
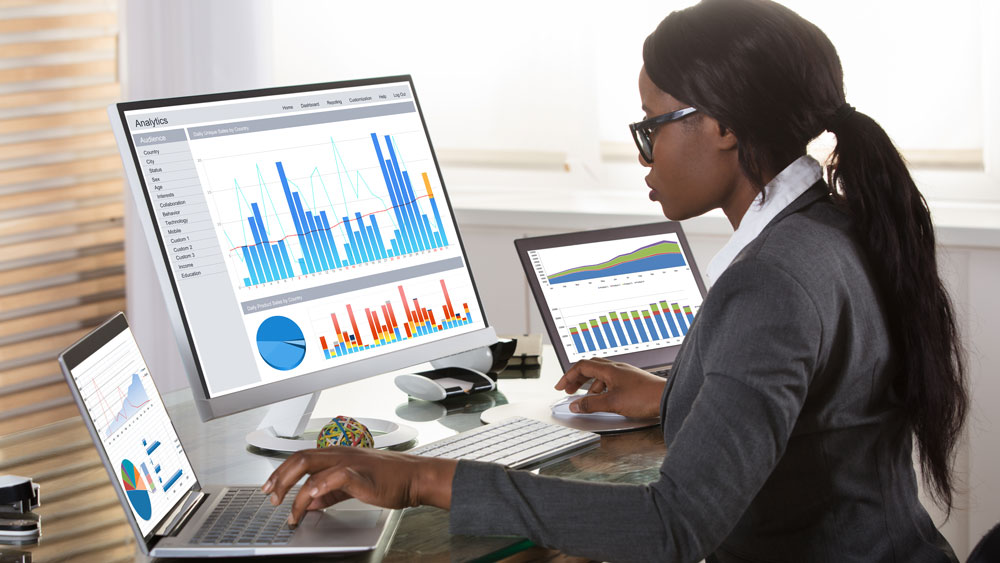
Data has become the lifeblood of modern organizations, driving strategic decision-making, operational efficiency, and customer satisfaction. However, the value of data depends heavily on its quality. Inaccurate, incomplete, or inconsistent data can lead to flawed analyses, misguided decisions, and negative business outcomes. As an IT Director or Senior Manager, it is crucial to master data quality best practices to ensure your organization harnesses the full potential of its data assets. In this article, we will explore actionable strategies and practical examples to help you achieve data quality excellence.

The Impact of Poor Data Quality
Before diving into the best practices, let's understand the far-reaching consequences of poor data quality. According to Gartner, poor data quality costs organizations an average of $15 million per year. These costs arise from various factors, such as:
Misinformed Decision-Making: Inaccurate or incomplete data can mislead decision-makers, leading to suboptimal strategies and missed opportunities. For example, consider a retail company relying on flawed customer data to identify market trends. The resulting inaccurate insights could result in misguided inventory management decisions, leading to excess stock or stockouts.
Operational Inefficiency: Inconsistent or duplicate data can hinder operational efficiency by causing errors, delays, and rework. For instance, imagine a manufacturing company with multiple systems recording product specifications. If these systems have inconsistent data, it could lead to errors during production, quality issues, and increased costs.
Poor Customer Experience: Data quality issues can directly impact customer satisfaction. For instance, imagine a customer service representative relying on outdated customer information. This could lead to frustrated customers repeating information, longer resolution times, and diminished customer loyalty.
Best Practices for Data Quality Mastery
Now that we understand the significance of data quality, let's explore the best practices that IT Directors and Senior Managers can implement to master data quality within their organizations.

1. Establish Data Quality Metrics and Goals
To improve data quality, it is crucial to define measurable metrics and set clear goals. Consider the following steps:
- Identify Key Data Quality Metrics: Determine the metrics that align with your organization's specific needs and challenges. Examples include data accuracy, completeness, consistency, and timeliness.
- Set Target Goals: Define realistic and achievable goals for each data quality metric. For instance, aim for 95% data accuracy or 100% completeness.
