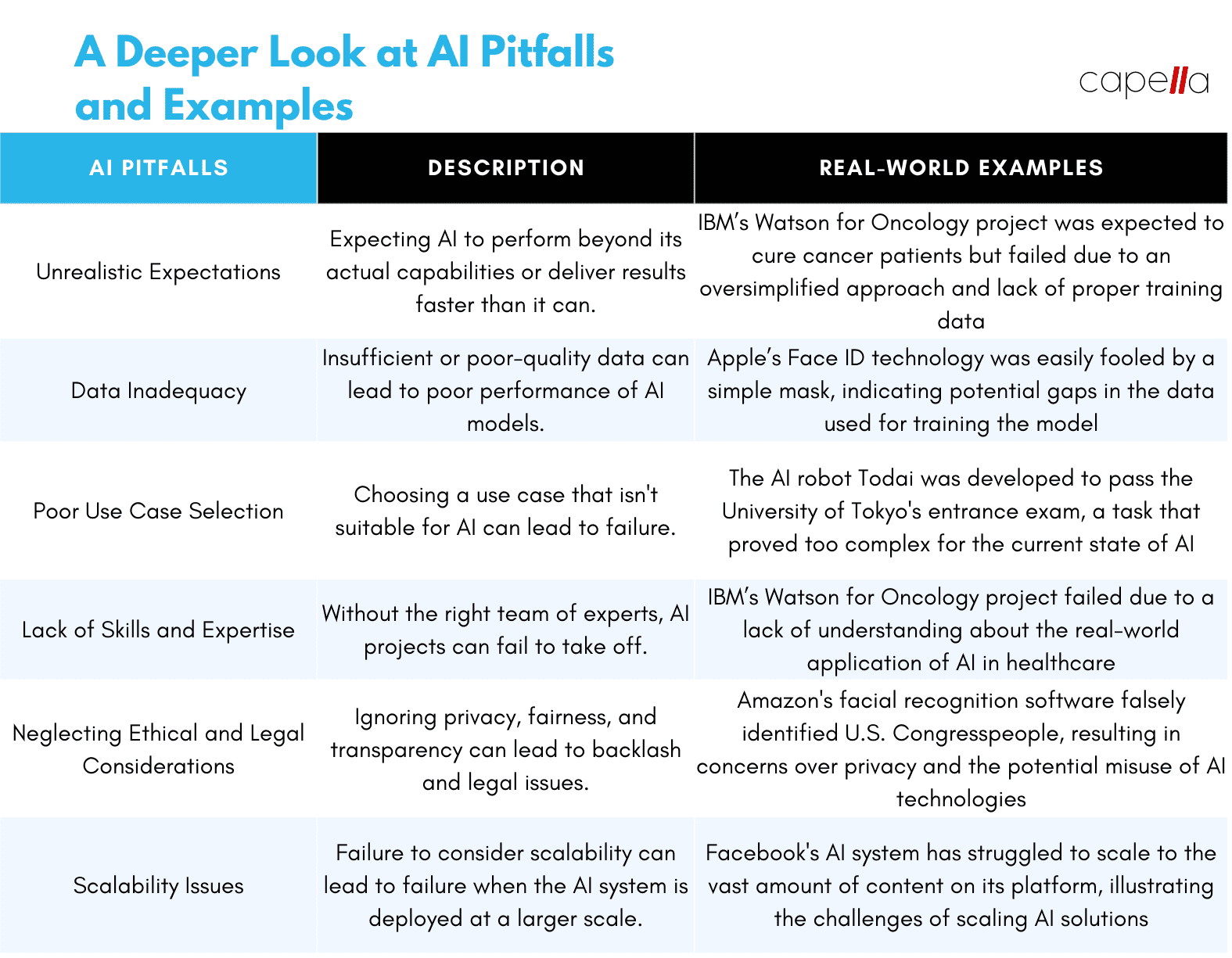
Section 1: The Trap of Unrealistic Expectations
Pitfall: Overhyping AI Capabilities
In the race to stay competitive and relevant, organizations often fall into the trap of overhyping AI's capabilities. They expect AI to solve all their problems and deliver exponential returns right from the get-go.
Example: Consider a large corporation that decides to implement an AI-based customer service chatbot. The decision-makers may expect the chatbot to completely replace human customer service representatives, significantly cut costs, and even improve customer satisfaction scores. In reality, though, AI chatbots are still largely limited to handling simple queries and tasks, and human intervention is required for complex issues.
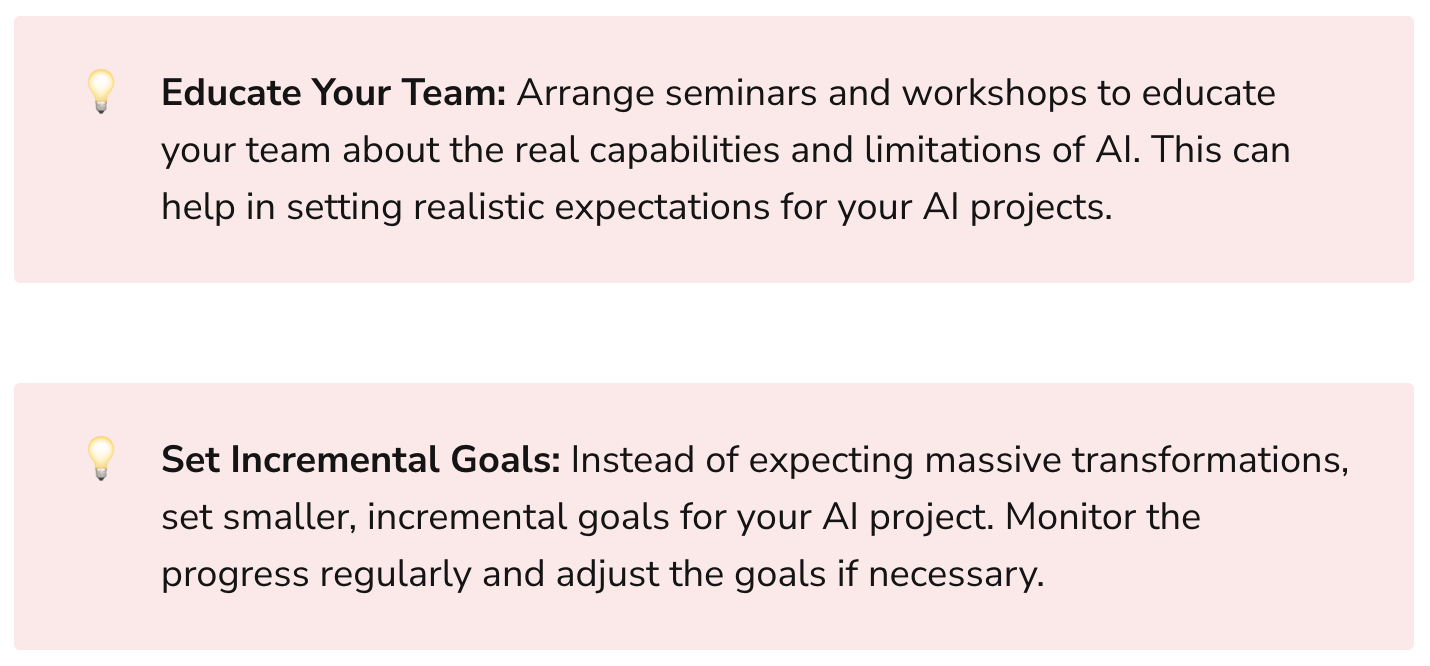
Preventive Measure: Setting Realistic Expectations
To avoid this pitfall, it is crucial to set realistic expectations from your AI projects. Understand the limitations of AI and ensure that your goals align with its current capabilities. Do not expect overnight success; instead, view AI as a tool that can incrementally improve and streamline business processes over time.
Section 2: The Hurdle of Data Inadequacy
Pitfall: Lack of Quality Data
AI projects often fail due to a lack of quality data. AI models learn from data, and if the data is flawed, incomplete, or biased, the model's performance will be affected.
Example: Suppose a retail company wants to use AI to predict future sales. However, if they only have sales data for the past year, the AI model may not be able to accurately forecast sales during periods like Black Friday or Christmas simply because it hasn't seen data for these periods before.

Preventive Measure: Data Management and Augmentation
Invest time and resources into data management. Collect as much diverse and representative data as possible. In cases where data is limited, consider data augmentation techniques to enrich your dataset.