- Track and Monitor Progress: Implement a data quality monitoring system to regularly assess and track progress towards the defined goals. This allows you to identify areas that require improvement and measure the impact of data quality initiatives.
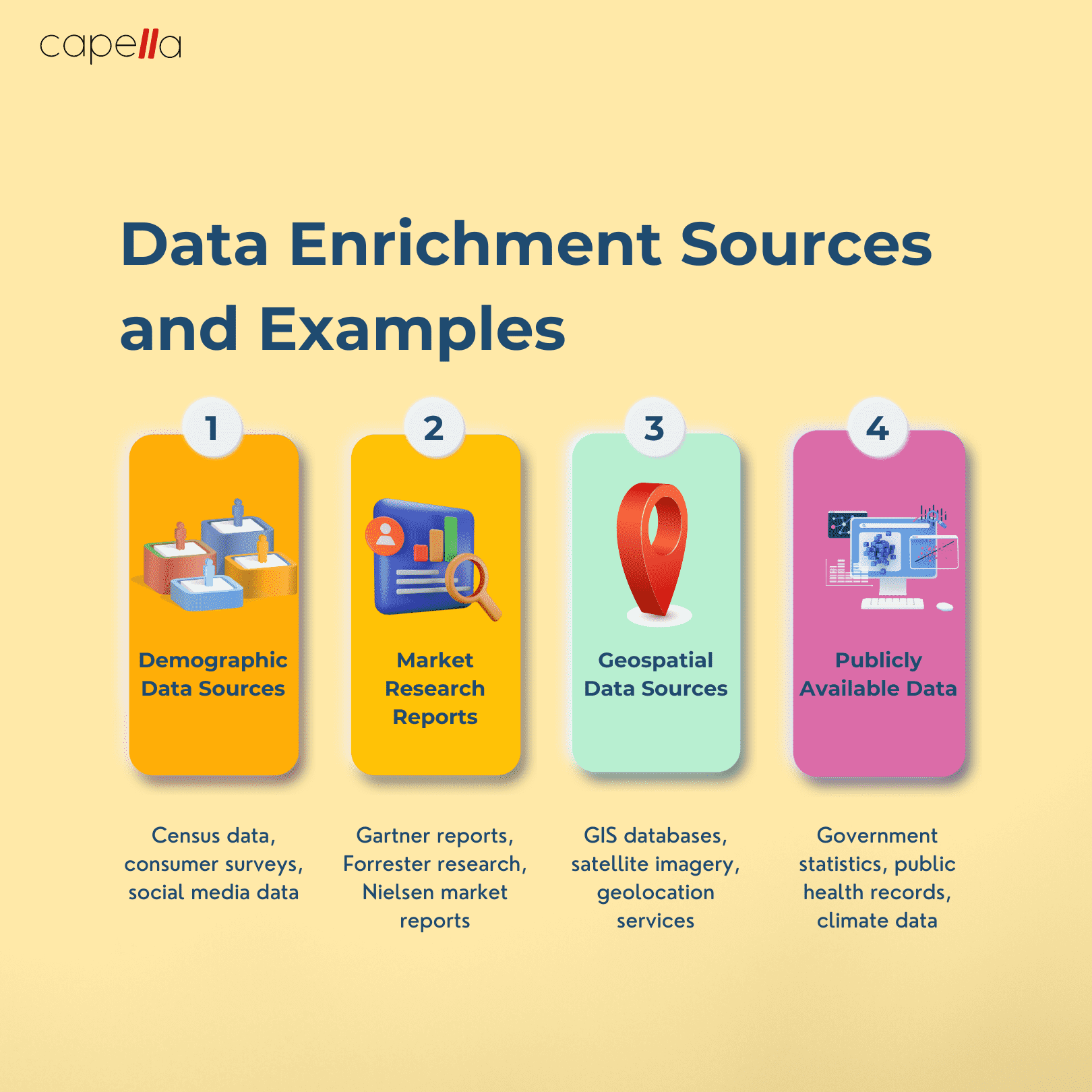
2. Implement Data Validation Processes
Data validation is a critical step in ensuring data quality. By implementing robust data validation processes, you can detect and address data issues early on. Consider the following strategies:
- Data Profiling: Perform data profiling to analyze the structure, content, and quality of your data. This helps identify anomalies, outliers, and potential data quality issues.