Section 3: The Issue of Poor Use Case Selection
Pitfall: Choosing the Wrong Use Case
Choosing the wrong use case for AI implementation can lead to project failure. Not every problem requires an AI solution, and using AI for the sake of using AI can be a costly mistake.
Example: A manufacturing company may decide to use AI to predict machine failures. However, if machine failures are rare events, the AI model may struggle to make accurate predictions due to a lack of failure examples in the data.
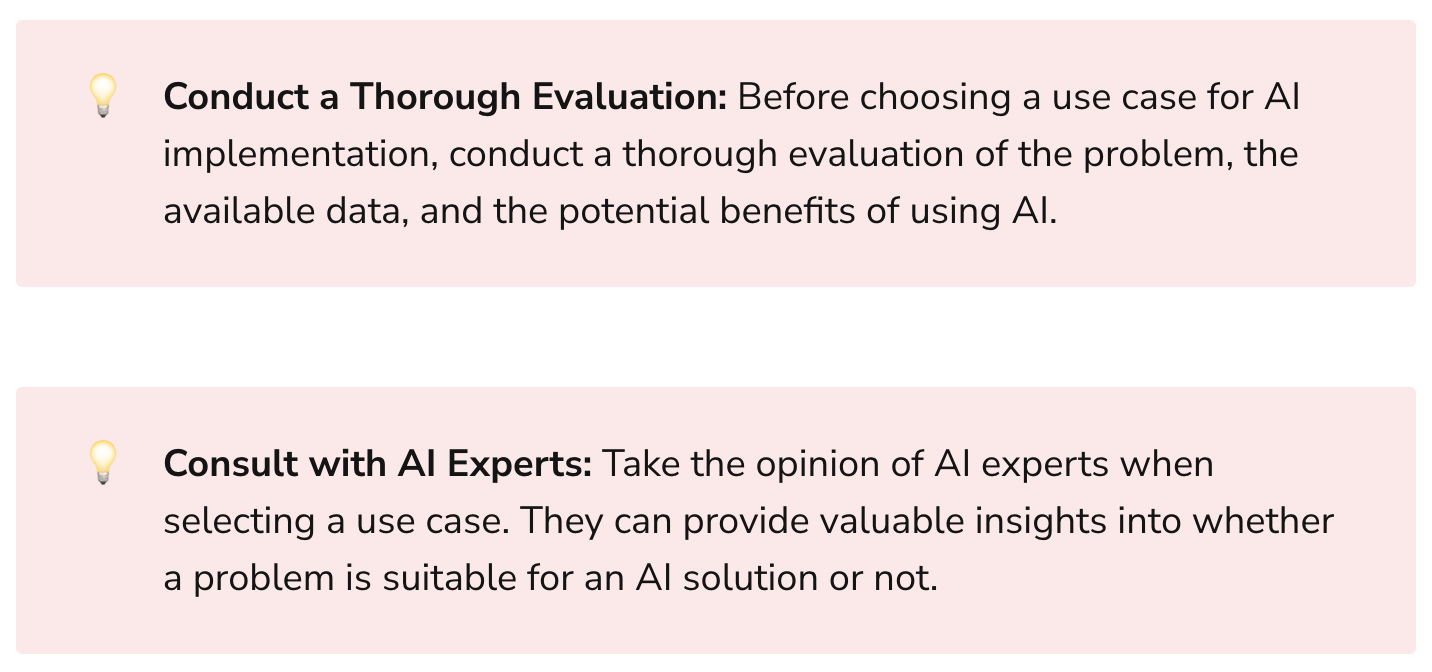
Preventive Measure: Thorough Use Case Evaluation
Ensure a thorough evaluation of potential use cases. Identify the problem you want to solve and assess whether AI is indeed the best solution. You may find that a simpler, non-AI solution can solve the problem more efficiently and cost-effectively.
Section 4: The Shortfall of Skills and Expertise
Pitfall: Lack of In-house AI Expertise
Lack of in-house AI expertise is another major reason why AI projects fail. Implementing AI is not just about running some algorithms. It requires a deep understanding of AI and its intricacies.
Example: IBM's Watson for Oncology project is a prime example of how a lack of in-house AI expertise can lead to failure. IBM, in collaboration with the University of Texas MD Anderson Cancer Center, spent $62 million developing an advanced Oncology Expert Advisor system. The aim was noble: to cure cancer patients. However, the project ultimately failed because IBM's engineers trained Watson on a relatively smaller dataset and ignored other significant features related to cancer patients. When applied in the real world, it was found that Watson recommended unsafe treatments, highlighting a mismatch between the way machines learn and the way physicians work.

Preventive Measure: Building AI Expertise and Collaborating with AI Specialists
To avoid this pitfall, organizations need to invest in building AI expertise. This can be done by upskilling existing staff, hiring AI specialists, or collaborating with external AI experts. It's important to remember that implementing AI is a multi-disciplinary task that requires expertise in fields like data science, machine learning, and domain knowledge.
Section 5: The Challenge of Ethical and Legal Considerations
Pitfall: Ignoring Ethical and Legal Considerations
AI projects can also fail due to neglect of ethical and legal considerations. Issues like data privacy, algorithmic bias, and lack of transparency can lead to legal repercussions and damage to the organization's reputation.
Example: Amazon's facial recognition software falsely recognized U.S. Congresspeople, demonstrating the risks of algorithmic bias and the potential for misuse of AI technologies.

Preventive Measure: Incorporating Ethics and Legal Compliance into AI Strategies
To address this challenge, organizations must incorporate ethics and legal compliance into their AI strategies from the very beginning. This includes conducting thorough privacy impact assessments, regularly auditing AI systems for fairness and bias, and being transparent about how AI systems make decisions.