- Automated Validation Rules: Define and implement automated validation rules to check data integrity, accuracy, and adherence to predefined standards. For example, you can validate email addresses, phone numbers, or product codes against specific patterns or formats.
- Error Reporting and Resolution: Establish a streamlined process for error reporting and resolution. Encourage users to report data quality issues promptly, and ensure timely resolution to prevent further downstream impacts.
3. Invest in Data Integration and Governance
Data integration and governance play a vital role in maintaining data quality across various systems and processes. Consider the following practices:
- Centralized Data Repository: Establish a centralized data repository or data warehouse that consolidates data from disparate sources. This reduces data fragmentation and enhances data consistency.
- Data Standardization: Define and enforce data standards, including naming conventions, formats, and coding schemes. Standardized data ensures consistency and facilitates accurate data analysis.
- Data Access Controls: Implement data access controls and permissions to ensure that only authorized personnel can modify or manipulate data. This minimizes the risk of unauthorized changes or data corruption.

4. Ensure Data Cleansing and Enrichment
Data cleansing and enrichment processes are essential to address existing data quality issues and enhance the value of your data. Consider the following approaches:
- Duplicate Identification and Removal: Utilize data matching algorithms and techniques to identify and eliminate duplicate records. This prevents confusion, improves data accuracy, and enhances operational efficiency.
- Data Enrichment: Augment your existing data with external sources to enhance its completeness and accuracy. For instance, integrating demographic data from reputable sources can provide valuable insights for targeted marketing campaigns.
- Periodic Data Audits: Conduct regular data audits to proactively identify data quality issues and correct them. This includes verifying data accuracy, completeness, and consistency against predefined standards.
5. Foster a Data Quality Culture
Data quality is not solely an IT responsibility; it requires a collective effort across the organization. To foster a data quality culture, consider the following steps:
- Data Quality Training: Provide comprehensive training to employees on data quality concepts, processes, and best practices. This ensures that everyone understands their role in maintaining data quality.
- Data Stewardship: Designate data stewards within each department or business unit who are responsible for overseeing data quality initiatives and driving continuous improvement.
- Promote Data Accountability: Foster a culture of data accountability by clearly defining roles, responsibilities, and ownership of data within the organization. This encourages employees to take ownership of data quality and raises awareness of its importance.
Conclusion
Data quality is a critical factor in driving successful business outcomes in the digital age. By mastering data quality best practices, IT Directors and Senior Managers can ensure the reliability, accuracy, and usefulness of their organization's data assets. From establishing metrics and goals to fostering a data quality culture, every step contributes to the overall data quality excellence. Embrace these best practices, and watch as your organization unlocks the full potential of its data, propelling it towards greater success.
Remember, as the renowned data expert W. Edwards Deming once said, "In God we trust, all others must bring data."