Section 6: The Problem of Scalability
Pitfall: Failing to Scale AI Projects
Many AI projects fail to scale beyond the proof-of-concept stage. They may work well in a controlled environment but fail when applied to real-world, large-scale scenarios.
Example: The Facebook AI system has struggled to detect and prevent the upload of hate content, showing the difficulties of scaling AI solutions to handle the vast amount of content generated on social media platforms.

Preventive Measure: Planning for Scalability from the Outset
To overcome the scalability challenge, organizations must plan for scalability from the outset. This involves choosing scalable machine learning models, using scalable infrastructure, and adopting a modular approach that allows for incremental scaling of AI solutions.
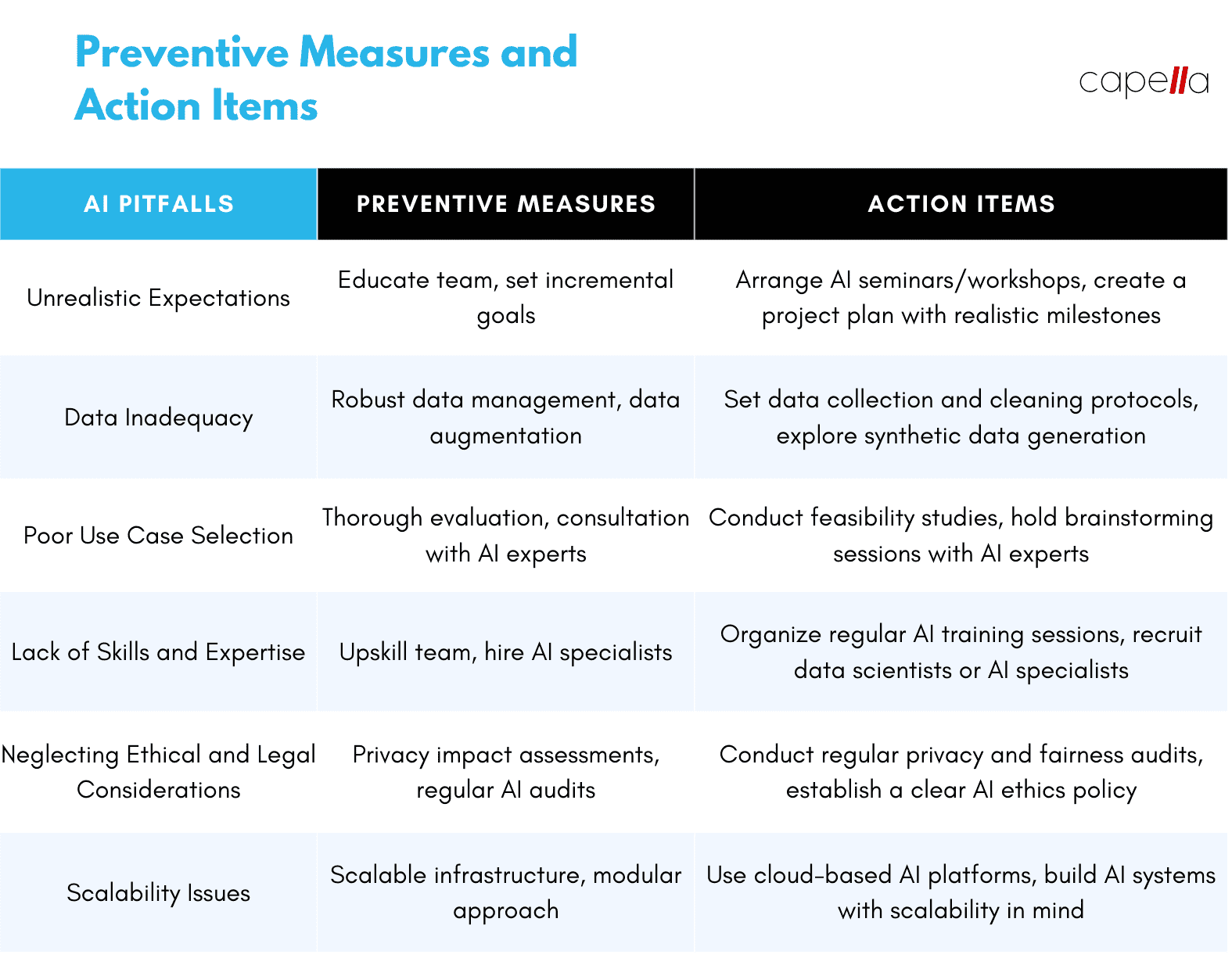
Wrapping Up
While the allure of AI is indeed enchanting, it is crucial to approach it with a sense of pragmatism and caution. AI projects can fail for a variety of reasons, from unrealistic expectations to poor use case selection, from data inadequacy to lack of expertise, and from neglect of ethical considerations to scalability issues.
But by being aware of these common pitfalls and taking preventive measures, you can increase the chances of your AI projects succeeding. Remember, the magic of AI lies not just in its technology but also in its thoughtful and responsible application.
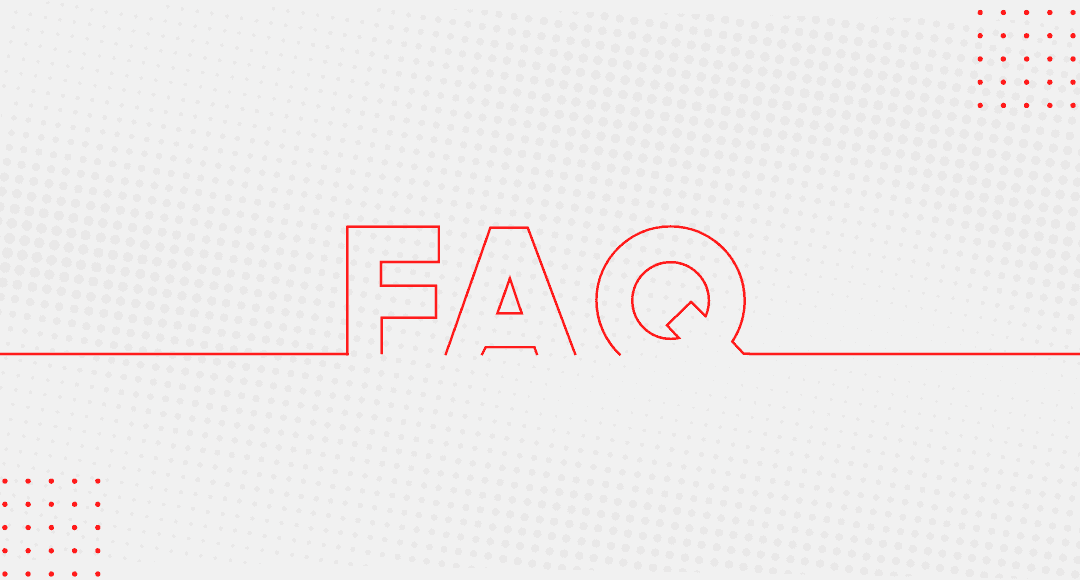
1. Why do most AI projects fail?
AI projects often fail due to a variety of reasons. These can include unrealistic expectations, poor use case selection, inadequate data, lack of expertise, negligence of ethical and legal considerations, and scalability issues. Each of these problems can significantly hamper the success of an AI project.
2. How can we prevent unrealistic expectations in AI projects?
To prevent unrealistic expectations, it's crucial to educate all team members about the capabilities and limitations of AI. AI is a powerful tool, but it's not a magic wand. It's important to set achievable, incremental goals and manage expectations from the start. Regular communication and progress updates can also help keep expectations in check.
3. How important is the quality of data in an AI project?
Data quality is paramount in AI projects. AI models learn from the data they are trained on, so poor-quality data can lead to poor results. It's crucial to have robust data management processes in place, including data cleaning, validation, and augmentation. Without high-quality, relevant data, an AI project is likely to fail.
4. What do you mean by "poor use case selection", and how can it be avoided?
Poor use case selection refers to choosing a problem or task for AI implementation that is not suitable or feasible. This can happen when the problem is too complex for current AI capabilities, or when AI is not the right tool for the job. To avoid this, it's important to conduct a thorough evaluation of potential use cases, and consult with AI experts when necessary.
5. Why is a lack of skills and expertise a common pitfall in AI projects?
AI is a complex field that requires a high level of expertise. Without the right team of experts, AI projects can fail to take off. This can be due to technical challenges, or a lack of understanding about the real-world application of AI. To prevent this, it's important to upskill the team, hire AI specialists, and foster a culture of continuous learning.
6. Why is it important to consider ethical and legal aspects in AI projects?
Ignoring ethical and legal considerations can lead to backlash and legal issues. For instance, AI models can inadvertently perpetuate bias or invade privacy, leading to ethical concerns and potential legal repercussions. Conducting privacy impact assessments, auditing AI systems regularly, and establishing clear AI ethics policies can help mitigate these risks.
7. What are scalability issues in AI projects, and how can they be avoided?
Scalability issues occur when an AI system that works well on a small scale fails when deployed at a larger scale. This can be due to computational constraints, data management issues, or a lack of robustness in the AI model. To avoid this, it's important to choose scalable models and infrastructure, and adopt a modular approach to AI development.
8. How can we ensure the success of an AI project?
Ensuring the success of an AI project involves careful planning, setting realistic expectations, choosing the right use case, managing data effectively, building a skilled team, considering ethical and legal aspects, and planning for scalability. It's also crucial to monitor the project closely, adjust plans as needed, and learn from any failures or setbacks.
9. What are some examples of AI projects that have failed due to these pitfalls?
There are several notable examples of AI projects that have failed due to these pitfalls. For instance, IBM’s Watson for Oncology project failed due to unrealistic expectations and a lack of understanding about the real-world application of AI. Similarly, Apple’s Face ID technology was easily fooled by a simple mask, indicating gaps
10. How can large enterprises benefit from AI?
Large enterprises can greatly benefit from AI. AI can automate repetitive tasks, optimize processes, provide valuable insights from data, and enhance customer experience, among other things. However, to reap these benefits, it's crucial for enterprises to avoid the common pitfalls of AI projects, such as unrealistic expectations, poor use case selection, inadequate data, lack of expertise, negligence of ethical and legal considerations, and scalability issues. Implementing preventive measures and action items, as discussed in this article, can help enterprises successfully navigate their AI journey.
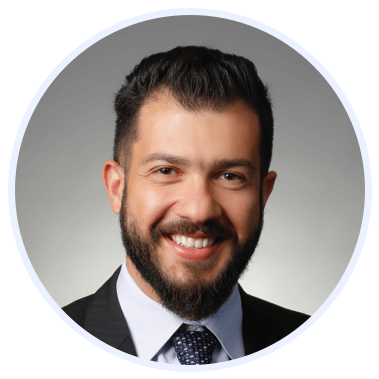
Rasheed Rabata
Is a solution and ROI-driven CTO, consultant, and system integrator with experience in deploying data integrations, Data Hubs, Master Data Management, Data Quality, and Data Warehousing solutions. He has a passion for solving complex data problems. His career experience showcases his drive to deliver software and timely solutions for business needs.