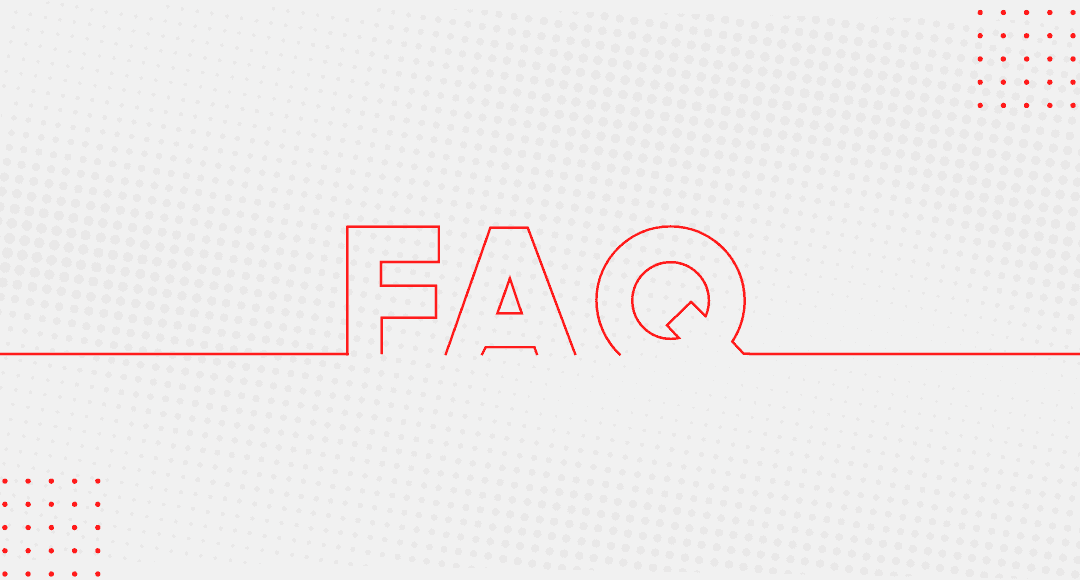
1. Why is data quality important for organizations?
Data quality is crucial for organizations because it directly impacts decision-making, operational efficiency, and customer satisfaction. Accurate, complete, and consistent data ensures informed decision-making, prevents errors and delays in operations, and enhances the overall customer experience. Poor data quality can lead to misguided strategies, operational inefficiencies, and negative business outcomes, emphasizing the need for organizations to prioritize and master data quality.
2. How can I measure data quality within my organization?
To measure data quality, you need to establish key metrics that align with your organization's goals and challenges. Common data quality metrics include data accuracy, completeness, consistency, and timeliness. Set target goals for each metric, such as aiming for 95% data accuracy or 100% data completeness. Implement a data quality monitoring system that regularly tracks and assesses progress towards these goals, enabling you to identify areas for improvement and measure the impact of data quality initiatives.
3. What are some practical steps for improving data quality?
Improving data quality requires a multi-faceted approach. Some practical steps include:
- Implementing data validation processes with automated validation rules to ensure data integrity and adherence to predefined standards.
- Conducting data profiling to identify anomalies and potential data quality issues.
- Establishing a streamlined process for error reporting and resolution to promptly address data quality issues.
- Investing in data integration and governance practices, including centralized data repositories and standardized data formats.
- Performing data cleansing and enrichment processes to address existing data quality issues and enhance the value of your data.
- Fostering a data quality culture by providing comprehensive training, designating data stewards, and promoting data accountability within the organization.
4. What are some common data validation rules that organizations can implement?
Organizations can implement various data validation rules to ensure data quality. Some common examples include:
- Email Format Validation: Verifying if email addresses conform to a valid format, preventing the inclusion of incorrect or malformed email addresses.
- Phone Number Format Validation: Ensuring that phone numbers adhere to a specific format, such as including the country code and a specific number of digits.
- Product Code Verification: Validating product codes against a predefined set of accepted codes to ensure consistency and accuracy.
- Date Range Validation: Checking if dates fall within a specific range or follow a defined date format, preventing the inclusion of invalid or out-of-range dates.
5. How can data integration and governance practices help improve data quality?
Data integration and governance practices play a vital role in maintaining data quality. By establishing a centralized data repository or data warehouse, organizations can consolidate data from various sources, reducing data fragmentation and enhancing data consistency. Data integration also enables streamlined data access and eliminates data silos.
Data governance ensures that data is managed effectively and adheres to predefined standards. This includes defining and enforcing data standards, naming conventions, and coding schemes. By implementing data access controls and permissions, organizations can ensure that only authorized personnel can modify or manipulate data, reducing the risk of unauthorized changes or data corruption.
6. How can data cleansing and enrichment processes enhance data quality?
Data cleansing and enrichment processes are essential for addressing existing data quality issues and enhancing the value of data. Data cleansing involves identifying and correcting data errors, such as removing duplicates, resolving inconsistencies, and standardizing formats. This ensures data accuracy and reliability.
Data enrichment involves enhancing existing data with external sources to improve its completeness and accuracy. For example, integrating demographic data from reputable sources can provide valuable insights for targeted marketing campaigns. By augmenting data with relevant external sources, organizations can enhance decision-making, improve customer segmentation, and gain a competitive edge.
7. How can organizations foster a data quality culture?
Fostering a data quality culture requires active engagement from all levels of the organization. Here are some key steps to promote a data quality culture:
- Provide comprehensive data quality training to employees across departments, educating them on data quality concepts, processes, and best practices.
- Designate data stewards within each department or business unit who are responsible for overseeing data quality initiatives, driving continuous improvement, and collaborating with other teams.
- Clearly define roles, responsibilities, and ownership of data within the organization to promote data accountability.
- Encourage employees to take ownership of data quality by emphasizing its importance and the impact it has on business outcomes.
- Recognize and reward individuals or teams that demonstrate outstanding commitment to data quality.
8. How can data audits help improve data quality?
Data audits are essential for proactively identifying and addressing data quality issues. By conducting regular data audits, organizations can:
- Verify data accuracy, completeness, and consistency against predefined standards.
- Identify data anomalies, outliers, and potential data quality issues.
- Take corrective actions to resolve identified issues, such as cleansing data, removing duplicates, or standardizing formats.
- Monitor the effectiveness of data quality initiatives and measure progress over time.
Data audits provide insights into the overall health of data quality and allow organizations to continuously improve their data management processes.
9. What are the roles and responsibilities related to data quality management?
Data quality management involves several roles and responsibilities within an organization. Here are some key roles and their responsibilities:
- Data Steward: Oversees data quality initiatives, defines and enforces data standards, and ensures data integrity and consistency.
- IT Director: Establishes data quality metrics and goals, implements data validation processes, and ensures the availability of necessary resources for data quality initiatives.
- Senior Manager: Fosters a data quality culture, promotes data accountability, and allocates resources for data quality improvement.
- Data Analyst: Conducts data profiling and analysis, implements data cleansing and enrichment processes, and collaborates with stakeholders to address data quality issues.
It is important to clearly define these roles and ensure effective collaboration among them to achieve data quality excellence.
10. How can organizations justify investments in data quality initiatives?
Investing in data quality initiatives yields significant benefits for organizations. Justifying these investments can be done through several key points:
- Improved Decision-Making: Accurate and reliable data leads to informed decision-making, resulting in better strategic choices and increased business success.
- Operational Efficiency: Enhanced data quality reduces errors, delays, and rework, resulting in streamlined operations and cost savings.
- Customer Satisfaction: High-quality data enables personalized and efficient customer experiences, leading to increased customer satisfaction and loyalty.
- Regulatory Compliance: Good data quality ensures compliance with regulations, mitigating legal and financial risks.
- Competitive Advantage: Organizations with superior data quality gain a competitive edge by making data-driven decisions and providing better customer experiences.
By emphasizing the potential return on investment and the value of data quality, organizations can justify the allocation of resources towards data quality initiatives.
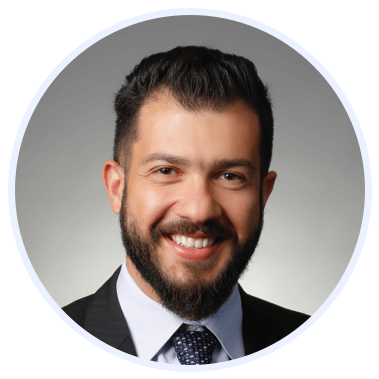
Rasheed Rabata
Is a solution and ROI-driven CTO, consultant, and system integrator with experience in deploying data integrations, Data Hubs, Master Data Management, Data Quality, and Data Warehousing solutions. He has a passion for solving complex data problems. His career experience showcases his drive to deliver software and timely solutions for business needs.